By: Jampula Navaneeth1
1Vel Tech University, Chennai, India
2International Center for AI and Cyber Security Research and Innovations, Asia University, Taiwan Email: navaneethjampula@gmail.com
Abstract
New and better algorithms in the ever-expanding field of cybersecurity is deep learning, for sophisticated solutions in predicting and countering threats. This paper aims at discussing how deep learning can be used in identification of advanced threats, for instance; phishing, malware, and network intrusion. Thus, deep learning harnessing the power of bulk data and revealing the underlying structures lets predict possible threats and develop prevent measures. Advancing beyond simple methods of identification and remediation of attacks after they occur, deep learning enables organizations to stop attacks from happening, improving the security position as well as defining new trends in the sphere of cyber protection.
Introduction
The proliferation of the digital environment is, therefore, coupled with the proliferation of cyber threats, and a corresponding demand for enhanced protective measures. Traditional strategies and security tactics with social engineering still remain effective up to some extent, however, they are purely based on end user point in time security and defensive posturing once a risk has occurred [1]. On the other hand, deep learning is a different architectural approach to the problem by providing a possibility to prevent threats before they happen.
Being a part of machine learning, deep learning utilizes neural networks to interpret massive volumes of inputs and then determine possible cyber threats. Such capability can help to detect the risks before they occur and prevent them in the process of their development [2]. In this article, we outline how deep learning is changing cybersecurity from being simply a predictive field, to one that is preventative in nature – that is, one which seeks to prevent cyber-crime at its source.
The Role of Deep Learning in Cyber Security
The subfield of machine learning known as deep learning utilization high neural network multi-layered structures to mimic complex data arrangements. In cybersecurity this provides the opportunity of detecting different type of threats such as phishing, malware, ransomware and network intrusion in improved way. Deep learning ability to work with big data at real time enhances the field’s capability in responding to the dynamic threats [3].
Deep learning models are therefore poised to find biological meanings and patterns from large datasets that could be very difficult for traditional rule-based systems as well as basic or even advanced machine learning algorithms [4]. This leads to better prediction of adversarial actions which in turn empowers the Security Operations Center to be able to contain any future cyber threats with efficiency [5].
Prediction: The First Line of Defense
They have certain underlying uses that is threat prediction is one of the key tasks solved at the core of deep learning [6]. This includes, pattern and anomaly detection and trend analysis on large sets of data that would show a possibility of a cyber-attack.
For instance, in the application of phishing detection deep learning models are used to predict future nuisances of phishing attempts via an analysis of emails, URLs, and user behavior that exhibits features of phishing scams. Likewise, in case of malware, it is possible for deep learning models to analyze binary code and file structures or network traffic to detect if a program is malicious even that it is not in their database [1].
They are powerful prediction capabilities that afford organizations an opportunity to respond proactively before an attack emerges. Deep learning early warning system gives confidence to security professionals to prevent potential risks than to counter an actual incident.
From Prediction to Prevention: The Next Leap
Of course, prediction is useful, but the aim of the contemporary process of safeguarding is to prevent and to ensure the threat does not occur in the first place. Deep learning presents a way to this more undoubtedly proactive countermeasure. But of course, once an attack pattern has been identified to predict future attacks, the system is capable of launching early intervention.
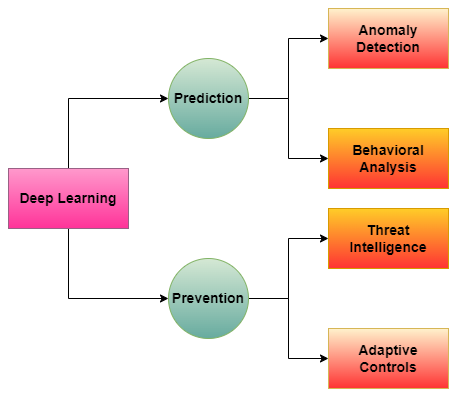
For example, in the area of network security, deep learning models can change a firewall in real time, quarantine infected machines, even modify the routing of the network in an attempt to contain an attack. Concerning phishing, and through deep learning algorithms, the suspicious emails or URL can be efficiently filtered and blocked from ever reaching the user [7].
Further, deep learning helps endpoint security in observing the endpoint devices for possible malicious activity, an approach that enables for timely response to and neutralization of threats [8]. These systems can even become intelligent enough with the help of AI to adapt to the new threats which are used by the cybercriminals, thanks to integrating intelligence into the system.
Challenges
Although deep learning has good prospects in relation to cybersecurity, it is important to have some appreciation of its problems. One major problem is that of adversarial examples, with the ability of such individuals to place malicious inputs into deep learning models which will cause errors or missed threats. However, deep learning models tend to demand significant computing resources and the availability of large datasets to perform effectively and this represent a challenge to some organizations [2].
However, the future is bright for Deep learning in cybersecurity advancement, given the challenges mentioned above. There’s no doubt that deep learning will continue to develop as more work is done into adversarial defenses and more efficient model architectures. As the AI and deep learning tools become more advanced, one can only imagine the more advanced techniques for detecting and preventing the cyber threats, and the world will become more secure to use online.
Conclusion
Deep learning has quickly integrated into cybersecurity by shifting from being just a predictive technology to the preventive one. Deep learning is the best solution for detecting and mitigating cyber risks in real-time due to it characteristics of analyzing large amount of data and recognizing various patterns. With the escalating threats and frequency of cyber threats further the role of deep learning as key to the security and integrity of digital structures.
References
- P. Dixit and S. Silakari, “Deep Learning Algorithms for Cybersecurity Applications: A Technological and Status Review,” Computer Science Review, vol. 39, p. 100317, Feb. 2021, doi: 10.1016/j.cosrev.2020.100317.
- Y. N. Imamverdiyev and F. J. Abdullayeva, “Deep Learning in Cybersecurity: Challenges and Approaches,” IJCWT, vol. 10, no. 2, pp. 82–105, Apr. 2020, doi: 10.4018/IJCWT.2020040105.
- M. Macas, C. Wu, and W. Fuertes, “A survey on deep learning for cybersecurity: Progress, challenges, and opportunities,” Computer Networks, vol. 212, p. 109032, Jul. 2022, doi: 10.1016/j.comnet.2022.109032.
- Z. Chen, “Deep Learning for Cybersecurity: A Review,” in 2020 International Conference on Computing and Data Science (CDS), Aug. 2020, pp. 7–18. doi: 10.1109/CDS49703.2020.00009.
- P. Pappachan, N. S. Adi, G. Firmansyah, and M. Rahaman, “Deep Learning-Based Forensics and Anti-Forensics,” in Digital Forensics and Cyber Crime Investigation, CRC Press, 2024.
- “Towards Security Threats of Deep Learning Systems: A Survey | IEEE Journals & Magazine | IEEE Xplore.” Accessed: Sep. 30, 2024. [Online]. Available: https://ieeexplore.ieee.org/abstract/document/9252914
- E. Rodríguez, B. Otero, N. Gutiérrez, and R. Canal, “A Survey of Deep Learning Techniques for Cybersecurity in Mobile Networks,” IEEE Communications Surveys & Tutorials, vol. 23, no. 3, pp. 1920–1955, 2021, doi: 10.1109/COMST.2021.3086296.
- Y. Shen, E. Mariconti, P. A. Vervier, and G. Stringhini, “Tiresias: Predicting Security Events Through Deep Learning,” in Proceedings of the 2018 ACM SIGSAC Conference on Computer and Communications Security, Toronto Canada: ACM, Oct. 2018, pp. 592–605. doi: 10.1145/3243734.3243811.
- Sharma, P. C., Mahmood, M. R., Raja, H., Yadav, N. S., Gupta, B. B., & Arya, V. (2023). Secure authentication and privacy-preserving blockchain for industrial internet of things. Computers and Electrical Engineering, 108, 108703.
- Tan, H., Gu, Z., Wang, L., Zhang, H., Gupta, B. B., & Tian, Z. (2022). Improving adversarial transferability by temporal and spatial momentum in urban speaker recognition systems. Computers and Electrical Engineering, 104, 108446.
- Zhou, Z., Wang, M., Ni, Z., Xia, Z., & Gupta, B. B. (2021). Reliable and sustainable product evaluation management system based on blockchain. IEEE Transactions on engineering management.
Cite As
Navaneeth J. (2024) Deep Learning in Cybersecurity: From Prediction to Prevention, Insights2Techinfo, pp.1