By: Vanna karthik; Vel Tech University, Chennai, India
Abstract
Smishing is a form of phishing through SMS that has grown into a serious cyber threat, which brings financial and data losses. Traditional detection mechanisms are unable to detect the scam messages in an advanced nature; hence, this requires the deployment of sophisticated Artificial Intelligence and security tools. The project intends to explore the possibilities of AI-driven methods in smishing attack detection through natural language processing and machine learning models. Additionally, it assesses security tools designed to improve message filtering and threat detection. Findings indicate that incorporating AI-based models into security solutions greatly enhances smishing attempt detection and mitigation, offering a strong protection against online threats.
Introduction
Smishing, or phishing via SMS, has become a widely used tool by cybercriminals with the rapid adoption of mobile communication. These messages try to trick users into divulging sensitive information like banking credentials and personal data. Traditional spam filters are unable to distinguish between good and malicious messages due to constantly changing tactics employed in smishing[1]. AI and security tools promise to analyze message patterns, linguistic features, and contextual indicators to provide a better detection capability. The paper discusses the role of AI in detecting smishing messages and integrating security tools to strengthen the defense against such cyber threats.
The Growing Threat of Smishing
Most of the smishing attacks are made to appear as if they were coming from a valid organization, including banks, government agencies, or service providers, in order to trick individuals into clicking malicious links or revealing their personal information[2]. Because these messages are personalized and context-aware, they mostly evade traditional filtering. The aftermath of being victimized by smishing varies from mere financial loss to identity theft; thus, the need for enhanced means of detection.
Leveraging AI for Smishing Detection
Notably, machine learning and natural language processing methods driven by artificial intelligence have been very helpful in the identification of smishing efforts. The machine learning algorithms look for fraud by analyzing trends in the huge amount of SMS texts. In order for the AI to learn how to distinguish between harmful and legitimate messages using certain language, contextual, and behavioral markers, these models are trained using labeled datasets[3].
Natural Language Processing is helpful in making sense of the structure of scam messages. These NLP techniques analyze the tone, syntax, and semantic content of SMS messages to enable the AI systems to identify deviations from normal presentations indicative of fraudulent intent. Meanwhile, deep learning algorithms further improve detection because they continuously learn from the evolving smishing tactics to keep pace with the emerging threats[4].
Security Tools and Their Role in Smishing Prevention
Beyond artificial intelligence (AI), smishing protection is aided by security solutions including multi-factor authentication (MFA), real-time message screening, and mobile security apps[5]. Before they reach users, these solutions filter out questionable messages using AI-powered detection methods. To stop consumers from visiting malicious websites, some sophisticated security programs use URL analysis to confirm the authenticity of links included in SMS messages.
Network-level security measures have also been put in place by cybersecurity and telecommunications providers to detect and stop smishing attacks. These techniques can identify and stop major smishing attacks before they affect potential victims by examining metadata, sender reputation, and SMS traffic patterns.
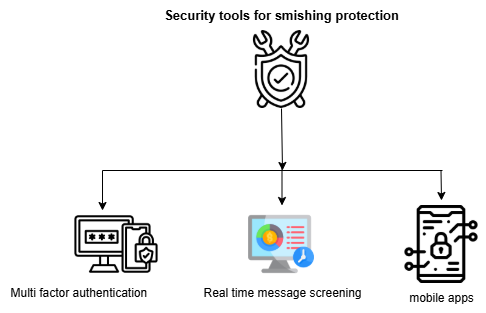
The Future of Smishing Detection
Continuous improvements in AI and security systems will be crucial for staying ahead of smishing threats as fraudsters hone their strategies. Future advancements could include improved encryption methods to protect mobile communications, real-time user education tools that issue alarms and warnings, and AI-driven chatbots that react automatically to suspicious smishing messages.
Despite the promising solutions provided by AI and security tools, smishing prevention still heavily relies on human knowledge. People should stay alert, refrain from clicking on unwanted links, and confirm the legitimacy of messages before acting. Users and businesses may effectively combat smishing threats and improve overall cybersecurity by combining technology developments with well-informed decision-making.
Conclusion
Smishing detection has advanced thanks in large part to AI and security tools. The suggested framework improves the capacity to differentiate between authentic and fraudulent messages by utilizing machine learning and natural language processing approaches. Real-time detection and reaction to smishing threats is ensured by integrating AI models into security systems. Future studies should concentrate on lowering false positive rates and enhancing model responsiveness to new smishing strategies. All things considered, AI-powered solutions present a viable way to stop smishing and protect users from changing online dangers.
References
- S. Mishra and D. Soni, “DSmishSMS-A System to Detect Smishing SMS,” Neural Comput. Appl., vol. 35, no. 7, pp. 4975–4992, Mar. 2023, doi: 10.1007/s00521-021-06305-y.
- K. Gowri and S. Brindha, “ADVANCEMENTS IN SMISHING DETECTION: A NOVEL APPROACH UTILIZING GRAPH NEURAL NETWORKS,” vol. 24, no. 1000, 2024.
- M. R. A. Saidat, S. Y. Yerima, and K. Shaalan, “Advancements of SMS Spam Detection: A Comprehensive Survey of NLP and ML Techniques,” Procedia Comput. Sci., vol. 244, pp. 248–259, 2024, doi: 10.1016/j.procs.2024.10.198.
- W. L. T. T. N. Kumarasiri, M. K. J. C. Siriwardhana, S. A. D. S. L. Suraweera, A. N. Senarathne, and S. M. B. Harshanath, “Cybersmish: A Proactive Approach for Smishing Detection and Prevention using Machine Learning,” in 2023 7th International Conference on I-SMAC (IoT in Social, Mobile, Analytics and Cloud) (I-SMAC), Kirtipur, Nepal: IEEE, Oct. 2023, pp. 210–217. doi: 10.1109/I-SMAC58438.2023.10290228.
- R. Chithambaramani, M. Sankar, J. Vimala Ithayan, M. D, P. Sivaprakash, and D. P. Mohan, “SMS Guard: Android Application to Prevent Smishing Attacks,” in 2024 Second International Conference on Intelligent Cyber Physical Systems and Internet of Things (ICoICI), Coimbatore, India: IEEE, Aug. 2024, pp. 69–74. doi: 10.1109/ICoICI62503.2024.10696674.
- Chui, K. T., Gupta, B. B., Alhalabi, W., & Alzahrani, F. S. (2022). An MRI scans-based Alzheimer’s disease detection via convolutional neural network and transfer learning. Diagnostics, 12(7), 1531.
- Gokasar, I., Pamucar, D., Deveci, M., Gupta, B. B., Martinez, L., & Castillo, O. (2023). Metaverse integration alternatives of connected autonomous vehicles with self-powered sensors using fuzzy decision making model. Information Sciences, 642, 119192.
- Gupta, B. B., Gaurav, A., Marín, E. C., & Alhalabi, W. (2022). Novel graph-based machine learning technique to secure smart vehicles in intelligent transportation systems. IEEE transactions on intelligent transportation systems, 24(8), 8483-8491.
- Katiyar K. (2024) Genetic Algorithms and Data Analytics for Cybersecurity in Phishing and Blockchain Systems, Insights2Techinfo, pp.1
Cite As
Karthik V. (2025) Advance Smishing Detection : Using AI and Security Tools to Identify Scam messages , Insights2techinfo pp.1