By: Gopal Mengi , Sudhakar Kumar
It’s only been 20 years since the twenty-first century and, certainly, one of the best momentous advancements and enabling impacts for the human culture of this century will be Artificial Intelligence[12]. It is a grounded fact that AI and related operations and stages are set to reform the global point of view, impact productivity for the better, improve lifestyle, and generate huge amounts of wealth. For instance, the research firm Gartner expects worldwide AI-based economic activities to be increased from $1.2 trillion in 2018 to about $3.9 trillion in 2022 [1][9]. AI is still in a developing stage and therefore there are questions about its accountability but explainable AI is a technology that is helping in this matter because it is very important as in healthcare the stakes are very high [6][10]. Artificial Intelligence & Machine Learning in Healthcare is very usefull and is being implemented in various forms some of which are:-
- AI is being used to address the low-level problem which is yet very effective and costs a major chunk of resources that is the administrative work that consumes a lot of time of the doctors, nurses, and other hospital staff and AI can come in handy to automate all this work and save the time of the staff to treat patients [5][14]. In the first scale, we can also include the AI applications based on imaging which are already being used in clinical fields such as radiology, pathology, etc [2].
- The second approach in which AI can be helpful is that it can be used in remote monitoring, that is if the patient is transferred from hospital to home their monitoring could be done effectively [5]. These can include AI-powered alerting systems or virtual assistants that if a patient is in need then gives an alert to the doctor. Moreover, Natural Language Processing has a clinical use that it can use to change the text over to well-organized data which then, at that point, helps in a superior comprehension of the patient’s clinical history and furthermore group patients and sum up data.
- The third phase witnessed a more refined approach towards the diagnostics part as it can be used to diagnose patients and predict/suggest a more practical and feasible cure based on the previous clinical trials and AI can also help in improving clinical decision making [5].
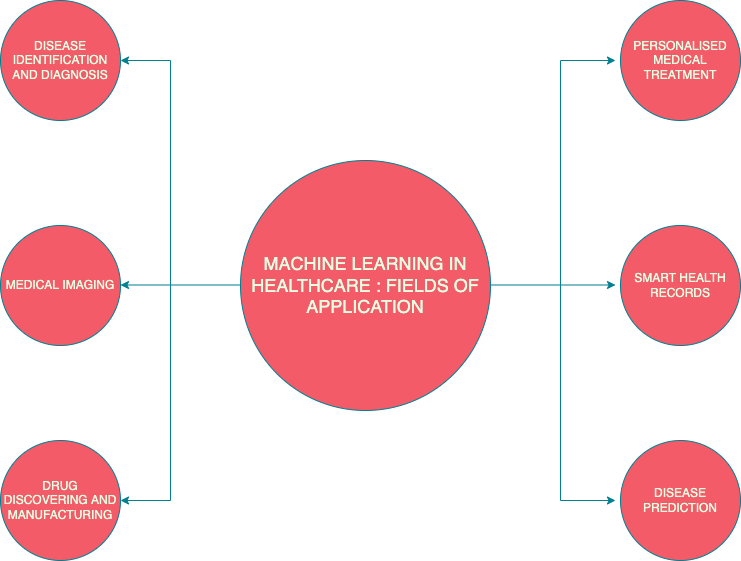
The utilization of AI in demonstrative clinical imaging is going through extensive assessment. Artificial intelligence has shown amazing accuracy and responsiveness in identifying and imaging abnormalities and vows to improve tissue-based detection and characterisation. Traditional methods/techniques in the field of artificial intelligence largely depend on the predefined algorithms which use feature engineering based on the previous results and conclusions[11][13]. These features are utilised to plan 3D imaging of growth or the intratumoral surface and dispersion of pixel powers. Authentic AI models then train this data to perceive potential imaging-based biomarkers. One of the examples includes Support Vector Machine (SVM)[3]. Recently, pixel/voxel-based has been discovered in medical imaging which does not require the explicit input of features thus reducing the scope of error and giving more precise results [8]. Also, deep learning technologies such as convolutional neural networks have been showing great progress in the restructuring of images and improving the resolution which is being used in a more precise view of the human body scans [7].
Although AI is being implemented in various aspects in terms of healthcare. Following are a few Artificial Intelligence & Machine Learning in Healthcare applications that are showing promising results [15-17]:-
- Thoracic Imaging: Cellular breakdown in the lungs is quite possibly the most well-known and dangerous tumour. Cell breakdown in the lungs screening can help with perceiving aspiratory nodules and with early acknowledgment has proven to be lifesaving in various patients. AI can be useful in recognizing these nodules and characterising them as harmless or dangerous [3].
- Colonoscopy/Sigmoidoscopy: Polyps in the colon that are unable to be detected or are incorrectly identified address a potential danger of colorectal harmful development. Be that as it may, most polyps are from the start harmless, they can become risky over the long haul. Hereafter, early acknowledgment and consistent seeing with AI-based instruments would be exceptionally valuable [3].
- Brain Imaging: Whether malignant, benign, or asymptomatic, brain tumours display abnormal tissue growth; Artificial Intelligence makes it possible to predict their presence as well as their prognosis [3].
- DNA Sequencing: With the increasing availability of sequencing data, genomic endpoints are becoming increasingly useful in diagnosing cancer. Tools based on AI can analyse and extract features that can be useful in correlating somatic point mutations with cancer types as well can be useful in predicting the genetic mutations [3].
References
- Sarkar, T. (2021, August 19). AI and Machine Learning for Healthcare. Medium. Retrieved February 12, 2022, from https://towardsdatascience.com/ai-and-machine-learning-for-healthcare-7a70fb3acb67
- Mohammed Yousef Shaheen. AI in Healthcare: medical and socio-economic benefits and challenges. ScienceOpen Preprints. DOI: 10.14293/S2199-1006.1.SOR-.PPRQNI1.v1
- Hosny A, Parmar C, Quackenbush J, Schwartz LH, Aerts HJWL. Artificial intelligence in radiology. Nat Rev Cancer. 2018;18(8):500-510. doi:10.1038/s41568-018-0016-5
- Goldenberg, S., Nir, G. & Salcudean, S.E. A new era: artificial intelligence and machine learning in prostate cancer. Nat Rev Urol 16, 391–403 (2019). https://doi.org/10.1038/s41585-019-0193-3
- Spatharou, A., Hieronimus, S., & Jenkins, J. (2021, July 1). Transforming healthcare with ai: The impact on the workforce and Organizations. McKinsey & Company. Retrieved February 12, 2022, from https://www.mckinsey.com/industries/healthcare-systems-and-services/our-insights/transforming-healthcare-with-ai
- U. Pawar, D. O’Shea, S. Rea, and R. O’Reilly, “Explainable AI in Healthcare,” 2020 International Conference on Cyber Situational Awareness, Data Analytics and Assessment (CyberSA), 2020, pp. 1-2, DOI: 10.1109/CyberSA49311.2020.9139655.
- Kim, Mingyu et al. “Deep Learning in Medical Imaging.” Neurospine vol. 16,4 (2019): 657-668. doi:10.14245/ns.1938396.198
- Kenji Suzuki. 2012. Pixel-based machine learning in medical imaging.Journal of Biomedical Imaging 2012, Article 1 (January 2012), 1 pages. DOI:https://doi.org/10.1155/2012/792079
- Inderpreet Singh, Sunil Kr. Singh, Sudhakar Kumar, Kriti Aggarwal (2021), “Dropout-VGG based Convolutional Neural Network for Traffic Sign Categorization”, in the proc. of 2nd Congress on Intelligent Systems (CIS 2021), Lecture Notes on Data Engineering And Communication Technologies. Springer, Berlin, Heidelberg.
- Aggarwal, K., Singh, S. K., Chopra, M., & Kumar, S. (2022). Role of Social Media in the COVID-19 Pandemic: A Literature Review. In B. Gupta, D. Peraković, A. Abd El-Latif, & D. Gupta (Ed.), Data Mining Approaches for Big Data and Sentiment Analysis in Social Media (pp. 91-115). DOI-10.4018/978-1-7998-8413-2.ch004
- Chopra, M., Singh, S. K., Aggarwal, K., & Gupta, A. (2022). Predicting Catastrophic Events Using Machine Learning Models for Natural Language Processing. In B. Gupta, D. Peraković, A. Abd El-Latif, & D. Gupta (Ed.), Data Mining Approaches for Big Data and Sentiment Analysis in Social Media (pp. 223-243). IGI Global. https://www.igi-global.com/gateway/chapter/293158.
- Chopra, M., Singh, S. K., Aggarwal, MLOps: A New Era of DevOps, Powered by Machine Learning. Insights2Techinfo. Retrieved February 12, 2022, from
- Chopra, M., Singh, S. K., Mengi G. & Gupta D.,(2021) Assess and Analysis Covid-19 Immunization Process: A Data Science Approach to make India self-reliant and safe. International Conference on Smart Systems and Advanced Computing (Syscom-2021), December 25–26, 2021. CEUR Workshop Proceedings (CEUR-WS.org). 30 January 2022. URL: http://ceur-ws.org/Vol-3080/10.pdf
- Chopra, M., Singh, S., Sharma, S. & Mahto, D., 2022. Impact and Usability of Artificial Intelligence in Manufacturing workflow to empower Industry 4.0., International Conference on Smart Systems and Advanced Computing (Syscom-2021), December 25–26, 2021. CEUR Workshop Proceedings (CEUR-WS.org). 30 January 2022. URL: http://ceur-ws.org/Vol-3080/4.pdf.
- Gupta, B. B., Tewari, A., Cvitić, I., Peraković, D., & Chang, X. (2021). Artificial intelligence empowered emails classifier for Internet of Things based systems in industry 4.0. Wireless networks, 1-11.
- Nguyen, G. N., Le Viet, N. H., et al. (2021). Secure blockchain enabled Cyber–physical systems in healthcare using deep belief network with ResNet model. Journal of Parallel and Distributed Computing, 153, 150-160.
- Gupta, B. B., Prajapati, V., Nedjah, N., Vijayakumar, P., El-Latif, A. A. A., & Chang, X. (2021). Machine learning and smart card based two-factor authentication scheme for preserving anonymity in telecare medical information system (TMIS). Neural Computing and Applications, 1-26.
Cite this article as:
Gopal Mengi; Sudhakar Kumar (2022) Artificial Intelligence and Machine Learning in Healthcare, Insights2Tecinfo, pp. 1