By: Poojitha Nagishetti, Department of Computer Science & Engineering (Data Science), Student of Computer Science & Engineering, Madanapalle Institute of Technology and Science, Angallu(517325), Andhra Pradesh.
Abstract –
Overweight is a chronic, multifactorial pathological condition, which has become global pandemic resulting in numerous adverse effects on the health of individuals, communities, and societies, as well as on social and economic development. The conventional methods of studying and putting strategies in place to combat obesity have always used the disease model which has many disadvantages such as not encompassing for the complex patterns of inheritance of genes and thus the regulation of weight. New technologies such as artificial intelligence and data science have created other ways of approaching obesity and offer better, precise, and efficient treatments. This article also focuses on the emerging field of AI and data science with respect to obesity and its prevention, identification, and control, the existing as well the prospects and attempting to demystify the scarcity.
Keywords –
Introduction –
Overweight is currently one of the major topics of concern in the modern world and in the sphere of public health. It is a disease which is expressed by the ability of an individual to accumulate body fat levels with special emphasis on the body mass index. It is noteworthy that BMI is one of the popular indicators, although it can hardly provide a sufficient picture of obesity, which is a multifactorial process depending on genetics, environment, metabolism, and behavior[1]. Obesity has more than doubled in the world and has risen to 650 million adults and 340 million children and adolescent who are obese. This increase is very crucial to human health since obesity is among the predictors of diseases such as type 2 diabetes, cardiovascular diseases, some forms of cancer, and musculoskeletal illnesses. Furthermore, obesity increases morbidity and mortality, and decreases the quality of life and costs of healthcare.
The causation of obesity is complex and has strong relationship with modem living habits which include low level energy expenditure, high energy intake and social economics[2]. They include dietary modification, exercise, and behavior therapy and have always been known to result in poor compliance especially in the management of obesity. It is a pity that those methods do not take into consideration the genetic factor, metabolism rate, and psychological backgrounds of people that result in obesity. Thus, the demand for tailored and efficient public health programs is more than pressing.
In the more recent past, healthcare brought into light the use of artificial intelligence and data science in creating novel approaches to the management of conditions such as obesity. Because of this, and because of the sheer volume of data that can be analyzed, AI has the capability of providing hitherto unknown patterns and trends. This capability is especially useful in the case of obesity since the disease is very multifactorial, portraying a genetic-environmental-behavioral interaction system.
In that regard, data mining that refers to the identification of patterns and knowledge from structured and unstructured databases expands AI in a way that the latter uses data and statistics such as EHRs, genetics, leader records, and social media activity. Therefore, AI and data science can be used as the methods that extend the knowledge about obesity, improve the estimation of the risks, and contribute to the development of the more effective treatment strategies.
So far, only little beginnings were made in the application of AI and data science in the context of obesity; the potential is tremendous. For instance, it is achievable to identify the individuals with obesity risk using the machine’s learning models before they develop this disease. Today, the source of the prescriptions that are unique to the data of an individual’s genetics and metabolisms can offer healthier as well as more efficient solutions to weight loss[3]. Besides, through the help of AI, accurate identification of the behavioral and psychological aspects that can lead to obesity can be achieved and therefore there are hopes of getting better or improved treatment plans for the management and maintenance of obesity in consultation with the physical as well as psychological aspects.
However, there are hurdles that have been placed in the way toward using AI and data science in obesity management. The issues such as data protection, lack of data of the minorities, and the integration of AI in the practice can also arise and must be addressed for these technologies to be efficiently utilized. However, prospects of obesity management for the future do not seem so bleak, all because of credit to the use of artificial intelligence and data analytics, to curtail this vice that is ravaging the world.
In this article, we present what techniques current and future AI and data science can bring to the discussion of obesity alongside with recommendation of analytic approaches as well as real-time prediction of treatment, behavioral analysis, and genomic ones. A discussion of implications, specifically limitations and future research and clinical applications of these technologies is also provided, with an emphasis on the ability of the technologies’ clinical applications in ameliorating obesity and improving clients’ quality of life. Table 1 provides a concise overview of key terms related to obesity, which can be useful in understanding the complex aspects of the condition and its management.
Table 1: Key Terms and Descriptions Related to Obesity.
Keyword | Description |
Metabolic Syndrome | A cluster of conditions linked to obesity, including hypertension, high blood sugar, and excess body fat, increasing the risk of cardiovascular diseases and diabetes. |
Body Mass Index (BMI) | A measure used to categorize individuals as underweight, normal weight, overweight, or obese based on their weight relative to height. |
Visceral Fat | Fat stored around the internal organs in the abdominal cavity, which is associated with higher risks of metabolic and cardiovascular diseases. |
Obesity Epidemic | The global increase in obesity rates, characterized by widespread prevalence and significant public health implications. |
Behavioral Interventions | Strategies aimed at changing eating habits, increasing physical activity, and promoting lifestyle modifications to manage or prevent obesity. |
Overview of Obesity –
Obesity is quickly becoming a universal disease that is now on the rise being an epidemic on the global population cutting across all age and economic classes. Obesity is a clinical condition that is defined by the intake and storage of body fat more than biological requirements; body mass index (BMI) is typically prepared from weight and height figures. However, the phenomenon of obesity is described inadequately by BMI index since it is the result of multiple factors involving genetics, metabolism, behavior, and the environment. Obesity has become rampant in recent decades with; currently, over 650 million adults, and 340 million children and adolescents are considered obese. This rise in obesity is associated with the rise in desk-bound job options, high calorie, nutrient-poor diets, or any other form of lifestyle changes that go hand in hand with the process of urbanization and economic development.
The obesity has serious and diverse outcomes for health. The condition is also considered as a significant and stable risk factor to other chronic diseases such as type 2 diabetes, cardiovascular diseases, hypertension, cancer, and arthritis among other skeletal disorders[4]. Obesity also increases the incidence of clinical disorders and mortality; besides, obesity affects the quality of life. In addition to the effects on the people’s health, obesity also affects the health care systems by raising the costs of treatment of diseases associated with obesity. Going further into the effects of obesity in society, the productivity at the workplace and mental health of employees is affected all because of obesity.
Even though obesity is one of the most recognized and studied areas in the field of public health, traditional techniques like use of diets, increased physical activity, and behavior modification have frequently failed, especially when it comes to effective and sustained weight loss. Indeed, the nature of obesity is marked by significant interaction of genetic, environmental, and behavioral factors. Therefore, it is necessary to apply more sophisticated and individualized methods of prevention and treatment of this pathology. Therefore, people are in search of new forms which include the use of the advanced technologies to intervene and manage obesity at the individual and population levels.
The Role of Data Science in Understanding and Managing Obesity –
Big data processing is revolutionizing the park of obesity research and its prevention and intervention by mining large and complicated datasets to produce more information than before. Being complex, involve genetic, environmental, behavioral, and socio-economic factors, the approach to analysis of obesity should allow for processing of the data of various types as to disclose all factors that result in this condition.
Through collection of information from EHRs, patient’s DNA, devices, surveys on the lifestyle, and social network accounts, data science can gather complete data on all the aspects that lead to obesity[5]. This integration of data helps the researchers or the healthcare providers to get the picture of possible specific risk factors that might lead an individual to obesity like gene markers, rates of metabolic, or lifestyle etc. For instance, predictive models resulting from machine learning algorithms, can estimate the probability of an individual becoming an obese based on the explained information. These models may also flag those who are potential candidates for obesity before it has fully developed, thus the interventions will be more effective because they are done at the right time.
In the concept of treatment, data science plays an essential role in formulating customized treatment procedures since treatment is not a general solution to the problem. Therefore, using data science an individual’s dietary preference, the kind of activity that the person prefers, or the metabolism of body can be well analyzed and thus the kind of diet and exercise regime that is personalized as a way of improving on it. This form of intervention enhances the chances of attaining long-term gains in obesity management because people are provided with unique care plans.
In addition, data science also helps in the ongoing updating and tracking of the effectiveness of such individualized treatment regimes. New clinical findings can be collected from the devices and other data sources and compared to the patient’s progress to make necessary changes to the treatment plan to maintain its effectiveness in the future. This dynamic process of obesity management not only makes the process more effective but also increases patients’ compliance with the treatments suggested.
Further, they have the role of explaining behavioral and psychological problems associated with obesity. Depending on the kind of inputs, data science can discover regularities and prompts linked to eating habits, physical activity, and emotional health in case the input is generated from social media posts or the patients’ feedback. This understanding can help to build more extensive interventions which will be targeting the physical as well as psychological meaning of obesity.
To sum up, data science has become a panacea for curing obesity by explaining its way, analyzing the multifaceted and heterogeneous data, constructing different models and forecasts, designing individual and interactive treatment plans, and observing patients’ outcomes in real-time. In the future, data science will be also valuable for the fight against obesity, which is an urgent global problem; as a result, new and efficient methods for the prevention and treatment of this phenomenon will be created of which better correspond to the needs of patient and population. Figure 1 explains the detailed flowchart of understanding and managing of rare diseases using AI and data science.
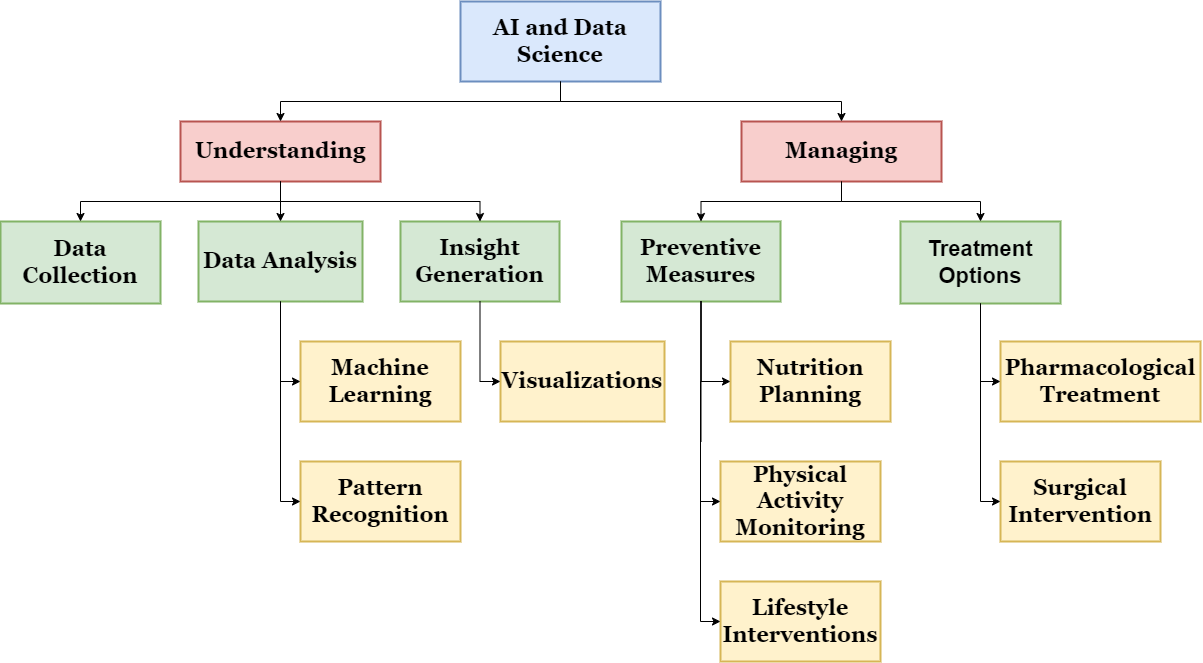
The Role of Artificial Intelligence in Understanding and Managing Obesity –
Such diseases like obesity have now started being managed by AI, as the technology has indicated more efficient approaches necessary for analyzing, forecasting, as well as countering, obesity. The detailed client’s data derived from EHRs, one’s genome, and other factors leading to obesity elaborated by machine learning are large and elaborate in nature. This in turn assist in the generation of models for an individual’s obesity risk and therefore contradict potential obesity.
It is also notable in recommending first-line treatment tailored to the patient’s specifics. Depending on the personal material like genes and daily routines of those who suffer from obesity, it is possible to get different dieting and exercising schedules that raise the chances of effective obesity treatment greatly. Moreover, AI has abilities to monitor the gathered progresses from the wearable devices and make necessary changes for the improved result on treatment plans.
Besides, the excessive weight of the population, as well as the difference between incidences and prevalence in obesity in governmental organizations, can be studied by AI to create personal solutions for the population campaigns. It also emphasizes the psychological approach towards obesity using NLP which arises from one of the methodologies used, to decipher a pattern of behavior from the information that lacks any specific format or categorization for it to be considered as such. Further, in the analysis of medical images and the recognition of patients’ body composition, there are improvements towards the effectiveness of the attribute of diagnosis and monitoring cue for a healthier treatment.
Therefore, it can be stated that AI is a valuable tool in predicting and preventing obesity, diagnosing it, and controlling its further development and symptoms, which essentially means that numerous opportunities will emerge for concentrating effective prevention and treatment at different stages of the disease.
The Combined Power of AI and Data Science in Tackling Obesity –
Both AI and data science can help to manage obesity given that both functions are integrated in the diagnosis, prediction, and treatment of the condition. AI refers to the techniques that study massive data from patients’ electronic records, genetic data, and their lifestyle, among others, and more to find out the results of their research and more to point out researchers the risk factors. Analytics guarantees that such data is processed correctly that makes the insights of AI realizable.
Together with other clinical data it can provide information about an individual’s risk and necessity of an early intervention related to overweight and obesity. AI and data science are also used in real-time monitoring using wearable devices for early intercostals adjustments of therapeutic plans. On the population side, they assist in establishing the prevalence of issues common in a specific population or demographic and the differences between them. Namely, AI and data science are accurate in their prediction, tailored to a patient, and can analyze the whole population, thus being useful in addressing obesity.
Application of AI and Data Science in Obesity Management –
Computer aided intelligent techniques and data science has been integrated into the management of Obesity, because of its efficiency in presenting new strategy for the control of the disease. These technologies help to state risk indicators utilizing big data from eHealth, genomics, and lifestyle records of people with the aim to find the obese ones. It can estimate probable obesity risks and the appropriate actions must be taken as early as possible. In addition, AI-based solutions create personal health plans according to the specific personal data regarding metabolism rate, a diet, and physical activity considering the case-based approach. In addition, these applications are made effective through integration of Wearable Technology by constantly monitoring the progress of the client and the adjustment of the treatment programs on the spot. At the macro level, the AI and data science help the governmental frameworks to work with the population level data and understand the patterns for the heath and diseases which require intervention to change the overall condition of the populace. Thus, these applications improve the results and effectiveness of obesity management and help to improve the health outcomes and become more active to address the problem of obesity internationally.
Challenges and Future Prospects –
However, issues arise as to the shortcomings associated with the use of AI and data science pertaining to obesity management. Another issue can be identified in relation to the protection of provided personal data and their non-disclosure[6]. Due to the collecting and processing of personal health information that involve patient data there has been concern on how this information is dealt with raising the question on how to protect Patients’ data and ensure that the organization is compliant. However, it must be added that the efficiency of AI models to an extent depends on the quality and, especially, on the representativeness of data sets used for AI models training. What has been realized is that many datasets are relatively unrepresentative, and this can be a major challenge if the result includes the prediction followed by the intervention, because the latter will also be less representative for the targeted population.
Yes, there are practical difficulties in applying AI tools to the existing health care systems now. The following are some of the problems that may prevent the adoption of healthcare technologies – reliability, training, disruption of practice cultures. This is perhaps the most crucial aspect concerning the realization of AI solutions as users desire the solutions to be as simple to integrate in the existing systems as possible.
On the same note, regarding the prospects of AI and data science in obesity management soon, several speakers offer some inputs on it. Future advancements in technology would improve the functionality of the myths, as well as the personalization of the therapy[7]. The elaboration of the algorithms with higher quality and variety data and the addition of more and diverse data sources will provide a broader perspective of the cause of obesity by considering the interaction of multiple factors. Correspondingly, the trends in research and technology are expected to prompt the need for new instruments and techniques in monitoring and treatment of the disease of streaming.
Lastly, it is safe to suggest that several challenges notwithstanding, the pioneers in AI and data science shall continue rendering the fight against obesity the much-needed progressive shape. Thereby easing the global burden of obesity and promoting a healthier population of human beings.
Conclusion –
Therefore, the adoption of AI and data science in managing obesity challenges is a revolutionary change in the recognition of obesity and the formulation of solutions towards combating the condition. Since these technologies rely on the use of algorithms as well as an extensive and intensive collection of data, they introduce novel ways through which risk can be assessed, treatment given, and the application of interventions made. Nonetheless, there are opportunities presented by BI such as. Improved data quality, Accomplishment of set business goals, reduction of cost, Increased organizational productivity, Accomplishment of business strategies, Enhanced decision-making & control, and data security & privacies. In the future as the technology behind AI and data science improves-obesity can be fought more effectively due to the enhanced accuracy of the insights and the efficiency of the strategies that can be designed by the systems. It will remain imperative to innovate and employ these tools further as the issue of global obesity persists and progresses to further influence the public’s health.
References –
- X. Pang, C. B. Forrest, F. Lê-Scherban, and A. J. Masino, “Prediction of early childhood obesity with machine learning and electronic health record data,” Int. J. Med. Inf., vol. 150, p. 104454, Jun. 2021, doi: 10.1016/j.ijmedinf.2021.104454.
- A. Chatterjee, M. W. Gerdes, and S. G. Martinez, “Identification of Risk Factors Associated with Obesity and Overweight—A Machine Learning Overview,” Sensors, vol. 20, no. 9, Art. no. 9, Jan. 2020, doi: 10.3390/s20092734.
- S. Arslan, “Exploring the Potential of Chat GPT in Personalized Obesity Treatment,” Ann. Biomed. Eng., vol. 51, no. 9, pp. 1887–1888, Sep. 2023, doi: 10.1007/s10439-023-03227-9.
- C. Breen et al., “Obesity in Adults: A 2022 Adapted Clinical Practice Guideline for Ireland,” Obes. Facts, vol. 15, no. 6, pp. 736–752, Oct. 2022, doi: 10.1159/000527131.
- M. Safaei, E. A. Sundararajan, M. Driss, W. Boulila, and A. Shapi’i, “A systematic literature review on obesity: Understanding the causes & consequences of obesity and reviewing various machine learning approaches used to predict obesity,” Comput. Biol. Med., vol. 136, p. 104754, Sep. 2021, doi: 10.1016/j.compbiomed.2021.104754.
- M. Rahaman, C.-Y. Lin, P. Pappachan, B. B. Gupta, and C.-H. Hsu, “Privacy-Centric AI and IoT Solutions for Smart Rural Farm Monitoring and Control,” Sensors, vol. 24, no. 13, Art. no. 13, Jan. 2024, doi: 10.3390/s24134157.
- T. Haksoro, A. S. Aisjah, Sreerakuvandana, M. Rahaman, and T. R. Biyanto, “Enhancing Techno Economic Efficiency of FTC Distillation Using Cloud-Based Stochastic Algorithm,” Int. J. Cloud Appl. Comput. IJCAC, vol. 13, no. 1, pp. 1–16, Jan. 2023, doi: 10.4018/IJCAC.332408. Vajrobol, V., Gupta, B. B., & Gaurav, A. (2024). Mutual information based logistic regression for phishing URL detection. Cyber Security and Applications, 2, 100044.
- Gupta, B. B., Gaurav, A., Panigrahi, P. K., & Arya, V. (2023). Analysis of cutting-edge technologies for enterprise information system and management. Enterprise Information Systems, 17(11), 2197406.
- Gupta, B. B., Gaurav, A., & Panigrahi, P. K. (2023). Analysis of retail sector research evolution and trends during COVID-19. Technological Forecasting and Social Change, 194, 122671.
Cite As
Nagishetti P. (2024) AI and Data Science in Understanding and Managing Obesity, Insights2Techinfo, pp.1