By: Indu Eswar Shivani Nayuni, Department of Computer Science & Engineering (Data Science), Student of Computer Science & Engineering, Madanapalle Institute of Technology and Science, Angallu(517325), Andhra Pradesh.
Abstract
This is more so in the present day business environment where competition is cut throat and information is processed within the shortest time possible, it becomes extremely important to predict revenues to the best of one’s abilities in a dignified manner that promotes management and sustenance of the competitive advantage of a firm. Despite the fact that there are a number of traditional measures for calculation of the forecast, which are sufficiently useful, still they give insufficient coverage to what is expected of the modern, and, in every respect, more liberal markets. Therefore, to focus on the topic, this article will solely elucidate how the generative AI will revolutionize the way to forecast the business’ revenue in advance. Their ability to handle big and various datasets enable generative artificial intelligence to produce accurate and timely forecasts, which is accompanied by differentiation of concerned complex patterns and relations not given by traditional method. From this paper, it is established that the application of AI in revenue prediction enables data to be handled with ease, the accuracy of the predictions to be improved, and the solution made more uniform and hence improves business decisions. Some of the implementation challenges listed out are as follows are describing the need of specialization coupled with not insignificant costs of the initial stages of SFA, but it is offset by the advantages of efficiency and superior decision making offered by SFA. Based on the perspective provided in the given article, certain crucial points can be regarded as the following: Criminology of generative AI points to the latter as one of the most effective tools to predict a plethora of issues to the necessary degree as per the industry’s demands; The required economy needs a manager that is knowledgeable of and efficient in the field of forecasting, and such a manager is generative AI; The required economy’s need for constant and sustainable growth is possible with generative AI.
Keywords: Generative AI, Advanced analytics, Predictive analytics, Revenue forecasting, Business intelligence, Accurate predictions, Efficiency in forecasting, Modern business environment, Technology in finance
Introduction
With an increase in the business complexity and interaction with various types of risks and competitors, forecasting of revenues is important in most companies for the purpose of prediction[1]. After that, it turns into an essential issue for many of the traditional methodologies of forecasting which is used to dealing with aggregative information and simple mathematical apparatuses; employing all of this experience to solve the new conditions of the Mercado and its rapidly shifting characteristics. One factor limiting the use of these methods is that when analyzing large amount of data to identify the pattern of the data or the differentiation between the customers and or the market, these methods are not very useful. Of course, these methods cannot be employed when working with big data, and when searching for activity and complexity in search of diverse patterns and shifts when it comes to the consumer behavior patterns and changes, as well as the conditions of the market.
Meet you generative AI – a brilliant instrument, which helps the organizations and uses the ML and algorithm to expand the possible ranges of revenues. In contrast with the pre-discussed methods, generative AI can incorporate a sales data, customers’ data, social media accounts, and macro-economic data to generate not only more expansive but also more accurate future revenue estimates.
The future will always be in generative AI because such AI can look for entangled patterns in data hence general AI will give better predictions. Similarly, it has the real-time update ability which help organizations to prevent the undecided market change, conduct preventive operations on business strategies. The value of this capability is proved even in the currently still relatively rather unstable economic environment where the aspect of timeliness and speed of the produced insights is of major importance.
This paper also examines how and why generative AI helps in improving the forecast of revenue, the enablement of data processing, and the quality of the prediction possible with the help of generative AI; and the issues and options brought by the use of generative AI. With comprehending the strengths and various aspects in the application of generative AI in forecasting, organisations will have the ability to reveal greater horizon of tactical functioning and new structure for constant progression and stability in the modernised composite world economy[2].
Comparison: Traditional Forecasting Methods vs. Generative AI vs Actual method
The following fig 1 explain about the revenue trends using generative AI
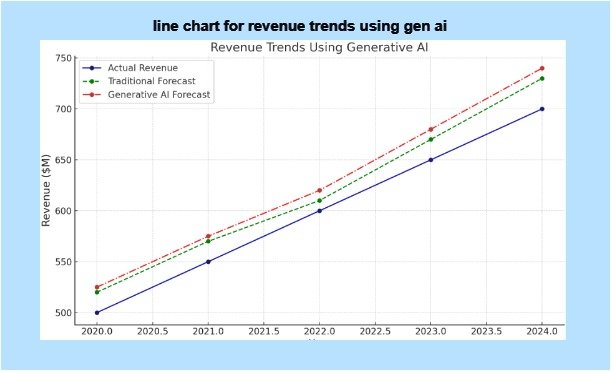
Fig 1: Line chart for the trends of the revenue using Gen ai
Dimension | Traditional Methods | Generative AI |
---|---|---|
Data Sources | Imitates habits by employing previous sales experience, simple cost indexes of the production and sometimes a limited amount of information from beyond the particular company[3]. | Uses information components that can be as diverse and substantial as company’s sales records, clients’ demographics, social media metrics, and national economic trends. |
Pattern Recognition | Appropriate for single patterns and trends which contain only the seasonality factor, but not appropriate for the complicated arch and combination of the datasets. | More effective than the other methods in recording and differentiating patterns and relations in compressed large data sets containing deeper information. |
Scalability | Coordinating large amounts of data is always costly, always time consuming, and always computationally expensive, most of the time[4]. | It is more effective on handling and analyzing data hence incredibly flexible in accommodating huge chunks of data. |
Predictive Accuracy | May be used sometimes to hint the stability of the process but fail in high-volatile markets because of their reliance on basic models. | It gives higher accuracy when compared to linear models of the data mainly due to incorporating the couplings in the data set. |
Adaptability | Less suitable for changing markets and customers’ behavior and, for this reason, less accurate in predicting state changes. | Relies on new data and on the fluctuations in the structure of the market and constantly updates the figures. Letting businesses quickly and easily adapt to the situations that occur in the market. |
Limitations | General, analytic and not as strong in estimating non-linearity and interaction between the factors being tested and used so lower predictability. | . It can be used to fake a number of scenarios and so provide the client with a plethora of options concerning potential outcomes for further business planning. |
Ease of Use | Typically changeable, and in most cases excludes data in which one variable affects another variable in a cyclical manner that would reduce the accuracy of the model. | Such possibilities that enables many scenarios to be applied such as the ability to imitate, allows to gain a broad outlook on the available opportunities based on which can contribute to the strategic management of companies and organizations in a particular area of activity. |
Expertise Required | Easy to apply with conventional statistical instruments and packages: They are based on fundamental statistics and thus, the results can be relied upon. | More hidden may be the methods related to the use of machine learning and AI where it may need some consultation with specialists in the field of the subject knowledge. |
Integration | Less interferences with other business practices and other business information systems compared to other business methods. | Technical integration may at times be rather complicated but is less complex when achieved than API integration. |
Automation | Little automation, that was most processes need to be updated and adjusting manually, it takes more time and plenty of space for human error. | Removes interference thus increasing efficiency and no longer has to be rigid in the schedule. |
Real-Time Forecasting | Often contain static views, which should be updated quite often, that sometimes can be a time-consuming process and, moreover, some of them do not reflect the current state of the market. | It uses real-time information and hence its updates are real time which is very useful in, decision making. |
Initial Costs | They typically take less in start-up capital and capital that needs to be invested hence can be run by comparatively small firms or firms with low capital. | Hardware expense It requires use of capital to acquire the AI gadgets, and staff training concerning the use of the identified systems. |
Ongoing Costs | Lower maintenance costs compared to the other types of databases; however, it may take less time to be updated to contain up-to-date information . | Realization of more effort in order to sustain the model given that, in this instance, the model is actively observed and adjusted. |
Resource Allocation | Less rigorous in their computational requirements, and as such, ideal for implementation especially for businesses with limited resources in their IT department. | High computational complexity particularly in terms of the processing of large data sets and training of models. Organizations might have to pay for the progressive infrastructure of Information Technology. |
Analysis
In the case of employing the generative AI for business revenues forecast it is observed that the specified model follows a different paradigm, however, the features of the model is far superior in terms of ownership, accuracy, flexibility and real-time.[5] The focus in this paper is the extent to which generative AI holds concern in relation to the improvement of the forecasting of revenues and impact on commerce. [4]
Data Handling and Integration
Data Sources: In the last section of the paper, there are different assortments of data: sales data, customer data, results of the social networking, and macroeconomic data that contribute to the summary of the research.
Pattern Recognition: Compared to the other analytical techniques, it stands out as more productive in practices of looking for rather subtle relations between several variables in as many and vast datasets for revealing the other types of connection which are not distinguished by the studying tools.
Scalability: With respect to this, it can treat inputs and provide the result properly therefore it is more flexible and can provide results on time concerning when it has been instructed to do so[4].
Predictive Accuracy and Adaptability
Predictive Accuracy: Nevertheless it is thus more fitting in the sense that ut transforms these trends of the data and ‘solidifies’ them in order for them to be manipulated according to complicated forms of non-linearity. It is going that there are precise values, whereby greater validity relates to other data series, by which the forecasts concerning the different forms of data are impacted.
Adaptability: Is in a position to perform an operation on newly entered data and get to the expected market state in the shortest time possible. Nevertheless, assists in the proper placement of such businesses so that they can be in a better position to handle matters concerning change within the market.
Sophistication: Is able to develop different action scenarios which exist in business and gives a clue on the result that is expected to be attained. It empowers business persons so that they can take the right decisions when making them and they always ensure that the most likely situation is adequate to address it is provided for.[6]
Implementation and Usability
Ease of Use: An integration of AI into a system may sometimes be lip due to skills in machine learning and AI existing in the system. The first one involves the identification of the right model, the next is training of the model followed by the implanting of the model in the organisation’s system[7].
Expertise Required: Concerning the data scientific analytics self-confidence, AI modeling approach, and the quantum of info-communication technology. AI may need to recruit or maybe directly employ some of the staff that will be useful in managing as well as updating the systems at a sometime basis.
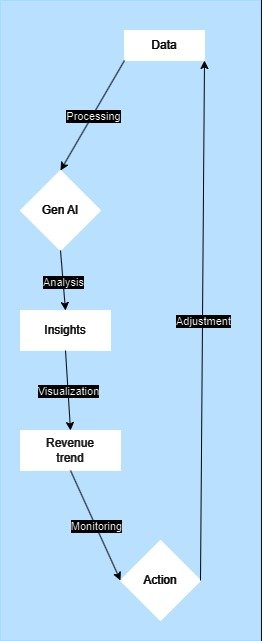
Fig 2: implementation of prediction of revenue trends
.
Implementation and Usability
Ease of Use: Implementing AI can be complex, requiring specialized knowledge in machine learning and AI. The setup process involves selecting appropriate models, training them, and integrating them into existing systems[8].
Expertise Required: Involves expertise in data science, AI modeling, and computational resources. Businesses may need to invest in training or hire specialized personnel to manage and maintain AI systems[8].
Integration: One weakness of SFA is that; It can be interfaced with other existing systems –as compared to when it has prospective abilities after integration. This becomes decisive, particularly in those subdomains that are concerned with the steady stream of data to and from the AI system and the familiarization of AI with the local structures.
Conclusion
Classic approaches utilized earlier in the field of revenue forecasting can be substituted by the generative AI’s entirely new and effective avenue, as mentioned above with its contribution to significant improvements in data implementation, precision, structure, and enriched immediate accessibility. While there are increased costs in relation to putting the system in and the work is more encompassing in a manner, the benefits of a system in the long run are worth the costs and are arguably more accurate, quicker, and yield more diagnostics.
Therefore, combining generative AI for businesses willing to stay relevant and forecast correctly is a perfect tool to improve the creep enhancement of the aforementioned aspects. Clearly stating, eliminating problems like integration, bias, and scalability, it is possible to unleash the AI potential that can help organizations achieve better accuracy when it comes to overall business performance and improving the organization’s performance to ensure higher profitability and growth.
In conclusion, it is possible to assert that generative AI is not just an evolution of the fine-tuning of traditional methods of forecasting but is an entirely new generation means of shaping the future of organizations’ revenue breakthrough. Corporations can gain competitive advantage through the adoption of AI’s superior features in operations as complex service sophistication increases in business operations thus making revenues and profits better.
Reference
- T. Haksoro, A. S. Aisjah, Sreerakuvandana, M. Rahaman, and T. R. Biyanto, “Enhancing Techno Economic Efficiency of FTC Distillation Using Cloud-Based Stochastic Algorithm,” Int. J. Cloud Appl. Comput. IJCAC, vol. 13, no. 1, pp. 1–16, Jan. 2023, doi: 10.4018/IJCAC.332408.
- M. Rahaman, F. Tabassum, V. Arya, and R. Bansal, “Secure and sustainable food processing supply chain framework based on Hyperledger Fabric technology,” Cyber Secur. Appl., vol. 2, p. 100045, Jan. 2024, doi: 10.1016/j.csa.2024.100045.
- “How artificial intelligence will change the future of marketing | Journal of the Academy of Marketing Science.” Accessed: Aug. 08, 2024. [Online]. Available: https://link.springer.com/article/10.1007/S11747-019-00696-0
- P. Pappachan, Sreerakuvandana, and M. Rahaman, “Conceptualising the Role of Intellectual Property and Ethical Behaviour in Artificial Intelligence,” in Handbook of Research on AI and ML for Intelligent Machines and Systems, IGI Global, 2024, pp. 1–26. doi: 10.4018/978-1-6684-9999-3.ch001.
- R. A. Abumalloh, M. Nilashi, K. B. Ooi, G. W. H. Tan, and H. K. Chan, “Impact of generative artificial intelligence models on the performance of citizen data scientists in retail firms,” Comput. Ind., vol. 161, p. 104128, Oct. 2024, doi: 10.1016/j.compind.2024.104128.
- A. M. Widodo et al., “Port-to-Port Expedition Security Monitoring System Based on a Geographic Information System,” Int. J. Digit. Strategy Gov. Bus. Transform. IJDSGBT, vol. 13, no. 1, pp. 1–20, Jan. 2024, doi: 10.4018/IJDSGBT.335897.
- M. Moslehpour, A. Khoirul, and P.-K. Lin, “What do Indonesian Facebook Advertisers Want? The Impact of E-Service Quality on E-Loyalty,” in 2018 15th International Conference on Service Systems and Service Management (ICSSSM), Jul. 2018, pp. 1–6. doi: 10.1109/ICSSSM.2018.8465074.
- J. P. Richardson et al., “Patient apprehensions about the use of artificial intelligence in healthcare,” Npj Digit. Med., vol. 4, no. 1, pp. 1–6, Sep. 2021, doi: 10.1038/s41746-021-00509-1.
- Chui, K. T. (2023, November). A Lightweight Generative Adversarial Network for Imbalanced Malware Image Classification. In Proceedings of the 5th International Conference on Information Management & Machine Intelligence (pp. 1-4).
- Gaurav, A., Gupta, B. B., Arya, V., Chui, K. T., & Peñalvo, F. J. G. (2023, October). Adaptive Defense Mechanisms Against Phishing Threats in 6G Wireless Environments. In 2023 IEEE 98th Vehicular Technology Conference (VTC2023-Fall) (pp. 1-5). IEEE.
Cite As
Nayuni I.E.S. (2024) Geni ai in predicting revenue trends, Insights2Techinfo, pp.1