By: KUKUTLA TEJONATH REDDY, International Center for AI and Cyber Security Research and Innovations (CCRI), Asia University, Taiwan, tejonath45@gmail.com
Abstract:
In today’s cyber environment, phishing attacks pose a devastating threat, requiring sophisticated detection techniques to protect individuals and organizations from potential harm. This article explores how Support Vector Classification (SVC) takes off effective in terms of phishing detection. Leveraging its strengths in handling high-dimensional, nonlinear data and strong generalization, SVC emerges as a powerful tool to accurately distinguish between legitimate phishing instances. If the discussion requires basic features such as parameter tuning and data imbalances dealt with internally, and establish the appropriateness of SVC in light of the evolving nature of phishing threats e.g. As organizations seek to strengthen their cybersecurity defences, understanding and implementing SVC in phishing detection techniques is critical to maintaining the integrity of the digital ecosystem and encrypting information needs protection.
Introduction:
Phishing attacks have become increasingly sophisticated, posing significant risks to individuals and organizations. As cybercriminals use more sophisticated methods to defraud users, the need for stronger detection techniques has never been greater [1]. One such powerful tool in cybersecurity is the Support Vector Classifier (SVC), which is proving highly effective in detecting and mitigating phishing threats [2].
Understanding Phishing:
Phishing is a type of computer attack where attackers use fraudulent techniques to disclose sensitive information such as passwords, credit card information, and other personal data to individuals These attacks typically consist of fraudulent emails or legitimate identifiable web messages but such is designed to compromise security [3].
The Role of Support Vector Classifier:
Machine learning algorithm Support Vector Classifier has gained popularity in various industries due to its ability to handle complex data and provide accurate predictions and when used in phishing detection, it has proven to be a valuable asset by SVC by exercising pattern recognition and classification capabilities [4].
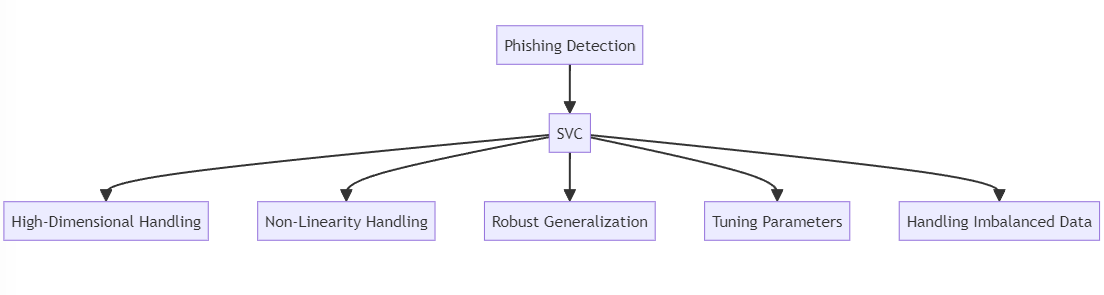
Figure :Role of Support Vector Classifier
Key Features of Support Vector Classifier in Phishing Detection:
High Dimensionality Handling:
Phishing detection data sets typically contain high quality features. SVC excels at processing such data types, as it can create a hyperplane that effectively separates data into different groups, and distinguishes between legitimate and phishing patterns.
Non-Linearity Handling:
Phishing attacks typically involve complex patterns and non-linear relationships in the data. Especially if you are using nonlinear kernels, SVC can capture this complex relationship very well, increasing the ability to detect subtle phishing signals.
Robust Generalization:
SVC exhibits strong generalization capability, which makes it more efficient on unseen data. This is important in the context of phishing detection, where new and evolving attack strategies require models that can be highly adaptable and comprehensive.
Tuning Parameters for Optimal Performance:
SVC provides various parameters that can be fine-tuned to achieve optimal performance. Proper parameter tuning ensures that the classifier is well-suited to the specific characteristics of phishing datasets, enhancing its accuracy and reliability.
Handling Imbalanced Data:
Phishing datasets often suffer from class imbalance, where the number of valid instances far exceeds the number of phishing instances. SVC is able to handle data imbalances by assigning appropriate weights to classes, ensuring that the sample is not biased towards the majority class.
Conclusion:
In the ever-evolving cybersecurity landscape, the use of advanced machine learning algorithms such as support vector classification is crucial for effective phishing detection SVC’s ability to handle high-quality, non-linear data and its robust normalization handling make it a powerful tool in combating phishing attacks In systems Integration can enhance their ability to identify and mitigate phishing threats, and ultimately create information that it is necessary to protect and maintain the integrity of digital ecosystems.
References:
- Torabi, Z. S., Nadimi-Shahraki, M. H., & Nabiollahi, A. (2015). Efficient support vector machines for spam detection: a survey. International Journal of Computer Science and Information Security, 13(1), 11.
- Anupam, S., & Kar, A. K. (2021). Phishing website detection using support vector machines and nature-inspired optimization algorithms. Telecommunication Systems, 76(1), 17-32.
- Kim, H. C., Pang, S., Je, H. M., Kim, D., & Bang, S. Y. (2003). Constructing support vector machine ensemble. Pattern recognition, 36(12), 2757-2767.
- Khamis, S. A., Foozy, C. F. M., Aziz, M. F. A., & Rahim, N. (2020). Header based email spam detection framework using Support Vector Machine (SVM) Technique. In Recent Advances on Soft Computing and Data Mining: Proceedings of the Fourth International Conference on Soft Computing and Data Mining (SCDM 2020), Melaka, Malaysia, January 22– 23, 2020 (pp. 57-65). Springer International Publishing.
- Dheepa, V., & Dhanapal, R. (2012). Behavior based credit card fraud detection using support vector machines. ICTACT Journal on Soft computing, 2(4), 391-397.
- Christmann, A., & Steinwart, I. (2008). Support vector machines.
- Li, Y., Yang, L., & Ding, J. (2016). A minimum enclosing ball-based support vector machine approach for detection of phishing websites. Optik, 127(1), 345-351.
- Wang, L., Li, L., Li, J., Li, J., Gupta, B. B., & Liu, X. (2018). Compressive sensing of medical images with confidentially homomorphic aggregations. IEEE Internet of Things Journal, 6(2), 1402-1409.
- Stergiou, C. L., Psannis, K. E., & Gupta, B. B. (2021). InFeMo: flexible big data management through a federated cloud system. ACM Transactions on Internet Technology (TOIT), 22(2), 1-22.
- Gupta, B. B., Perez, G. M., Agrawal, D. P., & Gupta, D. (2020). Handbook of computer networks and cyber security. Springer, 10, 978-3.
- Bhushan, K., & Gupta, B. B. (2017). Security challenges in cloud computing: state-of-art. International Journal of Big Data Intelligence, 4(2), 81-107.
Cite As
REDDY K.T (2023) Unveiling the Power of Support Vector Classification in Phishing Detection, Insights2Techinfo, pp.1