By: Jampula Navaneeth1
1Vel Tech University, Chennai, India
2International Center for AI and Cyber Security Research and Innovations, Asia University, Taiwan
Email: navaneethjampula@gmail.com
Abstract
Even though now we are living in the digital world, e-mail appears to be one of the most frequently used means of communication, however using it has certain dangers concerning security. As more and more such attacks as phishing, spamming, and malware exist, it is even more necessary to increase the level of anti-email security. This has made deep learning algorithms to stand out as a useful tool in eliminating these threats since it provides sophisticated methods on how to handle risks. But where the vision of transformation and great developments in e-mail security is concerned, in this article we will be looking at how Deep Learning Algorithms will improve e-mail security.
Introduction
In these days spam-email is one of the most expensive and quickly developing issues related to internet. In technical terms, spam emails are primarily a commercial one and holds the allure to the most trafficked site and but mostly, they go to the home page [1]. The DL is one of the sub-sectors of the machine learning industry therefore it has not matured yet. As input samples, they are in a long row which might assist him in learning what feature to choose. The DL has two main attributes which make it entirely unique from the other methods. Firstly, they can well learn the hierarchical as well as the complex feature representation. Second, it can store the previous registered huge sequence of inputs. Recently deep learning attributes to the improvement of precision of various fields including images, language, voice, and so on [2].
Role of Deep Learning in Email Security
Known as a subfield of ML, DL tries to imitate the functionality of the human brain’s neurons to process and analyse large chunks of information. When it comes to the protection of the emails deep learning algorithms can be used to recognize and pattern associated with malicious activities [1]. These algorithms can filter out the unwanted and reject, the wanted and desirable by using data from the past to compute the results.
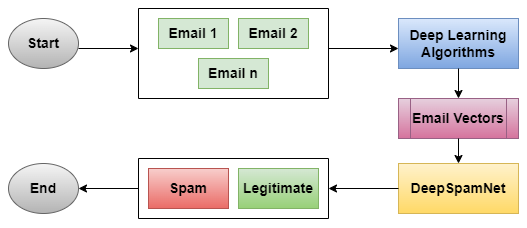
Email Security Threats
E-mail is therefore often under attack since it is common both in deployment and use as a mechanism for interacting with external and potentially untrustworthy organizations. Hackers may use e-mails to get control over an organization and subsequently compromise its Information Technology [3]. Some legal rights are the right to access to resources, and the right to access confidential information. The following are the common threats to email system:
- Malware
Slowly but surely, with e-mails and portable media devices one builds up many different forms of attack on the corporation through the use of malware that consists of virus, spyware, worm and a Trojan horse. It is also applied in post-production where application of deep learning techniques is inevitable. In other words, after the use of the training phase of the malware with the available signalling data, the problem solving of the assessment of the analysis tool/detection system can be done on the new real time data [4].
- Spam and Phishing
The consumer is harassed by Spam messages that reduce productivity, take up much of the IT bandwidth, and are used most frequently in delivering malware. Phishing is related to spam and it represent the fake computer-based methods to entice he particular person into responding to the e-mail and providing personal details. Spam messages are relayed through compromised e-mail hosts with an additional email address [4].
- Social Engineering
Instead of penetrating an organization’s system, e-mail can be employed to elicit information from consumers of an organization or gain users for performing functions. Considering the principles of social engineering, e-mail spoofing is a typical instance of the given kind of attack. There is successful impersonation of one user or program by the other in the context of concealing the sender’s identity of the e-mail [4].
Discussion
About the cyber realm, it is stated that copious data from numerous sources can be submitted to deep learning. However, a study in this area is bedevilled by poor availability of datasets which are either small, stale or developed within the organization and not in the public domain [5]. The benchmark datasets should be big, and should be updated frequently because stream is a big flow to enhance the cyber security solutions that can help to establish the true confidence in the deep learning algorithms [4].
Conclusion
The rise of cyber threats means that it is high time to strengthen the protection of the primary means of business communication. Advanced methods of risk prevention linked to phishing, spam, and malware apply deep learning algorithms that can adapt to this type of threats. To this end, with the help of deep learning, organizations are able to improve the security of their emails and preserve the confidentiality of the messages exchanged.
Thus, deep learning is viewed as the future of email security, which will finally provide a scalable and growing solution to one of the most critical problems of cybersecurity.
References
- K. V. Samarthrao and V. M. Rohokale, “Enhancement of email spam detection using improved deep learning algorithms for cyber security,” JCS, vol. 30, no. 2, pp. 231–264, Mar. 2022, doi: 10.3233/JCS-200111.
- S. Srinivasan, V. Ravi, M. Alazab, S. Ketha, A. M. Al-Zoubi, and S. Kotti Padannayil, “Spam Emails Detection Based on Distributed Word Embedding with Deep Learning,” in Machine Intelligence and Big Data Analytics for Cybersecurity Applications, Y. Maleh, M. Shojafar, M. Alazab, and Y. Baddi, Eds., Cham: Springer International Publishing, 2021, pp. 161–189. doi: 10.1007/978-3-030-57024-8_7.
- “Foundations of Phishing Detection Using Deep Learning: A Review of Current Techniques.” Accessed: Aug. 11, 2024. [Online]. Available: https://insights2techinfo.com/foundations-of-phishing-detection-using-deep-learning-a-review-of-current-techniques/
- M. M. Saeed and Z. A. Aghbari, “Survey on Deep Learning Approaches for Detection of Email Security Threat,” CMC, vol. 77, no. 1, pp. 325–348, 2023, doi: 10.32604/cmc.2023.036894.
- M. Rahaman, S. S. Bakkireddygari, S. Chattopadhyay, A. L. Gomez, V. Arya, and S. Bansal, “Infrastructure and Network Security,” in Metaverse Security Paradigms, IGI Global, 2024, pp. 108–144. doi: 10.4018/979-8-3693-3824-7.ch005.
- M. Casillo, F. Colace, B. B. Gupta, A. Lorusso, F. Marongiu and D. Santaniello, “Blockchain and NFT: a novel approach to support BIM and Architectural Design,” 2022 International Conference on Innovation and Intelligence for Informatics, Computing, and Technologies (3ICT), Sakheer, Bahrain, 2022, pp. 616-620, doi: 10.1109/3ICT56508.2022.9990815.
- P. Chaudhary, B. B. Gupta, K. T. Chui and S. Yamaguchi, “Shielding Smart Home IoT Devices against Adverse Effects of XSS using AI model,” 2021 IEEE International Conference on Consumer Electronics (ICCE), Las Vegas, NV, USA, 2021, pp. 1-5, doi: 10.1109/ICCE50685.2021.9427591.
Cite As
Navaneeth J (2024) Enhancing Email Security with Deep Learning Algorithms, Insights2Techinfo. pp.1