By: Dhanush Reddy Chinthaparthy Reddy, Department of Computer Science and Artificial Intelligence, Madanapalle Institute of Technology and Science, Angallu(517325), Andhra Pradesh
Abstract
In the modern world, networks encompass almost every aspect of people’s lives, thus they became critical objects for cyber threats. In this respect, more recent approaches to the identified threats are insufficiently good, as, on the one hand, cyber threats are getting more diverse and more extensive with time; on the other hand, traditional security measures are rather inadequate in terms of defending against such sophisticated attacks. Network Security is one of the areas that have benefited significantly from Artificial Intelligence (AI) with solutions that are dynamic and up to date than the traditional approaches.
This paper aims at investigating how AI can strengthen network protection against all forms of cyber security threats such as malware, phishing, DDoS and insider threats. AI systems use sophisticated ML algorithms that allow them to analyse large amount of data from network traffic searching for patterns that could signal security threats. The use of Artificial Intelligence based Intrusion Detection Systems and automated counter measures allow organizations to effectively counter threats with an incredible speed and accuracy thus preventing much of the possible harm. This paper sheds light on success stories on how AI has been applied in network security showing how organizations employ AI in guarding important infrastructure and data. However, the paper that is also presented does touch on the issues of concern that are related to AI adoption including but not limited to algorithmic bias, privacy, and over-dependence on automated systems. In the future as AI keeps on developing it becomes central to play a larger function as a key component of cybersecurity templates from trends which show that there is a stronger integration of AI to predictive analysis and threat hunting.
The paper concludes by discussing the development of the AI in network security in the future, highlighting the need for a proper integration of the new technologies into the security framework that will be both effective and ethical at the same time. Over the development of application of AI in the context of network security, there is the potential for the reshaping of ways in which we secure info-space and the stability of networks in an progressively interconnected global community.
Keywords: Artificial Intelligence, Protection, Networks.
Introduction
Indeed, networks are essential to the functioning of the modern world, supporting people’s connections and businesses’ operations as well as servicing a multitude of industries. As will be shown from financial institutions to healthcare providers and governments to multinational companies, the dependency on networked systems is now universal. But here as well, with our increasing reliance on these networks, so also lies the potential for harm. Hacktivism has grown in intensity and potency and seeks to breach network security to acquire and disseminate data and interrupt services, all in efforts to cause monetary and image loss.
Firewalls, anti-virus software systems, or traditional systems where vulnerability was matched against a known threat were previously enough to meet network defense. Nevertheless, there is an urgent problem here because the threats within cyberspace are constantly altering, and the methods listed above are becoming less effective because they are conventional. This post now features new-age techniques where hackers use techniques like zero-day targets, polymorphic viruses, and social engineering to overcome ordinary barriers and cause a lot of damage. The vast number of network connections and the nature of contemporary networks make this job much more challenging, along with the constant flow of threats.
To tackle these challenges Artificial Intelligence (AI) has risen to the challenge in the field of network security. Due to its capabilities to learn from data, to find patterns, and to take decisions by its own, AI can be considered as a valuable and adaptive instrument to protect the networks against current and emerging threats. Unlike the conventional security that works on scripts and masks that are constantly updated, these systems learn with new data, and therefore prevent threats as they arise.
Anomaly Detection
Another major part of AI involved in network security is the anomaly detection and is defined as the ability to find the points of deviation from the normal behaviour of the network[1]. Anomaly detection is different from conventional security systems that use set of rules and signatures to identify known threats in that it uses Advanced Machine Learning to define normal patterns of network traffic. They consist in an identification of new activity in the nets that can occur because of a nonparametric flow in the traffic, more frequent accesses, or preferences for different hours of the day. Another major benefit of the anomaly detection is the capability to detect such threats which were unknown and sometimes impossible to discover with the help of other methods, for example, zero-day attack. [2]AI systems change over time and can rapidly differentiate between normal network changes and real threats, and against possible threats, almost eliminating false alarms and improving the protection of the network. In other words, anomaly detection gives an organization a way of being ready and responding to new threats that are out there in the market, hence reducing effecting the impacts of these threats.
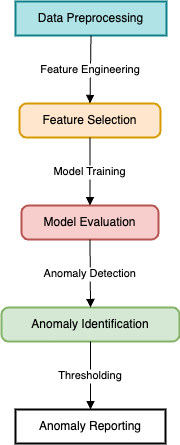
Privacy Concerns
There are tremendous advantages in appreciating the possibilities of using AI in network security; however, it will not be implausible to note that this would attract overwhelming privacy issues. AI systems might need large amounts of data for the networks to be monitored and threats to be identified. This data can also encompass what the user is doing, what they are saying, and even who they are, in form of personal details. This kind of data, when collected, stored and used by AI systems may cause violation of privacy if not well handled. In front of these AI controlled security measures be potentially violating the privacy of the users to the extent that they collect more information than required or as used with malicious intent. Also, applying AI in network security can lead to problems with the accountability and the transparency because the processes giving the output by the AI algorithms are normally complex and very hard to explain[3, p. 2], [4]. This makes the use of AI a ‘black box’ and difficult to ascertain whether or not the data being fed into the system is being used in an ethically sound or compliant with the privacy regulations. To mitigate these risks, data minimization, encryption, and compliance audits must be enacted while policies should adhere to security and the privacy of the individual’s rights. This means that as AI further remains an active constituent of the network security field, conversations and thoughtful and strategic consideration of the privacy aspects will remain key in the right use of these technologies.
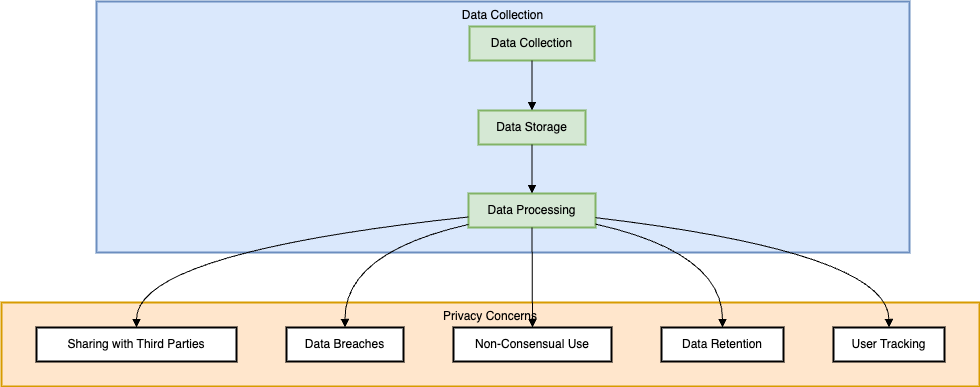
Bias in Algorithms
Social bias is an issue of worry for AI models, especially for applications to network security. AI systems learn from data and therefore, the kind of data fed to such systems can affect performance of the system. Bias can be built into the training dataset in terms of historical basis, random or deliberate, sampling, or skewed data representation and the AI model might replicate such bias in its decision making. In network security, this could occur in any of the ways in which the system relies on biased data in raising alarms, for instance erroneously categorizing certain kinds of network traffic as malicious or the extreme monitoring of certain groups of users as compared to others. For instance, if AI is trained on data which may not include some of the behaviours that prevail in the networks or some demographic groups, threats within the missing context may not be detected, and they will be treat threats as more threatening than they really are[3].
Whenever bias seeps into algorithms that AI uses, the ripple effects can be severe, reducing even the best security to a mockery and offering injustice. For instance, it is possibly to develop prejudiced AI models which may cause specific user accounts or devices being attacked while at the same time ignore real threats in other domains. This does not only make the network insecure but also makes some ethical and legal issues involved such as the aspect of anti-discrimination policies.
To reduce risks of unbiased AI algorithms, it is necessary to apply measures that will prevent bias and contribute to creation of an inclusive AI technology. This means that control of training data is born of making a point to avoid biased, old, and unrepresentative data. [5]Ensuring that bias is taken out annually or periodically in systems being used in the network also, requires the use of corrective measures to rectify the systems, for this reason, the following is important in preserving the efficacy; Corrective measures to rectify the system for bias annually/periodically Duties and responsibilities for corrective measures for bias in systems used in the network Rubber stamping of bias by an independent professional of the bias found in professional systems The Moreover, providing people with the function that would allow them to oversee AI decision-making processes and increasing transparency of AI action can contribute to the AI effectiveness and prevent inequitable treatment of people. As the use of AI further expands in security for our networks there will be a strong need to deal with issues of bias in algorithms to ensure the optimum fairness for all users.
Conclusion
AI is now an essential aspect in the fight against cyber threats that target networks as the threats evolve to become more and more complicated. Furthermore, AI takes the concept to a whole new level through use and implementation of machine learning, anomaly detection, and automated response system to provide a dynamic solution that would not otherwise be available in the security measures. It is in this regard that this paper has discussed on how AI fortifies network security which includes; threat detection and response in Realtime and generally boosting the capability of network assets. Based on extraordinary examples, we are witnessing changes in the use of AI to protect infrastructure and sensitive information from cyber threats.
But the integration of AI in network security has its problems that are worthy of consideration. Areas of concern include bias in the Artificial Intelligence algorithms, privacy, and the dangers of dependency on AI systems. These are some of the challenges that need to be meet halfway that is to enhance the benefits of AI and at the same time the best course of action ensure that AI is ethical in its security deliverables. AI in networks security is still developing and as it advances, network security utilizing the AI technologies is expected to enhance with AI future technological growth in threat identification and the predictive analytics.
All in all, AI delivers promising information to protect network security against current and future threats. But to achieve this, there will always be the need for innovations, ethics and voluntary disclosure. Based on these principles, organizations can utilize the AI technologies to secure their networks and at the same time respect the fair, private and accountable principles. Thus, summarizing the trend for the further development of the synergy of AI and human intelligence as the basis for the protection of the digital environment and stable CGI.
References
- Y. Himeur, K. Ghanem, A. Alsalemi, F. Bensaali, and A. Amira, “Artificial intelligence based anomaly detection of energy consumption in buildings: A review, current trends and new perspectives,” Appl. Energy, vol. 287, p. 116601, Apr. 2021, doi: 10.1016/j.apenergy.2021.116601.
- K. T. Putra, A. Z. Arrayyan, R. Z. Syahputra, Y. A. Pamungkas, and M. Rahaman, “Design a Two-Axis Sensorless Solar Tracker Based on Real Time Clock Using MicroPython,” Emerg. Inf. Sci. Technol., vol. 4, no. 1, Art. no. 1, May 2023, doi: 10.18196/eist.v4i1.18697.
- “Managing Bias in AI | Companion Proceedings of The 2019 World Wide Web Conference.” Accessed: Aug. 14, 2024. [Online]. Available: https://dl.acm.org/doi/abs/10.1145/3308560.3317590
- J. Carmody, S. Shringarpure, and G. Van de Venter, “AI and privacy concerns: a smart meter case study,” J. Inf. Commun. Ethics Soc., vol. 19, no. 4, pp. 492–505, Jan. 2021, doi: 10.1108/JICES-04-2021-0042.
- B. D. Alfia, A. Asroni, S. Riyadi, and M. Rahaman, “Development of Desktop-Based Employee Payroll: A Case Study on PT. Bio Pilar Utama,” Emerg. Inf. Sci. Technol., vol. 4, no. 2, Art. no. 2, Dec. 2023, doi: 10.18196/eist.v4i2.20732.
- Gupta, B. B., Gaurav, A., & Panigrahi, P. K. (2023). Analysis of retail sector research evolution and trends during COVID-19. Technological Forecasting and Social Change, 194, 122671.
- Aldweesh, A., Alauthman, M., Al Khaldy, M., Ishtaiwi, A., Al-Qerem, A., Almoman, A., & Gupta, B. B. (2023). The meta-fusion: A cloud-integrated study on blockchain technology enabling secure and efficient virtual worlds. International Journal of Cloud Applications and Computing (IJCAC), 13(1), 1-24.
Cite As
Reddy D.R.C. (2024) How Artificial Intelligence Protects Our Networks, Insights2Techinfo, pp1.