By: Ankita Manohar Walawalkar, Department of Business Administration, Asia University, Taiwan, ankitamw@ieee.com
Abstract:
Wide range of multidisciplinary connections among cyber security as well as artificial intelligence (AI). Primarily, to develop keen models for applying malware arrangement and interruption uncovering and menacing intellect sensing, deep learning, can be presented in cyber security. Additionally, AI will experience numerous cyber intimidations, which will interrupt their model, learning, and choices. Therefore, samples of AI require exact cyber security and fortification machineries to battle confrontational machine learning, preserve confidentiality in machine learning, safeguard united education.
Introduction:
New networking and computation tools like big data, Software Defined networking (SDN), and fog computing are pouring speedy cyberspace development. At the same time, the mounting significance of cybersecurity has made it a supreme concern in the developing cyberspace landscape. Classic security, trusting on inert controller of strategies similar to firewalls and intrusion detection systems (IDSs), is insufficient against modern intimidations like Advanced Persistent Threats (APTs) and zero-day outbreaks [1]. To capitalize on the safety level of essential system properties, it is crucial to grow pioneering safety defence procedures that can handle with expanded and renewable terror. AI has made wider progress and has allowed individuals to advantage from additional data, get improved outcomes [2].
Artificial intelligence a novel leaning of cyber security:
Initial AI implementation complex knowledge bases, but manual processes made it unreasonable. Machine Learning (ML) emerged to extract models from raw data, applying algorithms alike Bayesian, linear regression, and decision trees. Whereas powerful, ML has limitations in accuracy on complex data. Deep Learning (DL) discourses these shortages by pretending human neuron procedures, producing complex neural architectures [3]. DL is a protuberant research focus, extensively useful across diverse industrial situations. This outline explores classification and applications of cutting-edge DL replicas in various research areas [1].
A. Deep Learning Classification
The learning mechanism is founded on its classification of DL. Mainly three types of main learning
Instruments includes unsupervised learning, reinforcement learning and supervised learning [2].
i. Supervised learning (SL)
SL undoubtedly needs labelled input information, and is typically utilise as a regression mechanism or a sorting instrument. For instance, malware uncovering is a characteristic second organization scenario.
In dissimilarity to sorting, reversion knowledge outputs a forecast cost that is one or extra continuous-valued figures rendering to the involved information [4].
ii. Unsupervised learning (UL)
In divergence to SL, the contributed data of unverified learning is unlabelled. UL is frequently utilised to bunch data, decrease Complexity or evaluation density. Compounding a fuzzy Deep Belief Network (DBN) system with the Takagi-Sugeno-Kang (TSK) fuzzy system allows adaptive control of the DBN depth, easing extremely precise clump. [5].
iii. Reinforcement learning (RL)
RL is mainly on satisfying actions taken by a concentrating agent, combination of aspects of supervised learning (SL) as well as unsupervised learning (UL). It is mostly appropriate for tasks with delayed plunders. Concluded progressions in deep neural network training, such as the growth of the deep Q-network, reinforcement learning constructions can attain human-level regulator. [5].
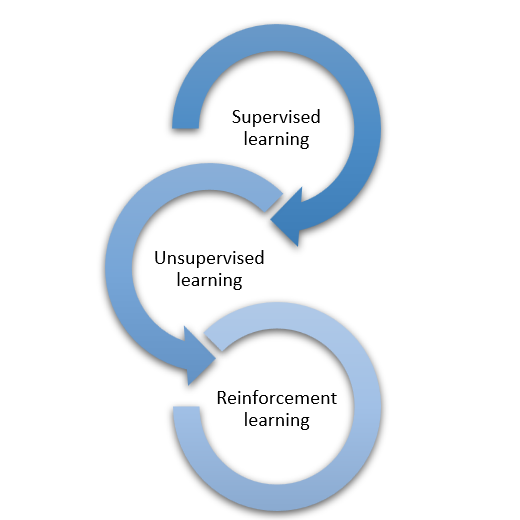
B. Application of DL
In this part, study going to state the claims of DL. DL is extensively utilised in independent schemes since of the important compensations in optimization, discrimination, as well as forecast. Due to the enormous request part classes, study present only a rare illustrative application areas [2].
i. Image and video recognition
DL’s essential research lies in image and video acknowledgement, mainly employing deep convolutional neural networks (CNN). This construction, utilizing difficulty and pooling to reduce image size, forms the basis for various study branches. Previous studies introduced a quicker CNN for real-time object detection, particularly dropping detection network running period [6].
ii. Text examination and natural language processing
It is determined by social networking as well as mobile Internet, vast human-generated data requires text analysis and natural language handing out for on-the-fly conversion and human-machine communication. DL claims to address these desires with advanced toolkits for core natural language examination [6].
iii. Finance, economics, and market analysis
DL, particularly utilized in stock trading and market models, aids as a potent predictive tool. Existing study introduced a economic time-series foretelling algorithm founded on CNN construction, representative a noteworthy reduction in forecasting error rates with forex market information [6].
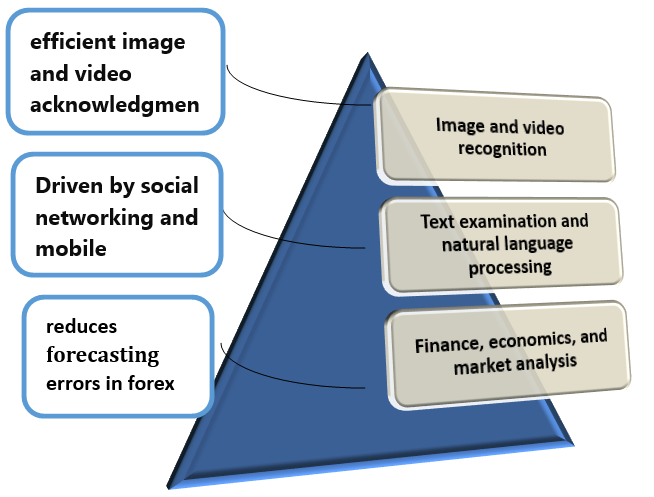
Conclusion:
In the end, the fusion of cybersecurity and artificial intelligence (AI), mainly deep learning, presents a essential advancement in digital Defence. AI models improve malware detection and threat intelligence but require devoted cybersecurity measures. The speedy development of cyberspace, determined by technologies like software-defined networking and big data, underscores the need for robust security beyond traditional methods[1]. The route of AI in cybersecurity, from multifaceted information bases to ML and DL, means a transformative expedition. DL, imitating human neural processes, is today a central focus in research, offering multipurpose applications across businesses [6].
Reference:
- M. Priyadarsini and P. Bera, “Software defined networking architecture, traffic management, security, and placement: A survey,” Comput. Netw., vol. 192, p. 108047, Jun. 2021, doi: 10.1016/j.comnet.2021.108047.
- J. Li, “Cyber security meets artificial intelligence: a survey,” Front. Inf. Technol. Electron. Eng., vol. 19, no. 12, pp. 1462–1474, Dec. 2018, doi: 10.1631/FITEE.1800573.
- M. Johnson, A. Albizri, A. Harfouche, and S. Fosso-Wamba, “Integrating human knowledge into artificial intelligence for complex and ill-structured problems: Informed artificial intelligence,” Int. J. Inf. Manag., vol. 64, p. 102479, Jun. 2022, doi: 10.1016/j.ijinfomgt.2022.102479.
- “Supervised Learning – an overview | ScienceDirect Topics.” Accessed: Mar. 06, 2024. [Online]. Available: https://www.sciencedirect.com/topics/computer-science/supervised-learning [5] “Supervised vs. Unsupervised Learning: What’s the Difference? – IBM Blog.” Accessed: Mar. 06, 2024. [Online]. Available: https://www.ibm.com/blog/supervised-vs-unsupervised-learning/
- A. Anaya-Isaza, L. Mera-Jiménez, and M. Zequera-Diaz, “An overview of deep learning in medical imaging,” Inform. Med. Unlocked, vol. 26, p. 100723, Jan. 2021, doi: 10.1016/j.imu.2021.100723.
Cite As
Walawalkar A. M. (2024) AI Safeguards: Securing the Cyber Security Landscape, Insights2Techinfo, pp.1