By: KUKUTLA TEJONATH REDDY, International Center for AI and Cyber Security Research and Innovations (CCRI), Asia University, Taiwan, tejonath45@gmail.com
Abstract:
Malicious URLS embedded in mail are among others important element that contributes towards cyber security as the rate of phishing attacks continues to rise today. Advanced approaches in phishing URL detection by incorporating advanced technology and methods.2]] This paper explores a wide array of defensive mechanisms, such as machine learning and artificial intelligence for behavioural profiling and natural language processing; real-time URL scanning and sandboxing. Moreover, the collaboration of threat intelligence feeds, user behaviour analytics, and email authentication protocols enhances defence line. Through understanding and adopting these advanced practices, organizations can greatly enhance their resilience towards email-based threats aimed at targeting both individual people and enterprises as a result.
Introduction:
Despite the digitisation age, email is still the major one for interaction in the world. However, it provides an opportunity for cyber-threats such as phishing attacks that are the largest and most destructive. Such phishing URLS embedded in emails are dangerous both for people and for companies. To counteract this rising danger, modern methods of detection of phishing URLs are developed applying up-to-date technologies and procedures aimed at improving email safety [1] [2].
Machine Learning and Artificial Intelligence:
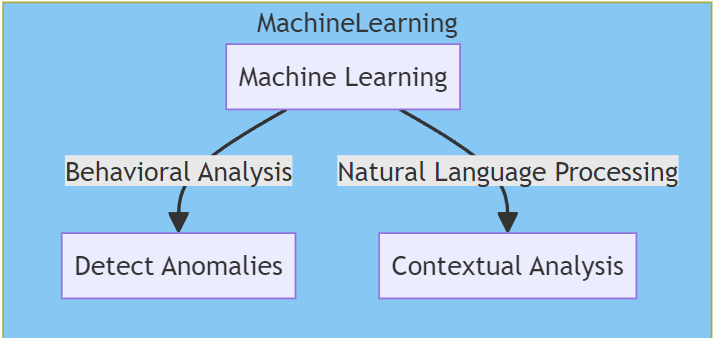
Behavioural Analysis: This means that the machine learning algorithms are able to assess the behaviour associated with URL patterns such as structure, content, and user interactions. The system is able to detect anomalies and deviations, which act as potential phishing warning signs in any event [3][2].
Natural Language Processing (NLP): The context of emails as well as their content is determined using NLP techniques. Through analysing the language structure and relating different parts together in a certain way, AI systems will find suspicious patterns that hint of cases of phishing.
Real-time URL analysis:
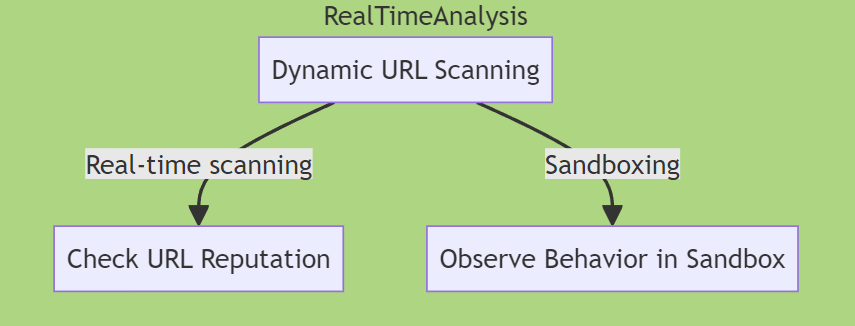
Dynamic URL Scanning: Advanced solutions use real-time URL scanning services that check the name of a link when clicked. This ensures that even if the URL appears innocuous at first, it can be blocked later if linked to phishing activities.
Sandboxing: Suspicious URLs can be opened in a controlled environment, called a sandbox, to monitor their actions without posing a risk to the actual user This enables security systems to detect malicious activity based on the sandboxed link of the URL in the 19th century.
Fishing Feed Combinations:
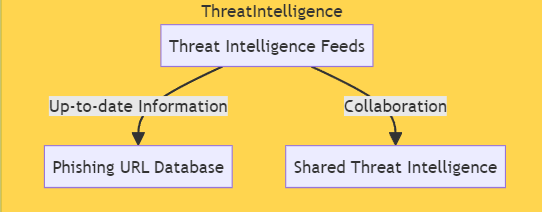
Threat intelligence feeds: When integrated with threat intelligence feeds, it provides up-to-date information about detected phishing URLs. This allows security systems to block or flag emails containing links to malicious websites listed in these feeds.
Collective threat intelligence: Shared threat intelligence across organizations enables mass defence against phishing attacks. Chat allows information about new phishing URLs to be spread quickly, improving the overall level of cybersecurity.
User Behaviour Analysis:
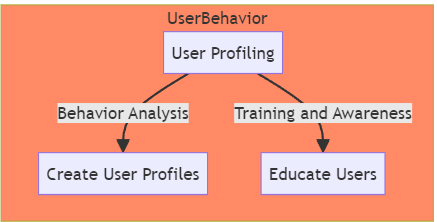
Figure 4:User profiling
User profiling: Monitoring and analysing the behaviour of individual users can help create user profiles. Deviating from established policies, such as clicking suspicious URLs, triggers additional alerts and security measures.
Training and awareness: Educating users about phishing threats and encouraging them to report suspicious emails can help protect them better. Machine learning models can also be trained based on user feedback to improve accuracy over time.
Email Authentication Protocols:
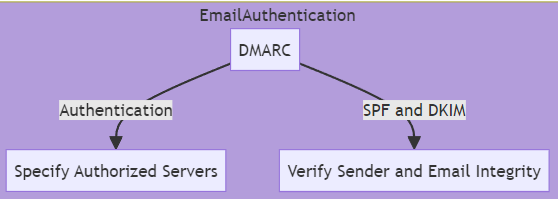
DMARC (Domain-based Message Authentication, Reporting, and Conformance): DMARC allows senders to specify which servers are allowed to send messages, which helps with email authentication This reduces the chances of phishing emails will appear as from trusted sources less [7].
SPF (Sender Policy Framework) and DKIM (DomainKeys Identified Mail): This protocol confirms the identity of the sender and the authenticity of the email content when used with DMARC.
Conclusion:
As phishing attacks become more complex, the importance of using advanced techniques to detect malicious URLs in emails becomes more apparent Through machine learning, real-time analytics, threat intelligence, the user by combining behavioural analytics, and robust email authentication mechanisms, organizations can establish robust processes. layered defences against these evolving threats have been demonstrated, significantly enhancing email security and reducing the potential harm from sophisticated phishing attempts.
Reference:
- Parekh, S., Parikh, D., Kotak, S., & Sankhe, S. (2018, April). A new method for detection of phishing websites: URL detection. In 2018 Second international conference on inventive communication and computational technologies (ICICCT) (pp. 949-952). IEEE.
- Nguyen, L. A. T., To, B. L., Nguyen, H. K., & Nguyen, M. H. (2013, October). Detecting phishing web sites: A heuristic URL-based approach. In 2013 International Conference on Advanced Technologies for Communications (ATC 2013) (pp. 597-602). IEEE.
- Ahammad, S. H., Kale, S. D., Upadhye, G. D., Pande, S. D., Babu, E. V., Dhumane, A. V., & Bahadur, M. D. K. J. (2022). Phishing URL detection using machine learning methods. Advances in Engineering Software, 173, 103288.
- James, J., Sandhya, L., & Thomas, C. (2013, December). Detection of phishing URLs using machine learning techniques. In 2013 international conference on control communication and computing (ICCC) (pp. 304-309). IEEE.
- Dutta, A. K. (2021). Detecting phishing websites using machine learning technique. PloS one, 16(10), e0258361.
- Kulkarni, A. D., & Brown III, L. L. (2019). Phishing websites detection using machine learning.
- Prakash, A., & Kumar, U. (2018). Authentication protocols and techniques: a survey. Int. J. Comput. Sci. Eng, 6(6), 1014-1020.
- Alsmirat, M. A., Jararweh, Y., Al-Ayyoub, M., Shehab, M. A., & Gupta, B. B. (2017). Accelerating compute intensive medical imaging segmentation algorithms using hybrid CPU-GPU implementations. Multimedia Tools and Applications, 76, 3537-3555.
- Tripathi, S., Gupta, B., Almomani, A., Mishra, A., & Veluru, S. (2013). Hadoop based defense solution to handle distributed denial of service (ddos) attacks.
- Almomani, A., Gupta, B. B., Wan, T. C., Altaher, A., & Manickam, S. (2013). Phishing dynamic evolving neural fuzzy framework for online detection zero-day phishing email. arXiv preprint arXiv:1302.0629.
- Gupta, B. B., Joshi, R. C., & Misra, M. (2012). ANN based scheme to predict number of zombies in a DDoS attack. Int. J. Netw. Secur., 14(2), 61-70.
Cite As
REDDY K. T. (2023) Advanced Techniques for Detecting Phishing URLs, Insights2Techinfo, pp.1