By: Achit Katiyar1,2
1South Asian University, New Delhi, India.
2International Center for AI and Cyber Security Research and Innovations, Asia University, Taiwan. Email: achitktr@gmail.com
Abstract
Blockchain technology, observed for its independent and unchangeable database, has serious security issues, especially with the introduction of quantum computing. Quantum-inspired evolutionary algorithms (QIEAs) provide interesting methods for improving blockchain security by harnessing quantum computing concepts to boost adaptability and efficiency. This article explores the use of QIEAs to secure blockchain networks, outlining its advantages, problems, and future perspectives.
Introduction
Blockchain technology has changed data integrity and transaction security across industries, from banking to supply chain management [1]. However, the increasing threat of quantum computing threatens present encryption approaches utilized in blockchain systems [2]. Quantum-inspired evolutionary algorithms (QIEAs), which incorporate quantum computing ideas into evolutionary algorithms, represent a unique way to improving blockchain security. Figure 1 shows the classification of blockchain security. This article discusses the principles of QIEAs, their applicability in blockchain security, and the obstacles associated with their implementation.
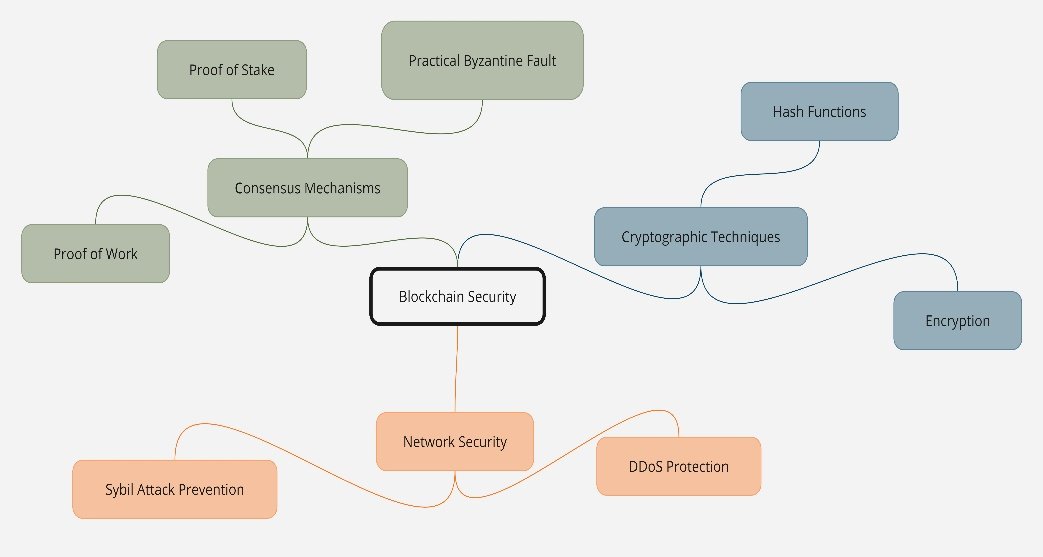
Quantum-inspired evolutionary algorithms
Quantum-inspired evolutionary algorithm (QIEA) is a group of recombinative algorithms originated from the basic principles of evolutionary algorithms; the examples of such implementation could be superposition or entanglement similar to the concept in quantum computing [3]. It is noted that some of these algorithms work with so called qubits or quantum bits and therefore, in the sense of search and optimization algorithms, they can work with many states simultaneously [4], [5].
- Quantum Genetic Algorithms (QGA):
QGAs are enhancements of basic GAs because all methods used are based on quantum theory in order to enhance exploitation of the areas of the searching space[3].
Application in Blockchain Security-
QGAs can improve the performances of cryptographic keys and consensus rules of a blockchain which in turn exposes the blockchain network to quantum assaults [3]. Regarding new security threats which may develop, organisations provide relative flexibility, and therefore, provide the necessary degree of protection to the systems based on the blockchain [4].
- Quantum Inspired Particle Swarm Optimization (QPSO):
The QPSO is another optimization algorithm that is related to the PSO due to the fact that it adopted quantum mechanics and also provided the particles with search possibilities in the solution spaces [6].
Application in Blockchain Security-
n this way, with the support of the suitable location of the blockchain cluster, and the applying of the computing power, QPSO enhances the protection, and the throughput of the blockchain systems [6].
Challenges in Implementing QIEAs
- Computational Complexity:
Thus, QIEAs are potentially optimal but, like all other identified quantum algorithms, it can be computational-loaded due to quantum physics’ uniqueness [3]. Balancing computational expense with security advantages is a critical problem for large-scale blockchain networks [4].
- Quantum-specific knowledge:
Implementing QIEAs involves specialist understanding of quantum computing and evolutionary algorithms, which can be an obstacle for many businesses [6]. Proper training and competence are critical for a successful deployment.
- Integration with existing systems:
Integrating QIEAs on current blockchain infrastructure can be difficult [2]. Ensuring compatibility and smooth functioning is critical for getting the most benefits.
Emerging trends and future directions
- Hybrid Quantum–Classical Approaches:
Combining quantum-inspired algorithms with conventional approaches can improve their efficiency and help solve computing problems [6]. Hybrid techniques combine the qualities of both paradigms to produce effective security solutions.
- Quantum Machine Learning:
Combining quantum-inspired evolutionary techniques with machine learning can enhance danger detection and response capabilities [3]. Quantum machine learning models have the potential to surpass traditional methods for identifying and reducing security risks.
Conclusion
There are the assumption that the further evolution of such defenses may be connected with the use of the techniques based on the quantum-inspired evolutionary algorithms [3]. These are algorithms based on the concepts of quantum computability that relate to flexibility, efficiency, and in terms of durability related to the management of security problems. It has primarily the appealing characteristic of no one would wish to reverse any specific transaction once it can be entered in the specific blockchain. Among various industries, the application of blockchain technology has been examined and implemented more in the financial sector especially concerning banking and cryptocurrencies [7]. While considerable implementation issues exist, the potential advantages make QIEAs an important tool for protecting blockchain networks [6]. Future research and development in hybrid techniques and quantum machine learning are expected to improve their efficiency [3].
References
- “Bitcoin: A Peer-to-Peer Electronic Cash System.” Accessed: Jul. 12, 2024. [Online]. Available: https://bitcoin.org/en/bitcoin-paper
- D. J. Bernstein and T. Lange, “Post-quantum cryptography,” Nature, vol. 549, no. 7671, pp. 188–194, Sep. 2017, doi: 10.1038/nature23461.
- K.-H. Han and J.-H. Kim, “Quantum-inspired evolutionary algorithm for a class of combinatorial optimization,” IEEE Trans. Evol. Comput., vol. 6, no. 6, pp. 580–593, Dec. 2002, doi: 10.1109/TEVC.2002.804320.
- A. Narayanan and M. Moore, “Quantum-inspired genetic algorithms,” in Proceedings of IEEE International Conference on Evolutionary Computation, May 1996, pp. 61–66. doi: 10.1109/ICEC.1996.542334.
- A. M. Widodo et al., “Quantum-Resistant Cryptography,” in Innovations in Modern Cryptography, IGI Global, 2024, pp. 100–130. doi: 10.4018/979-8-3693-5330-1.ch005.
- J. Sun, B. Feng, and W. Xu, “Particle swarm optimization with particles having quantum behavior,” in Proceedings of the 2004 Congress on Evolutionary Computation (IEEE Cat. No.04TH8753), Jun. 2004, pp. 325-331 Vol.1. doi: 10.1109/CEC.2004.1330875.
- M. Rahaman, F. Tabassum, V. Arya, and R. Bansal, “Secure and sustainable food processing supply chain framework based on Hyperledger Fabric technology,” Cyber Secur. Appl., vol. 2, p. 100045, Jan. 2024, doi: 10.1016/j.csa.2024.100045.
- Vajrobol, V., Gupta, B. B., & Gaurav, A. (2024). Mutual information based logistic regression for phishing URL detection. Cyber Security and Applications, 2, 100044.
- Gupta, B. B., Gaurav, A., Panigrahi, P. K., & Arya, V. (2023). Analysis of cutting-edge technologies for enterprise information system and management. Enterprise Information Systems, 17(11), 2197406.
- Gupta, B. B., Gaurav, A., & Panigrahi, P. K. (2023). Analysis of retail sector research evolution and trends during COVID-19. Technological Forecasting and Social Change, 194, 122671.
Cite As
Katiyar A. (2024) Enhancing Blockchain Security with Quantum-Inspired Evolutionary Algorithms, Insights2Techinfo, pp.1