By: Kwok Tai Chui, Hong Kong Metropolitan University (HKMU) , Hong Kong
The integration of deep learning into the field of healthcare is nothing short of revolutionary. In our blog post, we delve into the transformative power of deep learning algorithms and neural networks within the medical realm. This exploration begins with an in-depth understanding of deep learning and its inherent capabilities. From there, we investigate the myriad applications of deep learning in healthcare, disease diagnosis, drug discovery, and treatment planning. This blog post serves as a comprehensive guide to the impact of deep learning in healthcare, providing readers with insights, examples, and an understanding of the boundless potential it holds for revolutionizing diagnosis and treatment. Join us on this journey into the future of medicine powered by deep learning.
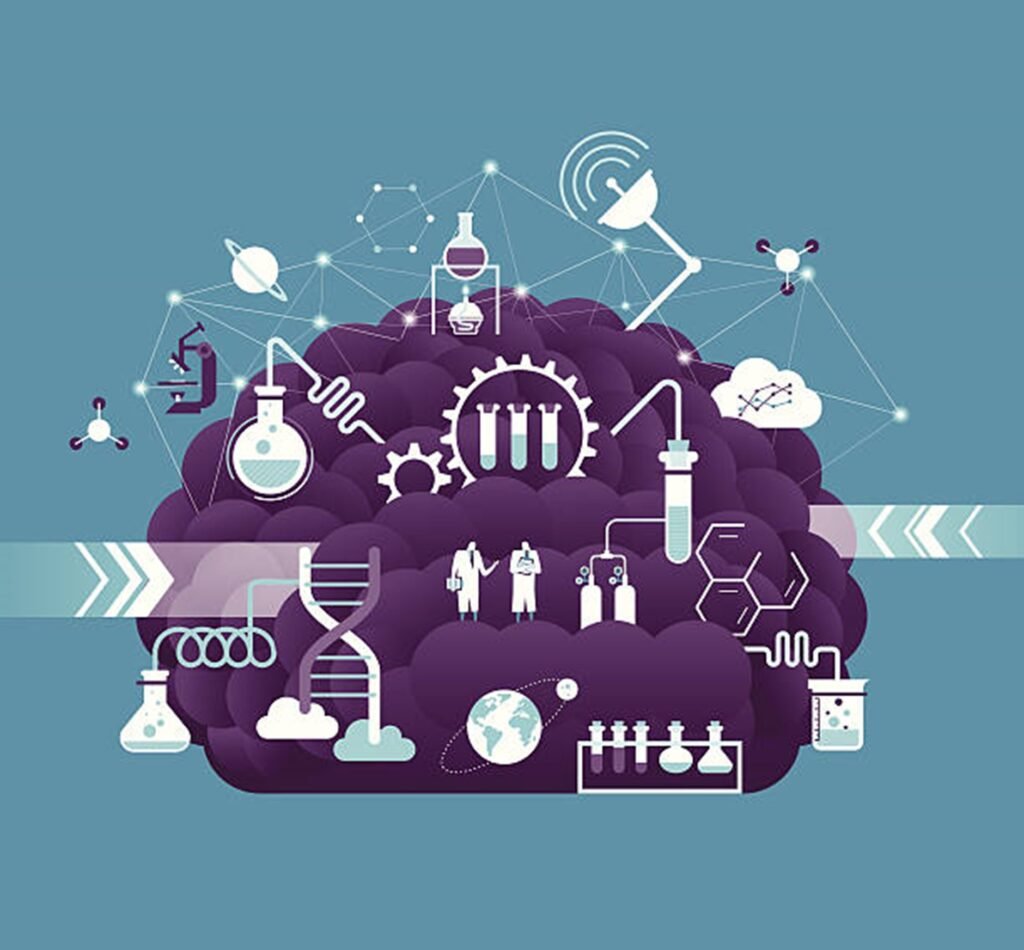
Table 1: Applications of Deep Learning in Healthcare
Application | Description |
Medical Imaging | – Radiology and deep learning – Pathology image analysis |
Disease Diagnosis | – Early detection of diseases – Predictive analytics for chronic conditions |
Drug Discovery | – Accelerating drug development – Target identification and drug repurposing |
Treatment Planning | – Personalized treatment recommendations – Clinical decision support systems |
Deep learning in healthcare
Deep learning is a branch of machine learning that utilizes artificial neural networks to represent and analyze data. It is a technique that has gained significant attention and popularity in various fields, including healthcare. Deep learning has been recognized as an excellent decision model tool for the control of Internet of Medical Things (IoMT) applications [9]. It has demonstrated state-of-the-art results in computer vision and machine learning tasks [2]. Unlike traditional machine learning algorithms, deep learning does not require manual feature engineering, as it automatically discovers features from the given data [4]. This makes it a powerful tool for processing and analyzing complex healthcare data.
The significance and impact of deep learning in the medical field are substantial. Deep learning has the potential to revolutionize healthcare by improving diagnosis, treatment, and patient care. In the field of medical image analysis, deep learning has achieved remarkable achievements, including the recognition and segmentation of lesion tissues [3]. It has been used in various medical imaging scenarios, such as pathology and dermatology, and has exhibited excellent performance [3]. Deep learning models trained on large amounts of medical image data have shown high accuracy in tasks such as skin cancer classification [8]. However, the field of medical image analysis faces challenges due to the lack of sufficient labeled data and the need for manual annotation [8]. Transfer learning approaches have been proposed to overcome these challenges by leveraging pre-trained models and fine-tuning them on limited labeled data [8].
Deep learning is also being applied in healthcare monitoring and motion detection. Inertial measurement units (IMUs) combined with deep learning algorithms have been used to detect and analyze human motion patterns for smart healthcare learning tools [6]. This has potential applications in areas such as ambient assisted living and physical therapy [6]. Deep learning has also been utilized in the development of intelligent mosquito nets for the control of mosquito-borne diseases [1]. By combining deep learning algorithms with sensor data, the intention of the user to open or close the mosquito net can be automatically determined [1].
Overall, deep learning has significant potential in healthcare due to its ability to process and analyze complex data, improve accuracy in medical image analysis, and enable intelligent monitoring and detection of human motion patterns. However, challenges such as the need for large labeled datasets and the mismatch between learned features in natural images and medical images need to be addressed to fully harness the power of deep learning in healthcare [8]. Further research and advancements in deep learning techniques and applications are needed to fully realize its impact in the medical field.
Accelerating drug development with deep learning
Deep learning has emerged as a powerful tool for accelerating drug development. By leveraging large datasets and complex algorithms, deep learning can analyze vast amounts of biomedical data and identify potential drug candidates for further investigation. One application of deep learning in drug development is drug repurposing, where existing drugs are evaluated for their potential to treat new diseases. For example, in the context of COVID-19, deep learning has been used to prioritize existing drugs for further investigation as potential therapeutics [11]. This approach can significantly speed up the drug development process by identifying drugs that have already undergone safety testing and may have known mechanisms of action. Additionally, deep learning can assist in the design of new drug molecules by predicting their properties and interactions with biological targets. By utilizing deep learning algorithms, researchers can generate virtual libraries of potential drug candidates and prioritize those with the highest likelihood of success [13]. Overall, deep learning has the potential to revolutionize drug development by accelerating the identification and optimization of potential drug candidates, ultimately leading to more efficient and effective treatments for various diseases [11]
Personalized treatment recommendations
Personalized treatment recommendations using deep learning have the potential to revolutionize healthcare by tailoring treatment plans to individual patients. Deep learning algorithms can analyze large amounts of patient data, including medical records, genetic information, and treatment outcomes, to identify patterns and make personalized treatment recommendations. By considering a patient’s unique characteristics and medical history, deep learning models can predict the most effective treatment options and optimize patient outcomes. For example, in cancer treatment, deep learning algorithms have been used to analyze genomic data and predict the response to different therapies, enabling oncologists to make more informed decisions about personalized treatment plans [15]. Additionally, deep learning can assist in predicting adverse drug reactions and identifying patients who may be at higher risk, allowing for proactive measures to be taken to prevent potential harm [15]. Overall, personalized treatment recommendations using deep learning have the potential to improve patient outcomes, reduce healthcare costs, and enhance the overall quality of care in the medical field.
Clinical decision support systems
Clinical decision support systems (CDSS) using deep learning have emerged as valuable tools in healthcare for aiding clinicians in making informed decisions. Deep learning algorithms can analyze large volumes of patient data, including medical records, laboratory results, and imaging data, to provide real-time recommendations and predictions. These systems can assist in various clinical tasks, such as disease diagnosis, treatment planning, and prognosis prediction. For example, in the field of oncology, deep learning-based CDSS have been developed to predict the grading of hepatocellular carcinoma, a type of liver cancer Wei et al. [17]. By leveraging multi-scale and multi-region analysis, as well as attention mechanisms, these systems can accurately predict the grading of hepatocellular carcinoma and assist clinicians in determining appropriate treatment strategies. The integration of deep learning into CDSS has the potential to enhance clinical decision-making, improve patient outcomes, and optimize healthcare resource utilization. However, challenges such as the need for large and diverse datasets, interpretability of deep learning models, and validation in real-world clinical settings need to be addressed for the widespread adoption of deep learning-based CDSS in healthcare.
Limitations and challenges
Table 2: Challenges and Limitations
Challenge/Limitation | Description |
Ethical Concerns | Privacy issues, bias in algorithms |
Data Quality Issues | Incomplete or noisy healthcare data |
Model Interpretability | Difficulty in understanding deep learning decisions |
Data Quality Issues: Deep learning models rely heavily on large, high-quality datasets for training, yet obtaining such data in healthcare can be an arduous task. Healthcare datasets are often fragmented, incomplete, or noisy, which can hinder the performance of deep learning algorithms. Furthermore, class imbalance is a common issue, where certain medical conditions are rare compared to others. This imbalance can lead to biased models and poor generalization, as the model may become overly biased toward the majority class, neglecting the minority class.
Ethical Concerns: Ethical considerations loom large in healthcare, particularly when handling sensitive patient information. Patient privacy is paramount, and deep learning’s hunger for data can raise concerns about data security and compliance with regulations such as HIPAA (Health Insurance Portability and Accountability Act) in the United States. Additionally, bias in AI algorithms is a pressing ethical challenge. Biased training data can perpetuate inequalities and lead to discriminatory outcomes, particularly concerning patients from diverse backgrounds.
Interpretability and Explainability: The “black-box” nature of deep learning models can be a double-edged sword. While these models excel at complex tasks, understanding their decision-making processes can be challenging. This lack of interpretability raises concerns in healthcare, where clinicians and patients need to trust and comprehend the reasoning behind medical recommendations. Ensuring that deep learning models provide transparent explanations for their predictions is vital for gaining acceptance and building trust.
Generalization Challenges: Deep learning models trained on data from specific populations may struggle to generalize effectively to diverse patient groups. This limitation can result in inaccuracies in diagnosis or treatment recommendations when applied to patients with different demographic characteristics or medical histories. Adverse events stemming from such generalization challenges could have serious consequences for patient care.
Regulatory Compliance: Complying with healthcare regulations is crucial when implementing deep learning solutions. Healthcare is heavily regulated to safeguard patient information and ensure the highest standards of care. Navigating the regulatory landscape, which varies by region, can be complex and time-consuming, requiring meticulous attention to detail when developing and deploying deep learning systems in healthcare settings.
Resource Intensiveness: The resource requirements for deep learning can be daunting. Training and running deep learning models demand substantial computational power and storage capacity, which may not be readily available in all healthcare institutions, particularly smaller or underfunded ones. Additionally, the cost associated with infrastructure, data acquisition, and model development can be prohibitive for some organizations.
Integration Challenges: Integrating deep learning solutions into existing healthcare systems and workflows can be technically challenging. It often requires significant changes to established practices and careful consideration of how AI-driven insights align with the responsibilities and expertise of healthcare professionals. Achieving seamless integration without disrupting patient care is a persistent challenge.
Conclusion
In the rapidly evolving landscape of healthcare, the integration of deep learning has emerged as a revolutionary force, holding the promise of transforming the way we diagnose and treat medical conditions. As we’ve explored in this blog post, deep learning’s applications in medical imaging, disease diagnosis, drug discovery, and treatment planning have already begun to yield remarkable results. Real-world case studies have highlighted its potential to enhance accuracy, efficiency, and patient outcomes. However, the journey toward fully realizing the potential of deep learning in healthcare is not without its challenges and limitations. Data quality issues, ethical concerns, and the inherent black-box nature of deep learning models all demand careful consideration. Moreover, the ability to generalize findings across diverse patient populations and navigate complex regulatory environments remains pivotal.
References
- Y. Liu, X. Wang, X. She, M. Yi, Y. Li, & F. Jiang, “Design of intelligent mosquito nets based on deep learning algorithms“, Computers Materials & Continua, vol. 69, no. 2, p. 2261-2276, 2021.
- Y. Lee, J. Kwon, Y. Lee, H. Park, H. Cho, & J. Park, “Deep learning in the medical domain: predicting cardiac arrest using deep learning“, Acute and Critical Care, vol. 33, no. 3, p. 117-120, 2018.
- L. Wu, J. Zhuang, W. Chen, Y. Tang, C. Hou, C. Liet al., “Data augmentation based on multiple oversampling fusion for medical image segmentation“, Plos One, vol. 17, no. 10, p. e0274522, 2022.
- S. Roopashree and J. Anitha, “Deepherb: a vision based system for medicinal plants using xception features“, Ieee Access, vol. 9, p. 135927-135941, 2021.
- Rajput, R. K. S., Goyal, D., Pant, A., Sharma, G., Arya, V., & Rafsanjani, M. K. (2022). Cloud data centre energy utilization estimation: Simulation and modelling with idr. International Journal of Cloud Applications and Computing (IJCAC), 12(1), 1-16.
- F. Hajjej, M. Javeed, A. Ksibi, M. Alarfaj, K. Alnowaiser, A. Jalalet al., “Deep human motion detection and multi-features analysis for smart healthcare learning tools“, Ieee Access, vol. 10, p. 116527-116539, 2022.
- Wang, L., Li, L., Li, J., Li, J., Gupta, B. B., & Liu, X. (2018). Compressive sensing of medical images with confidentially homomorphic aggregations. IEEE Internet of Things Journal, 6(2), 1402-1409.
- L. Alzubaidi, M. Al-Amidie, A. Al-Asadi, A. Humaidi, O. Al-Shamma, M. Fadhelet al., “Novel transfer learning approach for medical imaging with limited labeled data“, Cancers, vol. 13, no. 7, p. 1590, 2021. https://doi.org/10.3390/cancers13071590
- W. Al-Sit, N. Al-Dmour, T. Ghazal, & G. Issa, “Iomt-based healthcare framework for ambient assisted living using a convolutional neural network“, Computers Materials & Continua, vol. 74, no. 3, p. 6867-6878, 2023.
- Miri, M., Dowlatshahi, M. B., Hashemi, A., Rafsanjani, M. K., Gupta, B. B., & Alhalabi, W. (2022). Ensemble feature selection for multi‐label text classification: An intelligent order statistics approach. International Journal of Intelligent Systems, 37(12), 11319-11341.
- Zeng, X., Song, X., Ma, T., Pan, X., Zhou, Y., Hou, Y., … & Cheng, F. (2020). Repurpose open data to discover therapeutics for covid-19 using deep learning. Journal of Proteome Research, 19(11), 4624-4636.
- Dahiya, A., Gupta, B. B., Alhalabi, W., & Ulrichd, K. (2022). A comprehensive analysis of blockchain and its applications in intelligent systems based on IoT, cloud and social media. International Journal of Intelligent Systems, 37(12), 11037-11077.
- Yarragunta, A. (2022). The future of pharmaceuticals: ai drug discovery & covid-19 treatments. Journal of Student Research, 11(3).
- Peñalvo, F. J. G., Maan, T., Singh, S. K., Kumar, S., Arya, V., Chui, K. T., & Singh, G. P. (2022). Sustainable Stock Market Prediction Framework Using Machine Learning Models. International Journal of Software Science and Computational Intelligence (IJSSCI), 14(1), 1-15.
- Tang, H., Wang, Q., & Jiang, G. (2022). Discrete dynamic modeling analysis based on english learning motivation. Mathematical Problems in Engineering, 2022, 1-9.
- Srivastava, D., Chui, K. T., Arya, V., Peñalvo, F. J. G., Kumar, P., & Singh, A. K. (2022). Analysis of Protein Structure for Drug Repurposing Using Computational Intelligence and ML Algorithm. International Journal of Software Science and Computational Intelligence (IJSSCI), 14(1), 1-11.
- Wei, J., Ji, Q., Gao, Y., Yang, X., Guo, D., Gu, D., … & Ding, D. (2022). A multi‐scale, multi‐region and attention mechanism‐based deep learning framework for prediction of grading in hepatocellular carcinoma. Medical Physics, 50(4), 2290-2302.
- Pathoee, K., Rawat, D., Mishra, A., Arya, V., Rafsanjani, M. K., & Gupta, A. K. (2022). A cloud-based predictive model for the detection of breast cancer. International Journal of Cloud Applications and Computing (IJCAC), 12(1), 1-12.
- Chui, K. T., Kochhar, T. S., Chhabra, A., Singh, S. K., Singh, D., Peraković, D., … & Arya, V. (2022). Traffic accident prevention in low visibility conditions using vanets cloud environment. International Journal of Cloud Applications and Computing (IJCAC), 12(1), 1-21.
Cite As
Chui K.T. (2023), Deep Learning in Healthcare Revolutionizing Diagnosis and Treatment, Insights2Techinfo, pp.1