By: Jampula Navaneeth1
1Vel Tech University, Chennai, India
2International Center for AI and Cyber Security Research and Innovations, Asia University, Taiwan Email: navaneethjampula@gmail.com
Abstract
The threats are emerging at a significantly rapidly at a breakneck speed and traditional technologies are incapable of managing them in an adequate way. One of the subsets of machine learning, deep learning or artificial neural networks is slowly becoming a effective tool in fighting cyber threats. Different from conventional methods, where potential threats have to be defined by signatures and rules, deep learning has the capacities of learning from the raw feed what features are typical of novelties threats. However, there are some disadvantages that should be discussed now for instance, higher demand on computational power and the explanation of such models. With threats getting more sophisticated, deep learning is expected to play a very crucial role when it comes to improvement of security panoramic.
Keywords: Deep Learning, Cyber Threats, Security
Introduction
Security has been a crucial aspect especially in this modern world as people find themselves and companies more and more exposed to cyber threats. The world that is dominated by digital technologies has become more dangerous. Cyber threats and cyber-crime such as theft and loss of data, ransomware, frauds such as phishing and more recently, denial-of-service attacks have increased in recent years. Such attacks question the reliability of digital systems and the infrastructure, meaning that they entail not only monetary losses but other impacts as well [1]. This article will expand on the uses as well as the adversities that surround the incorporation of deep learning in cybersecurity.
Deep Learning Architectures for Cyber Threat Detection
Deep learning architectures, particularly CNNs and RNNs, have revolutionized cyber threat detection by enabling the automated analysis of diverse data types, including images, network traffic logs, and system logs [2]. Leveraging the hierarchical representation learning paradigm, these architectures automatically extract relevant features from raw data, facilitating accurate and efficient detection of cyber threats [1].
Key applications of threat detection
- Anomaly Detection: Here, deep learning is trained on the events of real user behaviour and identifies that which is beyond the normal standard and could be a sign of a breech or malicious activity. Anomaly detection is to find cases that are quite different from other cases, and this is a critical problem with numerous applications, including fraud detection, intrusion detection, and video surveillance [3].
- Malware Detection: In the fight against cyber threats, malware detection stands as a primary application of deep learning. By analysing characteristics and behaviour, deep learning models are trained to recognize and classify various malware types [1].
- Phishing Prevention: By using NLP deep learning can read through emails and websites to establish features that are peculiar of phishing attempts and protect the PC against such tricks in real-time [1].
- Network Traffic Analysis: It can also analyse and interpret different forms of traffic in a network, and quickly flag anomalies and possible intrusion.
- Behavioural Analysis: Deep learning techniques are utilized for behavioural analysis, where they analyse user behaviour and system activities to detect anomalies and potential security threats [1].
Performance Evaluation Metrics
There are performance metrics that researchers use when they compare and analyse deep learning models for use in cyber threat detection and response. These metrics offer important information on the performance of the model and it is reliability and robustness in different situations and on other datasets [1], [4].
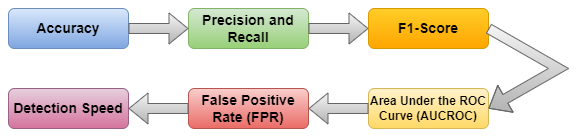
Challenges
The essential question of building more robust deep learning systems is not how well these models can learn from data but how they can be protected from adversarial input. Strengthening the assurance of deep learning solutions in the real-world security applications is always a ongoing work. Academic and industrial communities must therefore collar in efforts to improve the security of such systems, counter to the continuing emergence of increasingly inventive attack vectors [1].
Conclusion
It is now beyond doubt that deep learning is reshaping cyberspace threat analysis and providing the necessary protection for valuable assets. While cyber attackers are evolving so in their tool, hence incorporating the adaptive and perceptive features of deep learning will be an essential practice in the future.
References
- A. Joseph, “Deep Learning for Cyber Threat Detection,” vol. 04, no. 01.
- P. Pappachan, N. S. Adi, G. Firmansyah, and M. Rahaman, “Deep Learning-Based Forensics and Anti-Forensics,” in Digital Forensics and Cyber Crime Investigation, CRC Press, 2024.
- S. Yuan and X. Wu, “Deep learning for insider threat detection: Review, challenges and opportunities,” Computers & Security, vol. 104, p. 102221, May 2021, doi: 10.1016/j.cose.2021.102221.
- M. Rahaman, B. Chappu, N. Anwar, and P. K. Hadi, “Analysis of Attacks on Private Cloud Computing Services that Implicate Denial of Services (DoS),” vol. 4, 2022.
- Raj, B., Gupta, B. B., Yamaguchi, S., & Gill, S. S. (Eds.). (2023). AI for big data-based engineering applications from security perspectives. CRC Press.
- Gupta, G. P., Tripathi, R., Gupta, B. B., & Chui, K. T. (Eds.). (2023). Big data analytics in fog-enabled IoT networks: Towards a privacy and security perspective. CRC Press.
- Chaudhary, P., Gupta, B. B., & Singh, A. K. (2022). XSS Armor: Constructing XSS defensive framework for preserving big data privacy in internet-of-things (IoT) networks. Journal of Circuits, Systems and Computers, 31(13), 2250222.
- Sedik, A., Maleh, Y., El Banby, G. M., Khalaf, A. A., Abd El-Samie, F. E., Gupta, B. B., … & Abd El-Latif, A. A. (2022). AI-enabled digital forgery analysis and crucial interactions monitoring in smart communities. Technological Forecasting and Social Change, 177, 121555.
Cite As
Navaneeth J. (2024) How Deep Learning is Changing the Landscape of Cyber Threat Detection, Insights2Techinfo, pp.1