By: KUKUTLA TEJONATH REDDY, International Center for AI and Cyber Security Research and Innovations (CCRI), Asia University, Taiwan, tejonath45@gmail.com
Abstract:
This article delves into the critical role of multilayer perceptron (MLPs) in phishing detection is an essential part of modern cybersecurity: phishing attacks featuring deceptive social engineering tactics continuously target individuals and organizations take risks. The article explores the features of MLPs, multi-layered artificial neural networks, and describes their success in identifying complex patterns of phishing attempts Key characteristics including feature extraction, nonlinear decision boundaries, adaptability, anomaly detection, and increased accuracy Dynamic of phishing attacks -of nature Point out as formidable tools for mitigation The discussion also addresses important usability considerations by emphasizing the importance of high quality training data, hyperparameter tuning, and time emphasis on self-examination. By leveraging the capabilities of MLPs, organizations can strengthen their cybersecurity posture by enabling them to identify and respond to phishing threats in a timely manner, and thereby protecting sensitive information and preserving the integrity of digital ecosystems.
Introduction:
Phishing attacks continue to pose a serious threat to individuals and organisations, using social engineering techniques to deceive users and compromise sensitive information Artificial intelligence (AI) and machine learning (ML); play an important role in the ongoing fight against cyber threats. This article examines the use of multilayer perceptron (MLPs), a type of artificial neural network, in phishing detection [1][2].
Understanding multilevel perceptron:
Multilayer perceptron’s (MLPs) are artificial neurons composed of several connected layers or neurons. The architecture consists of an input layer, one or more layers of storage, and an output layer. MLPs excel at finding complex relationships in data, making them ideally suited for tasks such as pattern recognition and classification [2][3].
Key Features of MLP in Phishing Detection:
Feature extraction and pattern recognition:
MLPs are adept at extracting relevant elements from investment data, which is critical to uncovering patterns of phishing attempts. By training the network on different data sets with relevant phishing patterns, MLPs can learn to recognize subtle but important patterns in the data.
Nonlinear decision constraints:
Phishing attacks often involve complex and non-linear patterns that can be difficult to capture with traditional linear models. MLPs, with the ability to model nonlinear relationships, can create more flexible decision boundaries, allowing sophisticated phishing techniques to be detected.
Flexibility and Learning:
MLPs are able to adapt to new phishing trends. By continuously learning and updating data, these networks can improve their accuracy over time and stay ahead of dynamic phishing attacks.
Detection of Anomalies:
Phishing attempts often deviate from the norm, and MLPs excel at finding anomalies in the data. By identifying relevant connections and user interactions, MLPs can flag intercepts that could indicate a potential phishing attack.
Enhanced Accuracy and Reduced False Positives:
The multi-layered structure of MLPs enables the capture of complex relationships in the data, allowing greater accuracy in distinguishing between legitimate phishing activity. This helps to reduce, and provide, false positives highly reliable phishing detection system.
Implementation considerations:
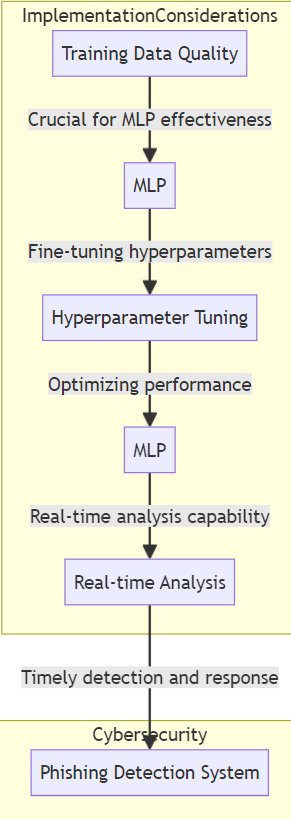
Quality training data:
The effectiveness of MLP in phishing detection is highly dependent on the quality and diversity of the training dataset. It is important to include multiple phishing scenarios to ensure the success of the model and to update the dataset regularly.
Hyperparameter settings:
Optimization of MLP hyperparameters, such as the number of hidden layers, neurons per layer, and learning rate, is important for optimal performance Testing and validation in different configurations can contribute to better results.
Real-time analysis:
Phishing attacks typically move quickly, requiring real-time analysis. Implementing MLP in a system that can detect and respond in a timely manner is critical to mitigating the impact of phishing incidents.
Conclusion:
Multilayer perceptron’s offer a powerful solution in the fight against phishing attacks, leveraging their ability to identify complex patterns and adapt to evolving threats. As technology advances, AI and the incorporation of sophisticated ML models are essential to improve cybersecurity strategies. By recognizing the capabilities of MLPs, organizations can strengthen their defences, proactively detect and prevent efforts to protect sensitive information and preserve the integrity of digital ecosystems.
References:
- Al-Ahmadi, S. (2020). PDMLP: phishing detection using multilayer perceptron. International Journal of Network Security & Its Applications (IJNSA) Vol, 12.
- Odeh, A. M. M. A. R., Alarbi, A. B. D. A. L. R. A. O. U. F., Keshta, I. S. M. A. I. L., & Abdelfattah, E. M. A. N. (2020). Efficient prediction of phishing websites using multilayer perceptron (MLP). Journal of Theoretical and Applied Information Technology, 98(16), 3353-3363.
- Alloghani, M., Al-Jumeily, D., Hussain, A., Mustafina, J., Baker, T., & Aljaaf, A. J. (2020). Implementation of machine learning and data mining to improve cybersecurity and limit vulnerabilities to cyber attacks. Nature-inspired computation in data mining and machine learning, 47-76.
- Chang, P. (2022). Multi-Layer Perceptron Neural Network for Improving Detection Performance of Malicious Phishing URLs Without Affecting Other Attack Types Classification. arXiv preprint arXiv:2203.00774.
- Kilincer, I. F., Ertam, F., Sengur, A., Tan, R. S., & Acharya, U. R. (2023). Automated detection of cybersecurity attacks in healthcare systems with recursive feature elimination and multilayer perceptron optimization. Biocybernetics and Biomedical Engineering, 43(1), 30-41.
- Ghillani, D. (2022). Deep learning and artificial intelligence framework to improve the cyber security. Authorea Preprints.
- Alsmirat, M. A., Jararweh, Y., Al-Ayyoub, M., Shehab, M. A., & Gupta, B. B. (2017). Accelerating compute intensive medical imaging segmentation algorithms using hybrid CPU-GPU implementations. Multimedia Tools and Applications, 76, 3537-3555.
- Tripathi, S., Gupta, B., Almomani, A., Mishra, A., & Veluru, S. (2013). Hadoop based defense solution to handle distributed denial of service (ddos) attacks.
- Almomani, A., Gupta, B. B., Wan, T. C., Altaher, A., & Manickam, S. (2013). Phishing dynamic evolving neural fuzzy framework for online detection zero-day phishing email. arXiv preprint arXiv:1302.0629.
- Gupta, B. B., Joshi, R. C., & Misra, M. (2012). ANN based scheme to predict number of zombies in a DDoS attack. Int. J. Netw. Secur., 14(2), 61-70. http://ijns.jalaxy.com.tw/contents/ijns-v14-n2/ijns-2012-v14-n2-p61-70.pdf
Cite As
REDDY K.T (2023) Enhancing Phishing Detection Through Multilayer Perceptron in Cybersecurity, Insights2Techinfo, pp.1