By: Aryan Rawat, email: mco22383@ccet.ac.in
Department of CSE, Chandigarh College of Engineering & Technology, Chandigarh, India.
ABSTRACT-
Get rid of the nightmare of traffic snarl-ups and visualize a cityscape where the traffic lights compose a silent symphony, boxing-in the air-tight waves of vehicles. See the emergence of the lush green places thriving with the aid of the data-driven insights. This is no longer the realm of science fiction; this is the real possibility of machine learning defining the urban landscape of the future. Although the technological progress exercises a remarkable influence, the art is the framework of the things which lie outside the charm of alluring gadgets. It dives into the vast sea of many opportunities, from predicting floods to the optimizing the use of resources and above all recognizing the crucial role of the ethics and the need for equity. Seatbelts on, for the future of our cities accelerates towards us with a growing momentum – let us embark on this journey with responsibility and also inclusivity as our compass.
1. INTRODUCTION-
Sustainable urban development [1] is more important than ever as our world gets more and more advanced. The difficult task of striking a balance between social justice, economic growth, and environmental preservation falls to urban planners and legislators. To overcome these obstacles and build environmentally sustainable cities, the incorporation of data analytics [2] and machine learning into urban planning procedures has become increasingly important in recent years.
The digital sensor proliferation, IoT devices along with the large amounts of data availability trigger new opportunities to use information for better understanding of urban systems and delivery of services [3, 4]. This allows cities to formulate, gain useful information from large volumes of data that is produced through sensors, social media, and municipal databases. These ML driven applications have the capacity to change old cities into dynamic and self-governing network that optimizes utilization of assets, develop benefit conveyance just as better quality of life for occupants. A resolution to these issues is vital to unleash the full potential of ML for smart cities and create sustainable urban networks which can satisfy evolving resident requirements.
Usage of data analytics[5] and machine learning in urban planning is imperative for sustainability since the population shift from rural areas towards peri-urban, suburbs as well as other dense city centers hosting major international corporations continues to increase. Introduction of digital sensors, Internet of Things devices with internet connectivity are now offering a rich source data and information which could essentially be applied in cities to optimize their systems and services.
The use of machine learning can aid change conventional city systems into intelligent networks that make better utilization of resources, increase the quality and provision of services improving therefore life for inhabitants. Additionally, predictive analytics helps cities predict and mitigate environmental risks, contributing to resilient and adaptive urban infrastructure.
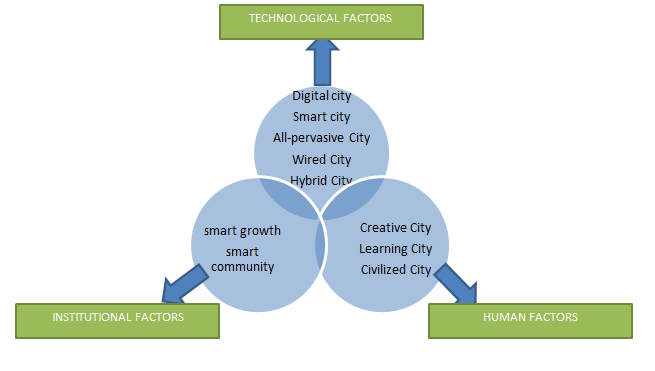
FIGURE 1- Three pillars of smart cities
2. Defining Smart cities:-
Use of smart computational compute to enable the designs and service delivery for critical infrastructure within a city – i.e., City healthcare, public safety
Because of the miraculous blend between technology and traditional urban planning, we now have an eye-catching concept called ‘smart cities’ [6].Gone are the days of the traditional approach. Connected technologies like grids, waste management systems, and air quality sensors are becoming integrated into the fabric of urban planning. This integration goes beyond mere efficiency, resulting in environmental sustainability [7] and improving quality of life.
Using data analysis and machine learning as planning tools is more than just a technical optimization. It’s a transformative change. This is an effective measure to the complex problems developed by rapid urbanization.
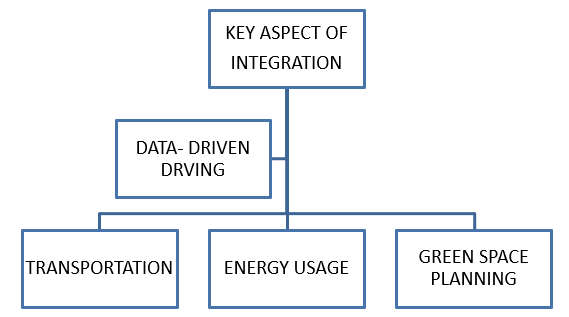
3. Key Aspects of Integration:-
3.1 Data-driven decision making –
Discover how real data and machine learning algorithms can improve your decision-making process. Examples of successful implementation and impact on city government.
3.2 Transportation System Optimization –
Discover how to use machine learning to optimize traffic flow and reduce congestion. Case studies demonstrate improvements in transport efficiency and environmental impact.
3.3 Energy efficiency [8] and resource management –
Discussion of intelligent energy networks and the use of machine learning for resource optimization. Presentation of successful resource management strategies in sustainable cities.
3.4 Predicting Events-
Floods: 4,444 deep learning models analyze historical data and map flood areas to enable proactive flood protection measures. Earthquakes: Recurrent neural networks (RNNs) can learn patterns of seismic activity and trigger early warning systems to potentially save lives. Extreme Weather: Complex algorithms process weather data and historical trends to predict hurricanes, tornadoes, and other extreme events, helping communities prepare for the worst.
3.5 Mitigating Impact-
Resource optimization: ML algorithms analyze disaster data to identify areas in need of emergency assistance and ensure efficient deployment of medical supplies and other critical resources. Infrastructure assessment: autonomous drones equipped with ML-powered image recognition can quickly scan disaster-affected areas, identify damaged buildings and roads, and direct repair efforts and prioritize critical infrastructure.
3.6 Scenario Simulation [9]-
ML models simulate the potential impacts of different disaster scenarios to help authorities plan evacuation routes, design resilient infrastructure, and develop effective response protocols. Helps you develop.
3.7 Green Space Planning –
Scope of how machine learning aids in the planning and optimization of urban green spaces. Examples of cities incorporating green infrastructure for enhanced livability.
4. Challenges and Ethical Considerations: –
Security threats exist as cities rely on ML-powered systems.
Strong cyber security measures [10], regular vulnerability assessments, and incident response plans are essential to mitigating risks such as traffic light hacks that cause traffic jams or energy grid breaches that plunge cities into darkness. Algorithmic bias [11], where biases in historical data are amplified, can lead to discriminatory results. To combat this, diverse datasets, regular fairness audits, and community participation in algorithm development are essential. Fair implementation is paramount. Transparency, inclusion, and digital literacy programs ensure that communities understand and benefit from ML[12-17].
By prioritizing marginalized communities, we can close the digital divide and ensure everyone thrives in the smart city revolution. Addressing these challenges can pave the way for a future where algorithms and urban planning work in harmony to create fair and prosperous cities for all.
Conclusion:
In the quest for sustainable cities, the combination of urban planning and data analysis with machine learning will be a beacon of innovation. By accessing the power of real-time data and predictive modeling, communities can optimize resources, improve quality of life, and reduce environmental impact.
As we approach this new era of urban development, it is important to approach these technologies with a focus on transparency, and ethical considerations.
REFERENCES:-
- Beatley, T., & Wheeler, S. M. (Eds.). (2004). The sustainable urban development reader. London, UK: Routledge.
- Wickham, H., & Wickham, H. (2016). Data analysis (pp. 189-201). Springer International Publishing.
- B. Pourghebleh and N. J. Navimipour, “Data aggregation mechanisms in the Internet of things: A systematic review of the literature and recommendations for future research,” Journal of Network
- M. Mohseni, F. Amirghafouri, and B. Pourghebleh, “CEDAR: A cluster-based energy-aware data aggregation routing protocol in the internet of things using capuchin search algorithm and fuzzy logic,” Peer-to-Peer Networking and Applications, pp. 1-21, 2022.
- H. Kosarirad, M. Ghasempour Nejati, A. Saffari, M. Khishe, and M. Mohammadi, “Feature Selection and Training Multilayer Perceptron Neural Networks Using Grasshopper Optimization Algorithm for Design Optimal Classifier of Big Data Sonar,” Journal of Sensors, vol. 2022, 2022.
- Chopra, M., Kumar, S., Madan, U., & Sharma, S. (2021, December). Influence and establishment of smart transport in smart cities. In International Conference on Smart Systems and Advanced Computing (Syscom-2021).
- Goodland, R. (1995). The concept of environmental sustainability. Annual review of ecology and systematics, 26(1), 1-24.
- Abir, S. A. A., Anwar, A., Choi, J., & Kayes, A. S. M. (2021). Iot-enabled smart energy grid: Applications and challenges. IEEE access, 9, 50961-50981.
- Jamshidian, F., & Zhu, Y. (1996). Scenario simulation: Theory and methodology. Finance and stochastics, 1, 43-67.
- Zhang, Y., Liu, M., Guo, J., Wang, Z., Wang, Y., Liang, T., & Singh, S. K. (2022, December). Optimal Revenue Analysis of the Stubborn Mining Based on Markov Decision Process. In International Conference on Machine Learning for Cyber Security (pp. 299-308). Cham: Springer Nature Switzerland.
- Akter, S., Dwivedi, Y. K., Sajib, S., Biswas, K., Bandara, R. J., & Michael, K. (2022). Algorithmic bias in machine learning-based marketing models. Journal of Business Research, 144, 201-216.
- Malik, M., Prabha, C., Soni, P., Arya, V., Alhalabi, W. A., Gupta, B. B., … & Almomani, A. (2023). Machine Learning-Based Automatic Litter Detection and Classification Using Neural Networks in Smart Cities. International Journal on Semantic Web and Information Systems (IJSWIS), 19(1), 1-20.
- Verma, V., Benjwal, A., Chhabra, A., Singh, S. K., Kumar, S., Gupta, B. B., … & Chui, K. T. (2023). A novel hybrid model integrating MFCC and acoustic parameters for voice disorder detection. Scientific Reports, 13(1), 22719.
- Chui, K. T., Gupta, B. B., Liu, J., Arya, V., Nedjah, N., Almomani, A., & Chaurasia, P. (2023). A survey of internet of things and cyber-physical systems: standards, algorithms, applications, security, challenges, and future directions. Information, 14(7), 388.
- Sharma, P. C., Mahmood, M. R., Raja, H., Yadav, N. S., Gupta, B. B., & Arya, V. (2023). Secure authentication and privacy-preserving blockchain for industrial internet of things. Computers and Electrical Engineering, 108, 108703.
- Upadhyay, U., Kumar, A., Sharma, G., Gupta, B. B., Alhalabi, W. A., Arya, V., & Chui, K. T. (2023). Cyberbullying in the metaverse: A prescriptive perception on global information systems for user protection. Journal of Global Information Management (JGIM), 31(1), 1-25.
- Alhalabi, W., Gaurav, A., Arya, V., Zamzami, I. F., & Aboalela, R. A. (2023). Machine learning-based distributed denial of services (DDoS) attack detection in intelligent information systems. International Journal on Semantic Web and Information Systems (IJSWIS), 19(1), 1-17.
Cite As
Rawat A (2024) Urban Planning and Data Analytics- Creating Sustainable Cities with Machine Learning, Insights2Techinfo, pp.1