By: Pinaki Sahu, International Center for AI and Cyber Security Research and Innovations (CCRI), Asia University, Taiwan, 0000pinaki1234.kv@gmail.com
Phishing attacks are still a threat, and they are changing all the time. To protect private information, you need to be able to identify and stop them right away. The article explains how deep learning methods can be added to chatbots to help them detect and stop hacking attacks better. Due to its natural ability to recognize patterns, deep learning is an optimistic strategy to stop hacking attempts that are getting smarter. This article delves into the principles, benefits, challenges, and real-world applications of deep learning-powered chatbots in the fight against phishing.
Introduction: The Continuous Risk of Phishing Attacks
People and businesses are still being targeted by phishing attacks, which use well-designed emails, websites, and social engineering to get private information. Cybercriminals are always changing their tactics, so old defenses like spam filters and rule-based systems can’t keep up. To stop phishing attacks successfully, we need to be able to find and stop them in real time.
Deep Learning’s Role in Cybersecurity
Deep learning is a type of artificial intelligence that has become popular in many areas because it is so good at recognizing patterns[1]. Deep neural networks, like convolutional neural networks (CNNs) and recurrent neural networks (RNNs), are used to handle data and learn from it[2]. This makes it perfect for tasks that need to find trends that are complicated and change over time.
How Deep Learning Is Being Used in Chatbots
Conversational assistance based on AI, or chatbots, have grown beyond simple customer service tools. They can now be combined with deep learning systems to help users spot fake attacks in real-time. These robots look at emails, texts, or websites that come in and compare them to known signs of phishing. They can then warn users or take steps to stop hacking[3].
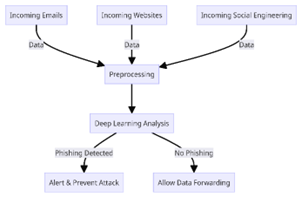
Challenges and Limitations
Bringing deep learning and chatbots together has a lot of potential, but there are also some problems to think about. Some of these are the need for a lot of computer power, the chance of fake positives or negatives, and the need to make sure that the model is always being updated to deal with new hacking techniques[5].
The Future of Chatbots Powered by AI in Phishing Defence
As hacking tactics change, chatbots that use deep learning are going to have a bigger part in the future of defense. Not only will these systems find attacks, but they will also teach people about possible risks and make them more aware of them. They may also be very important for gathering large amounts of dangerous information.
Conclusion
Phishing attacks are a persistent hazard that requires innovative solutions. Using their pattern recognition capabilities, chatbots powered by deep learning provide a strong defense against these attacks. Their ability to analyze and respond to phishing attempts in real time improves overall cybersecurity efforts, making them an invaluable asset in the ongoing fight against cybercrime. As technology evolves, the incorporation of deep learning into chatbots represents a promising strategy for enhancing online security.
References
- Csaky, R. (2019). Deep learning based chatbot models. arXiv preprint arXiv:1908.08835.
- Karri, S. P. R., & Kumar, B. S. (2020, January). Deep learning techniques for implementation of chatbots. In 2020 International conference on computer communication and informatics (ICCCI) (pp. 1-5). IEEE.
- Yoo, J., & Cho, Y. (2022). ICSA: Intelligent chatbot security assistant using Text-CNN and multi-phase real-time defence against SNS phishing attacks. Expert Systems with Applications, 207, 117893.
- Alconbury, M., Alaca, F., & Chiasson, S. (2015). Why phishing still works: User strategies for combating phishing attacks. International Journal of Human-Computer Studies, 82, 69-82.
- Sahoo, S. R., & Gupta, B. B. (2019). Hybrid approach for detection of malicious profiles in twitter. Computers & Electrical Engineering, 76, 65-81.
- Gupta, B. B., Yadav, K., Razzak, I., Psannis, K., Castiglione, A., & Chang, X. (2021). A novel approach for phishing URLs detection using lexical based machine learning in a real-time environment. Computer Communications, 175, 47-57.
- Cvitić, I., Perakovic, D., Gupta, B. B., & Choo, K. K. R. (2021). Boosting-based DDoS detection in internet of things systems. IEEE Internet of Things Journal, 9(3), 2109-2123.
- Alieyan, K., Almomani, A., Anbar, M., Alauthman, M., Abdullah, R., & Gupta, B. B. (2021). DNS rule-based schema to botnet detection. Enterprise Information Systems, 15(4), 545-564.
- Deveci, M., Pamucar, D., Gokasar, I., Köppen, M., & Gupta, B. B. (2022). Personal mobility in metaverse with autonomous vehicles using Q-rung orthopair fuzzy sets based OPA-RAFSI model. IEEE Transactions on Intelligent Transportation Systems.
Cite As
Sahu P. (2023) Deep Learning Chatbot Assistance for Real-Time Phishing Attack Detection, Insights2Techinfo, pp.1