By: Praneetha Neelapareddigari, Department of Computer Science & Engineering, Madanapalle Institute of Technology and Science, Angallu (517325), Andhra Pradesh. praneetha867reddy@gmail.com
Abstract
AI and Zero Trust Security are gaining popularity exponentially to be included in the modern approach to cybersecurity. The threats originating from cyberspace have become more diverse and frequent, therefore the conventional security paradigm based on the perimeter security mechanisms is insufficient. This is ignited by the fact that communications within internal networks are perceived to be safe and this gives room for a smart attacker to penetrate. But getting this information and comprehending the patterns of events to identify anomalies in time presents the following challenge to LSPs: AI helps to solve it. Thus, the integration of AI with the Zero Trust principles, under which no entity is trustworthy whether it is situated within or outside the network, will help organizations improve their security situation. This integration makes it possible for constant supervision and changing of the security policies real time, which minimizes on the threats posed for a stronger cybersecurity. The abstract describes how AI improves Zero Trust Security and examines the inefficiency of the previous security frameworks and the effectiveness of new threats.
Keywords: Artificial Intelligence, Zero Trust Security, Machine Learning
Introduction
In the contemporary context of organizations high vulnerability to effective cyber threats, the traditional approaches to security are no longer sufficient. The concept that has now risen to becoming a stable security model that can effectively tackle these problems is known as the Zero Trust security model as it shifts the way authorization of resources is conducted. This is diametrically opposed to traditional approaches of encoding security on the external borders as the Zero Trust model posits the concept of ‘never trust, always check,’ which means that even if a request comes within the organization’s perimeters, it will always be subject to strict evaluation[1].
It is, therefore, evident that AI is central to the provision of better Zero Trust security measures. The use of AI can help to monitor the network activity, find the suspicious signs, and predict the potential threats utilizing such theories as machine learning and big data[2]. Such integration makes possible the use of security measures that can be adjusted in real time to ameliorate threats and prevent the attacks that might have gone unnoticed due to normal, traditional patterns or approaches.
That is why, interaction between AI and security becomes better, as the number of companies, which use the Zero Trust model, increases. Conversation with an AI can be done through the application of tools that will help the security professionals maximize the level of security even down to the details and this would be very helpful most especially when it comes to applying the concept of Zero Trust security model. Not only does the new partnership increase protection but it also enables one to respond faster to threats, which speaking of Zero Trust, is an advantage[3]. The use of the like technologies together can be regarded as a giant step towards the goal of safeguarding data as well as integrity in today’s globalization.
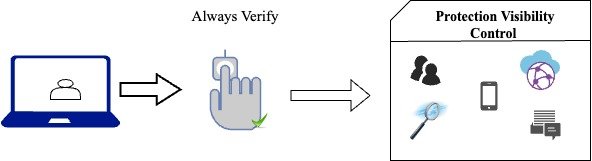
1. Basic Concepts of Artificial Intelligence
Artificial Intelligence or commonly known as AI is a cluster of technologies and techniques and the overall objective of which is to exhibit intelligent behaviour in machines[4]. Specific components of AI are ML in which data is provided to algorithms to learn and make decisions further and DL is the subcategory of ML where complex networks containing many layers are used to analyse data. In cybersecurity, AI is applied for threat detection, response automation and to predict risks from big data for deviation. Application of AI in cybersecurity has an added advantage in that it gives a better accuracy in detection, it operates efficiently and is capable of handling large data[5]. But at the same time, there are some disadvantages, which relate to the possible false signals, large amount of data and computational resources, and reasonable updating of AI systems in the framework of appearance of new threats.
2. Integration of AI with Zero Trust Security
In addition, the use of Artificial intelligence enhances the characteristics of the Zero Trust security model by expanding its capacity in Real-time validate and risk assessment. Largely relying on strict access controls and identities, Traditional Zero Trust Strategies are mostly blunt, and AI has amplified it in this regard in the sense that it enables the decision-making mechanism to be contextually informed. In terms of the risk assessment, machine learning algorithms when are trained on big data may identify the risk in conjunction with the requester for making a further decision on granting the access rights to specific user and devices only[6].
The first objective concerning the setting of the AI-based solutions for the authentication and authorization can be explained as the transition to a new level in applying the methods of protection for digital assets. In more secure and context related activity there is a possibility to have biometric recognition, behavioural analysis, and adaptive risk scoring with the help of AI. For instance, investigate the user’s steps and the circumstances of the used devices and realize that they are amazing and, ergo, fraudulent from a certain perspective. This enhanced authentication capability enables the possibility to permit or deny access to grant resources because of risk assessment and this, in essence, is usually checking each request for access, thus a Zero Trust model.
AI implementation in the real-time and the anomaly detection improves the security within the Zero Trust model’s method of approach. Algorithms involved in an AI system can be actively and continuously scanning through network traffic and users’ activity to identify signs or patterns of a possible penetration into a network. When it comes to such intelligent technologies as machine learning, these systems can demonstrate very high specificity in terms of what is normal and what is malicious, including the generation of early warnings when the latter is identified. Similarly, the identification of threats in real-time allows organizations to respond to security occurrences at the earliest time possible, which is part of the Zero Trust paradigm’s strict security measures.
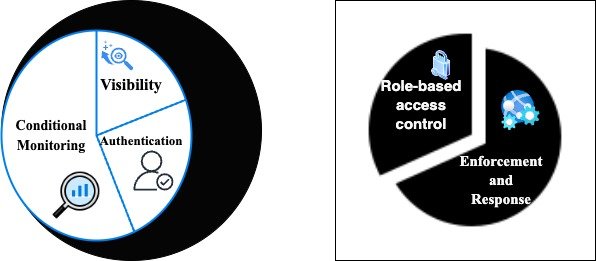
3. Real-World Applications
That is why many large organizations that managed to integrate AI within the Zero Trust model underline the increase in security levels and the optimization of production processes. For instance, Microsoft uses AI in identifying and combating threats by incorporating threat intelligence frameworks in its AI platform while Google has adopted machine learning in identifying threats within organisations faster than the processes taken by many organisations manually. Such implementations have proved to provide better solutions in identifying unusual cases as well as minimizing the cases of wrong alerts. Some of the insights are that training of AI models must be a constant process and that it needs to fit right into the organization’s security architecture[7]. The study shows that the organizations that incorporate AI into the Zero Trust model tend to operate in a stronger and more effective security system than those that have applied traditional approaches and could hardly cope with new threats.
4. Challenges
There are a few challenges of implementing AI alongside the Zero Trust, including the following such as risks that are associated with the AI and automated systems that can overlook high-risk threats while emphasizing on low-risk threats and give out false alarms. There are also issues of data protection and ethical concerns especially on data sensitivity where there is need to enhance adherence to privacy and use of Artificial Intelligence and its practice[8]. Furthermore, AI models need to be updated frequently because the threat environment is constantly shifting, as is the organizational context within which AI is used. AI systems must be updated often and integrated into the current best practices of maintaining the Zero Trust architecture.
5. Future Trends
Next-gen machine learning algorithms, quantum computer and more secure biometric systems are emerging technologies which will change the face of AI as well as Zero Trust security. Because of this future scrutiny, the predictions are that upcoming AI-based Zero Trust models will be far more dynamic and constantly adapt themselves to battle threats in near real-time using predictive analytics. In a landscape where cyber threats are constantly evolving, AI will clearly be an essential element for adaptive security controls and enhanced threat intelligence to generate responses with greater accuracy[8]. This could conceivably result in stronger, more secure and intelligent security architectures that are even better positioned to handle the constantly changing and increasingly sophisticated cyber threat environment
6. Recommendations
There are a number of best practices for how to successfully incorporate AI in Zero Trust architecture: The first thing is that they need to be well-integrated within your established security systems and secondly, you must have good view into as only then can you enable controls over the outputs from these decisions. Guidance on ethical usage of AI covering issues like data privacy and explainability in algorithmic decision-making is important to ensure trust as well as legal compliance. Assessment of AI Performance: Continuous monitoring both on its outputs, along continuous feedback loops and updates to models keeps the system effective over time (in response emerging threats)and in line with organizational needs. Further, improved cross-functional alignment with security and AI experts will improve the dynamic efficacy of Zero Trust in real-world applications.
Conclusion
To sum up, merging AI in Zero Trust structure along with all of its benefits advances the capability to identify complex threats adaptive security measures as well as improvement operational efficiency. The most important of those are that the solution must solve for key challenges such as data privacy and continued training on AI models, and need to also adhere to ethical practices. To keep a step ahead of threats and ensure security remains robust, development in both AI and Zero Trust is fundament.
References
- H. Kang, G. Liu, Q. Wang, L. Meng, and J. Liu, “Theory and Application of Zero Trust Security: A Brief Survey,” Entropy, vol. 25, no. 12, Art. no. 12, Dec. 2023, doi: 10.3390/e25121595.
- D. Ajish, “The significance of artificial intelligence in zero trust technologies: a comprehensive review,” J. Electr. Syst. Inf. Technol., vol. 11, no. 1, p. 30, Aug. 2024, doi: 10.1186/s43067-024-00155-z.
- E. S. Hosney, I. T. A. Halim, and A. H. Yousef, “An Artificial Intelligence Approach for Deploying Zero Trust Architecture (ZTA),” in 2022 5th International Conference on Computing and Informatics (ICCI), Mar. 2022, pp. 343–350. doi: 10.1109/ICCI54321.2022.9756117.
- C. Zhang and Y. Lu, “Study on artificial intelligence: The state of the art and future prospects,” J. Ind. Inf. Integr., vol. 23, p. 100224, Sep. 2021, doi: 10.1016/j.jii.2021.100224.
- P. Pappachan, Sreerakuvandana, and M. Rahaman, “Conceptualising the Role of Intellectual Property and Ethical Behaviour in Artificial Intelligence,” in Handbook of Research on AI and ML for Intelligent Machines and Systems, IGI Global, 2024, pp. 1–26. doi: 10.4018/978-1-6684-9999-3.ch001.
- B. Hale, D. L. Van Bossuyt, N. Papakonstantinou, and B. O’Halloran, “A Zero-Trust Methodology for Security of Complex Systems With Machine Learning Components,” doi: 10.1115/DETC2021-70442.
- “An Enhanced Multi-Factor Authentication and Key Agreement Protocol in Industrial Internet of Things,” Insights2Techinfo. Accessed: Aug. 14, 2024. [Online]. Available: https://insights2techinfo.com/an-enhanced-multi-factor-authentication-and-key-agreement-protocol-in-industrial-internet-of-things/
- Y. He, D. Huang, L. Chen, Y. Ni, and X. Ma, “A Survey on Zero Trust Architecture: Challenges and Future Trends,” Wirel. Commun. Mob. Comput., vol. 2022, no. 1, p. 6476274, 2022, doi: 10.1155/2022/6476274.
- Gupta, B. B., Gaurav, A., & Panigrahi, P. K. (2023). Analysis of retail sector research evolution and trends during COVID-19. Technological Forecasting and Social Change, 194, 122671.
- Aldweesh, A., Alauthman, M., Al Khaldy, M., Ishtaiwi, A., Al-Qerem, A., Almoman, A., & Gupta, B. B. (2023). The meta-fusion: A cloud-integrated study on blockchain technology enabling secure and efficient virtual worlds. International Journal of Cloud Applications and Computing (IJCAC), 13(1), 1-24.
Cite As
Neelapareddigari P. (2024) AI and Zero Trust Security, Insights2Techinfo, pp.1