By : Varsh Arya, Asia University Taiwan
In today’s interconnected digital world, the frequency and sophistication of cyber threats continue to rise. Distributed Denial of Service (DDoS) attacks, in particular, pose significant challenges for organizations of all sizes. These attacks can disrupt online services, causing severe financial and reputational damage. As the threat landscape evolves, so does the need for advanced defense mechanisms. In this blog post, we will explore how Artificial Intelligence (AI) and Machine Learning (ML) are reshaping the fight against DDoS attacks, empowering organizations to navigate this challenging threat landscape.
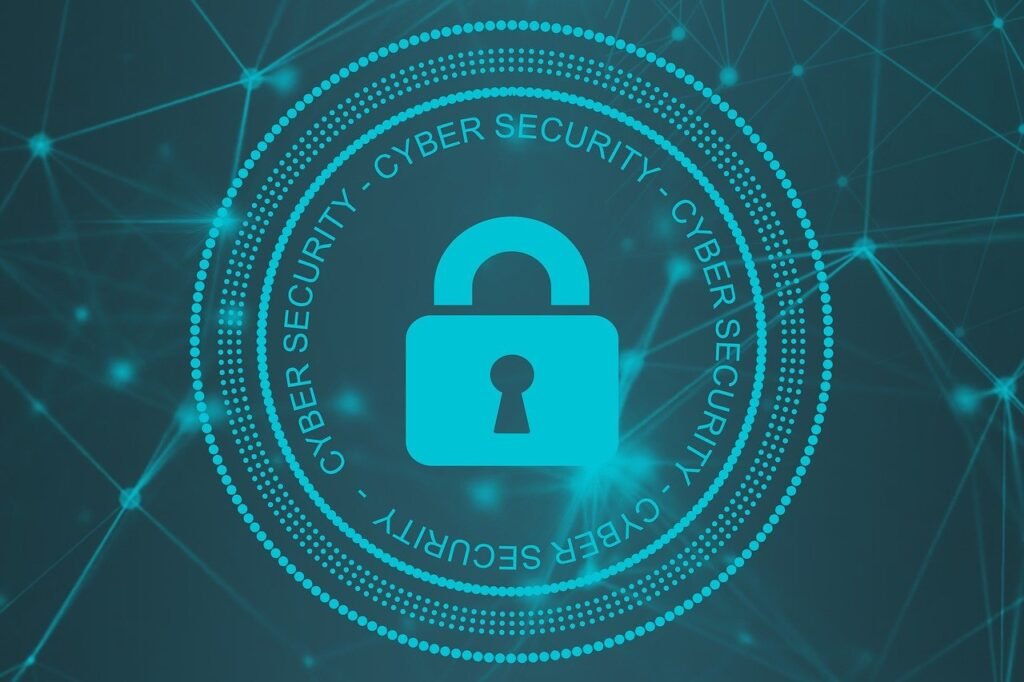
Understanding DDoS Attacks
Before diving into the role of AI and ML, let’s quickly review what DDoS attacks entail. A DDoS attack occurs when a network or website is overwhelmed with an enormous volume of malicious traffic, rendering it inaccessible to legitimate users. Attackers achieve this by either mobilizing a botnet, a network of compromised computers, or by exploiting vulnerable systems to amplify the attack’s impact. Traditional defense mechanisms struggle to handle these attacks due to their scale and complexity.
The Power of AI and ML in DDoS Defense
AI and ML technologies have emerged as game-changers in the battle against DDoS attacks. By harnessing their capabilities, organizations can augment their defense strategies, enabling proactive threat detection, effective mitigation, and faster response times. Here are some ways AI and ML contribute to navigating the DDoS threat landscape:
- Anomaly Detection: AI and ML algorithms can analyze network traffic patterns and identify anomalies that may indicate a potential DDoS attack. By learning from historical data, these systems can establish baselines and detect deviations, enabling early warning and proactive defense measures.
- Real-time Monitoring and Alerting: AI-powered monitoring systems continuously analyze network traffic in real-time. They can detect sudden spikes or unusual patterns that align with DDoS attack characteristics. When an attack is identified, alerts are generated, allowing swift response and mitigation actions.
- Intelligent Traffic Filtering: ML algorithms can analyze and classify incoming traffic in real-time, differentiating legitimate user requests from malicious traffic. By automatically filtering out malicious requests, organizations can ensure uninterrupted service availability for genuine users.
- Behavior-based Analysis: AI and ML models can learn the typical behavior of legitimate users, enabling them to identify abnormal user activities that may signify an ongoing DDoS attack. By monitoring user behavior and recognizing patterns associated with malicious intent, organizations can take immediate action to mitigate the threat.
- Adaptive Mitigation Strategies: ML algorithms can adapt and learn from previous attack patterns, improving the accuracy and efficiency of mitigation strategies over time. By continuously refining their defenses based on real-world data, organizations can stay one step ahead of evolving DDoS attack techniques.
Conclusion
In the era of AI and ML, organizations have powerful tools at their disposal to combat the growing threat of DDoS attacks. By leveraging the capabilities of these technologies, organizations can enhance their defense mechanisms, detect attacks in real-time, and respond swiftly to mitigate the impact. However, it is important to note that AI and ML are not standalone solutions but should be integrated into a comprehensive defense strategy that combines human expertise, network infrastructure enhancements, and continuous monitoring. By navigating the threat landscape with AI and ML as allies, organizations can bolster their resilience and ensure uninterrupted service availability in the face of DDoS attacks.
References
- Mirkovic, J., & Reiher, P. (2004). A taxonomy of DDoS attack and DDoS defense mechanisms. ACM SIGCOMM Computer Communication Review, 34(2), 39-53.
- Mirkovic, J., Prier, G., & Reiher, P. (2002, November). Attacking DDoS at the source. In 10th IEEE International Conference on Network Protocols, 2002. Proceedings. (pp. 312-321). IEEE.
- Gupta, B. B., Joshi, R. C., & Misra, M. (2012). ANN based scheme to predict number of zombies in a DDoS attack. Int. J. Netw. Secur., 14(2), 61-70.
- Osanaiye, O., Choo, K. K. R., & Dlodlo, M. (2016). Distributed denial of service (DDoS) resilience in cloud: Review and conceptual cloud DDoS mitigation framework. Journal of Network and Computer Applications, 67, 147-165.
- Gupta, B. B., Gupta, S., Gangwar, S., Kumar, M., & Meena, P. K. (2015). Cross-site scripting (XSS) abuse and defense: exploitation on several testing bed environments and its defense. Journal of Information Privacy and Security, 11(2), 118-136.
- Douligeris, C., & Mitrokotsa, A. (2004). DDoS attacks and defense mechanisms: classification and state-of-the-art. Computer networks, 44(5), 643-666.
- Feinstein, L., Schnackenberg, D., Balupari, R., & Kindred, D. (2003, April). Statistical approaches to DDoS attack detection and response. In Proceedings DARPA information survivability conference and exposition (Vol. 1, pp. 303-314). IEEE.
- Alsmirat, M. A., et al. (2017). Accelerating compute intensive medical imaging segmentation algorithms using hybrid CPU-GPU implementations. Multimedia Tools and Applications, 76, 3537-3555.
- De Donno, M., Dragoni, N., Giaretta, A., & Spognardi, A. (2018). DDoS-capable IoT malwares: Comparative analysis and Mirai investigation. Security and Communication Networks, 2018, 1-30.
- Deveci, M., et al. (2022). Personal mobility in metaverse with autonomous vehicles using Q-rung orthopair fuzzy sets based OPA-RAFSI model. IEEE Transactions on Intelligent Transportation Systems.
- Lee, K., Kim, J., Kwon, K. H., Han, Y., & Kim, S. (2008). DDoS attack detection method using cluster analysis. Expert systems with applications, 34(3), 1659-1665.
- Sahoo, S. R., et al. (2019). Hybrid approach for detection of malicious profiles in twitter. Computers & Electrical Engineering, 76, 65-81.
- Bawany, N. Z., Shamsi, J. A., & Salah, K. (2017). DDoS attack detection and mitigation using SDN: methods, practices, and solutions. Arabian Journal for Science and Engineering, 42, 425-441.
- Dahiya, A., et al. (2021). A reputation score policy and Bayesian game theory based incentivized mechanism for DDoS attacks mitigation and cyber defense. Future Generation Computer Systems, 117, 193-204.
- Nazario, J. (2008). DDoS attack evolution. Network Security, 2008(7), 7-10.
- Bhatti, M. H., et al. (2019). Soft computing-based EEG classification by optimal feature selection and neural networks. IEEE Transactions on Industrial Informatics, 15(10), 5747-5754.
- Yuan, X., Li, C., & Li, X. (2017, May). DeepDefense: identifying DDoS attack via deep learning. In 2017 IEEE international conference on smart computing (SMARTCOMP) (pp. 1-8). IEEE.
- Bhushan, K., et al. (2017). Security challenges in cloud computing: state-of-art. International Journal of Big Data Intelligence, 4(2), 81-107.
Cite As
Arya V. (2023) Navigating the Threat Landscape DDoS Attacks in the Era of AI and ML, Insights2Techinfo, pp. 1