By: Poojitha Nagishetti, Department of Computer Science & Engineering (Data Science), Student of Computer Science & Engineering, Madanapalle Institute of Technology and Science, Angallu(517325), Andhra Pradesh.
Abstract –
Artificial Intelligence (AI) is hugely enhancing the prediction and management of chronic disease in healthcare. Chronic diseases such as Alzheimer’s, cardiovascular conditions, diabetes, and cancer pose significant global health challenges. For enabling early detection and personalized treatment plans AI models process huge volume of datasets to identify patterns and risk factors. This article shows the various uses of AI in predicting these diseases, individualized care, underscoring its benefits like increased accuracy and proactive disease management. It also discusses about the challenges and future directions of AI in this field, along with the need of robust data security, model interpretability, ethical considerations, and integration with clinical workflows. As AI technology continues to explore, it holds the promise of significant improvement of patient outcomes and reducing the of burden on individuals of chronic diseases and healthcare systems worldwide.
Keywords – Artificial Intelligence, Chronic Disease Prediction, Personalized Medicine, Ethical AI, Wearable Technology.
Introduction –
Artificial Intelligence (AI) is transforming the healthcare sector, particularly in predicting and management of chronic diseases[1]. Chronic diseases such as cardiovascular diseases, diabetes, Alzheimer’s, and cancers will significantly contribute to the global morbidity and mortality, that requires long-term management and imposing heavy burdens on individuals and healthcare systems.
Chronic diseases are persistent and progressive, if not managed properly it often led to severe complications[2]. These diseases will heavily depend on manual analysis and clinical expertise if we use traditional methods of diagnosis. However, the volume and the growing complexity of healthcare data make it difficult to identify the patterns and risk factors signaling in early disease onset.
AI-powered models will provide a solution to analyze vast amount of data, uncovered hidden patterns and correlations that may be missed by human analysis by using advanced algorithms[3]. These models will examine diverse data sources, including medical records, genetic information, imaging data, and patient-reported outcomes, to predict the likelihood of developing chronic diseases. This high-precision data processing and analysis offer significant advantages over traditional methods.
AI is potential of beyond prediction to personalized medicine in healthcare, where treatment plans are tailored to individual patient profiles, and proactive care, where interventions are made before severe symptoms appear[4]. These capabilities not only used for improving patient outcomes but also reduce the healthcare costs by minimizing the need for extensive treatment and hospitalizations.
Despite its promise, integrating AI into chronic disease prediction comes with challenge[5]. Issues like data privacy, model interpretability, and integration with existing clinical workflow need to be addressed to fully leverage AI’s potential. Along with those ethical considerations must be carefully managed to ensure equitable and fair treatment for all patients.
This article describes the current applications of AI in predicting chronic diseases, that highlights the benefits, and addressing the challenges[6]. By exploring AI’s potential and limitations, we aim to provide a comprehensive overview of how this technology can transform chronic disease management and improve healthcare outcomes worldwide.
The importance of predicting chronic diseases –
Predicting chronic diseases is very crucial in modern healthcare for several key reasons. Chronic diseases like cardiovascular diseases, diabetes, Alzheimer’s, and cancer are major contributors to global illness and mortality rates[7]. Early detection and intervention can significantly lower healthcare costs, improve patient outcomes, and enhance overall public health.
1. Improving patient outcomes:
Timely intervention is essential for improving patient outcomes which make possible by early prediction. Early detection of diseases helps a patient to begin the treatment before the condition progresses. For example, recognizing early signs of cardiovascular disease can lead to preventive measures that stops heart attacks or strokes. Similarly, early detection of diabetes allows for lifetime modification and treatments that can prevent or delay complications like neuropathy, cardiovascular diseases, and retinopathy.
2. Developing personalized treatment plans:
AI-powered models for prediction can analyze extensive data to provide personalized risk assessments. These models will consider individual patient profiles, including lifestyle factors, medical history, and genetic information, to create tailored treatment plans. Personalized medicine ensures patient receive the most effective interventions, reducing the trial-and-error approach often associated with standard treatments. This leads to better treatment adherence and disease management.
3. Reducing healthcare costs:
Compared to the other diseases chronic diseases are most costly to treat due to their long-term nature and the need for ongoing care. Early prediction and prevention of chronic diseases can substantially reduce the healthcare expenses by decreasing the need for expensive treatments, hospitalizations, and emergency interventions. For example, managing diabetes early can prevent expensive complications like kidney failure or amputations. Similarly, early detection of cancer will often result in less costly treatment and less invasive.
4. Enhancing public health strategies:
At the population level predicting the chronic diseases can inform public health strategies and resource allocations. Identifying at-risk population can allows the healthcare providers and policy makers to implement targeted prevention programs and screening. This proactive approach can lessen their impact on society and reduce the prevalence of chronic diseases. For example, community-wide hypertension screening programs can lead to preventive measures that improve overall community health and identify individuals at risk of cardiovascular diseases.
5. Fostering proactive healthcare:
For effectively managing chronic diseases shifting from reactive to proactive is essential. Predictive models will enable the healthcare providers to move beyond merely treating symptoms to prevent disease onset. This shift supports a more sustainable healthcare systems focused on maintaining health rather than solely treating illness. By preventing disease related complications and maintain better overall health the proactive healthcare can enhance patient’s quality of life. Figure 1 shows the overview of AI-powered models for predicting chronic diseases, benefits, future directions, and ethical considerations.
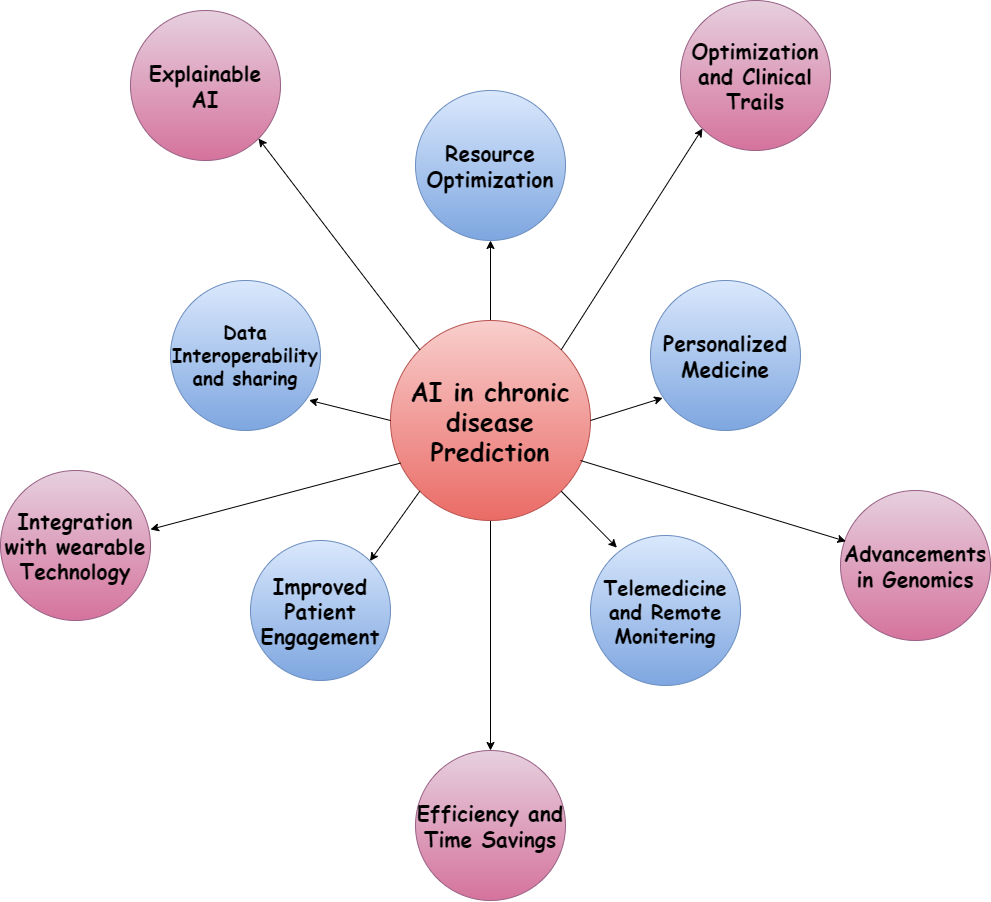
Benefits of AI in chronic disease prediction –
By offering numerous benefits in prediction and management of chronic diseases the artificial intelligence (AI) is transforming the landscape of healthcare[8]. The integration of AI into healthcare systems brings about significant improvements in efficiency, accuracy, and patient outcomes[9]. Here are some of the key benefits of using AI in chronic diseases.
1. Enhanced Accuracy:
AI models can analyze the huge volume of data with high precision, leading to more accurate prediction than the traditional methods. These models can identify the complex patterns and correlations in medical records, imaging results, genetic data, and patient-reported information that might be overlooked by human analysts. This heightened accuracy helps in the early detection of diseases, which is crucial for effective treatment and management.
2. Personalized medicine:
By considering the unique characteristics of each patient AI enables the development of personalized treatment plans. By analyzing individual lifestyle factors, genetic profiles, and medical histories, AI can recommend tailored interventions that are more likely to be effective. Personalized medicine improves treatment outcomes and reduces the risk of adverse reactions, as therapies are better suited to individual needs.
3. Continuous learning and Improvement:
AI models have the capability to learn and improve over time by continuously analyzing new data. This iterative learning process allows AI models to refine their predictions and adapt to changing healthcare environments. AI systems become increasingly accurate and reliable, enhancing their effectiveness in chronic diseases prediction and management, as more data becomes available.
Future Directions –
The future of AI in chronic disease prediction is highly promising, with advanced technology like using genomics for personalized treatment plans and integrating wearable technology for ongoing health monitoring. Better data interoperability will enable AI to provide more accurate and comprehensive predictions[10]. Developing Explainable AI will enhance trust and transparency, while robust ethical frameworks will ensure responsible use. Greater collaboration with healthcare professionals and AI-driven population health management will improve diagnostic accuracy and treatment planning. Additionally, AI will support telemedicine, remote monitoring for timely intervention, and optimize clinical trials[11]. Continuous learning will keep AI models effective, a more sustainable healthcare systems, and leading to better health outcomes. Table 1 explains the summary of AI in healthcare for chronic disease management.
Table 1: Summary of AI in Healthcare for Chronic Disease Management.
Aspect | Details |
Chronic Diseases | Cardiovascular Diseases, Diabetes, Alzheimer’s, Cancer |
AI Applications | Pattern Recognition, Risk Factor Identification, Early Detection, Personalized Treatment Plans |
Benefits of AI | Increased Accuracy, Individualized Care, Proactive Disease Management |
Key Challenges | Data Security, Integration with Clinical Workflows, Model Interpretability, Ethical Considerations |
Future Directions | Enhanced Data Security Measures, Better Integration with Healthcare Systems, Improved Model Transparency, Addressing Ethical Issues |
Impact on Healthcare | Improved Patient Outcomes, Reduced Burden on Healthcare Systems |
Ethical Consideration –
Ethical considerations are paramount in AI for chronic disease prediction. Ensuring security and data privacy is crucial to predict patient information. Addressing biases is also essential in AI models to prevent disparities in healthcare outcomes. Transparency in AI decision-making process is needed to build trust among patient and healthcare providers. Developing robust regulatory frameworks will ensure that AI applications adhere to ethical standards and are used responsibly. Additionally, obtaining informed consent from patients for ensuring equitable access and the use of their data to AI-driven healthcare solutions are critical to maintaining fairness and integrity in the healthcare.
Conclusion –
By concluding that AI models for predicting chronic diseases offer great potential to transform healthcare, providing increased accuracy, personalized treatment plans, and proactive care, which improve patient outcomes and reduce costs. Future developments, such as genomics, telemedicine, and integrating wearable devices, will further enhance AI’s capabilities. Addressing ethical concerns like bias, data privacy, and transparency is vital for the responsible use of AI in healthcare. By tacking these challenges, AI can play a crucial role in creating a more efficient, equitable, and sustainable healthcare system.
References –
- N. Noorbakhsh-Sabet, R. Zand, Y. Zhang, and V. Abedi, “Artificial Intelligence Transforms the Future of Health Care,” Am. J. Med., vol. 132, no. 7, pp. 795–801, Jul. 2019, doi: 10.1016/j.amjmed.2019.01.017.
- M. Moslehpour, K. Y. Chau, Y.-T. Tu, K.-L. Nguyen, M. Barry, and K. D. Reddy, “Impact of corporate sustainable practices, government initiative, technology usage, and organizational culture on automobile industry sustainable performance,” Environ. Sci. Pollut. Res. Int., vol. 29, no. 55, pp. 83907–83920, Nov. 2022, doi: 10.1007/s11356-022-21591-2.
- A. Zewail and S. Saber, “AI-Powered Analytics in Healthcare: Enhancing Decision-Making and Efficiency,” Int. J. Appl. Health Care Anal., vol. 8, no. 5, Art. no. 5, May 2023.
- F. C. Udegbe, O. R. Ebulue, C. C. Ebulue, and C. S. Ekesiobi, “AI’S IMPACT ON PERSONALIZED MEDICINE: TAILORING TREATMENTS FOR IMPROVED HEALTH OUTCOMES,” Eng. Sci. Technol. J., vol. 5, no. 4, Art. no. 4, Apr. 2024, doi: 10.51594/estj.v5i4.1040.
- U. E. Bauer, P. A. Briss, R. A. Goodman, and B. A. Bowman, “Prevention of chronic disease in the 21st century: elimination of the leading preventable causes of premature death and disability in the USA,” The Lancet, vol. 384, no. 9937, pp. 45–52, Jul. 2014, doi: 10.1016/S0140-6736(14)60648-6.
- I. Setiawan et al., “Utilizing Random Forest Algorithm for Sentiment Prediction Based on Twitter Data,” 2022, pp. 446–456. doi: 10.2991/978-94-6463-084-8_37.
- E. Badidi, “Edge AI for Early Detection of Chronic Diseases and the Spread of Infectious Diseases: Opportunities, Challenges, and Future Directions,” Future Internet, vol. 15, no. 11, Art. no. 11, Nov. 2023, doi: 10.3390/fi15110370.
- M. Subramanian et al., “Precision medicine in the era of artificial intelligence: implications in chronic disease management,” J. Transl. Med., vol. 18, no. 1, p. 472, Dec. 2020, doi: 10.1186/s12967-020-02658-5.
- G. Battineni, G. G. Sagaro, N. Chinatalapudi, and F. Amenta, “Applications of Machine Learning Predictive Models in the Chronic Disease Diagnosis,” J. Pers. Med., vol. 10, no. 2, Art. no. 2, Jun. 2020, doi: 10.3390/jpm10020021.
- T. Haksoro, A. S. Aisjah, Sreerakuvandana, M. Rahaman, and T. R. Biyanto, “Enhancing Techno Economic Efficiency of FTC Distillation Using Cloud-Based Stochastic Algorithm,” Int. J. Cloud Appl. Comput. IJCAC, vol. 13, no. 1, pp. 1–16, Jan. 2023, doi: 10.4018/IJCAC.332408.
- L. Triyono, R. Gernowo, P. Prayitno, M. Rahaman, and T. R. Yudantoro, “Fake News Detection in Indonesian Popular News Portal Using Machine Learning For Visual Impairment,” JOIV Int. J. Inform. Vis., vol. 7, no. 3, pp. 726–732, Sep. 2023, doi: 10.30630/joiv.7.3.1243.
- Sarin, S., Singh, S. K., Kumar, S., Goyal, S., Gupta, B. B., Arya, V., & Chui, K. T. (2024). SEIR‐driven semantic integration framework: Internet of Things‐enhanced epidemiological surveillance in COVID‐19 outbreaks using recurrent neural networks. IET Cyber‐Physical Systems: Theory & Applications.
- Chhabra, A., Singh, S. K., Sharma, A., Kumar, S., Gupta, B. B., Arya, V., & Chui, K. T. (2024). Sustainable and intelligent time-series models for epidemic disease forecasting and analysis. Sustainable Technology and Entrepreneurship, 3(2), 100064.
Cite As
Nagishetti N. (2024) AI-Powered Models for Predicting Chronic Diseases, Insights2Techinfo, pp.1