By: Dhanush Reddy Chinthaparthy Reddy, Department of Computer Science and Artificial Intelligence, Madanapalle Institute of Technology and Science, Angallu(517325), Andhra Pradesh
ABSTRACT
Therefore, in the dynamic field of network technology, development of connectivity and communication has been achieved at a very fast pace. But it has also created a way for envisioned threats in the cyber space and they are a biggest problem for network security. AI is one of the most important resources through which the network security can be reinforced more; it can detect the threats more effectively and even evaluate them and can provide the response for the security incidents as well. Therefore, it is necessary to focus on the following question of the paper: How is AI used in network security? What are the strength and opportunities as well as the weakness and threats? In brief, we discuss about the kinds of AI and some of it’s subcategories like the machine, learning and deep learning and neutral networks and how they can be applied in the detection of threats in cyberspace and how to fight the threats. The paper also attempts to identify the probable ethical issue and the prognosis towards the use of AI in the network security domain and there is always a need to initiate more research work as there is a continuous emergence of new threats.
Keywords: Artificial Intelligence, Threat Detection, Network Security
Introduction
Given the now common state of affairs in a technological world of developing threats and constant cyber attacks, this push for increasing the levels of network security has never been more relevant. While organizations’ operations depend on internal networks, their weaknesses have grown proportional to their interconnectivity, making conventional security mechanisms insufficient. This environment has however forced the search for new solutions especially the adoption of Artificial Intelligence (AI) in network security architecture. With complex algorithms and machine learning at their core, AI systems are expected to significantly change how organizations deal with security threats by offering IT analysis and forecast. Through the incorporation of Artificial Intelligence within an organization’s security infrastructure, not only is the ability to provide a defensive layer increased but also the ability to respond proactively to new threats thereby making the network environment more resilient. This work will focus on the subject of how AI is revolutionizing the network’s security system to discuss some of the opportunities and threats associated with the concept as well as the potential ethical concerns that will be at the horizon of network security in the future.
AI Techniques in Network Security
The definition of network security is the plans and measures put in place by managers to ensure body control of misuse, theft, vandalism, or piracy of networking facilities. Among the contemporary strategies of improving the network security system, there is the application of artificial intelligence. Machine learning which is a subcategory of artificial intelligence enables security models to learn from the data fed into the model and modify itself from the new data without requiring reprogramming. In other words, it entails developing appropriate software that can access information and perform calculations and come up with results on its own.
Cybersecurity is confronted with many obstacles such as DoS and DDoS, fraud, online spam, and spoofing. Some of them are closely connected with the emergence of anomalies, others are characteristic solely for inflation. For the last few years, different techniques have been proposed for the detection of Anomaly based on the automated procedure that includes static code analysis technique and the dynamic Malware behaviour analysis. Lately, the employment of learning-based approaches in particular has increased and has greatly enhanced the struggle and defences against malware authors. The usefulness of the machine learning tools is that they do not need to be explained each activity in detail as long as the tasks similar to earlier ones are being carried out.
Another approach of performing attacks in the context of network intrusion detection is to search for malware in network activities. The fundamental rule is to focus on the operator of the system, as well as the nature of the found holes, the possible consequences of the oversight of certain attacks, and the expertise of the attackers themselves. This way, the administrators gain tighter control over particular detectors, stemming the tide of cross-classification errors. One has to know what the system can and cannot see, why some detections fail, and improve the process incessantly.[1]
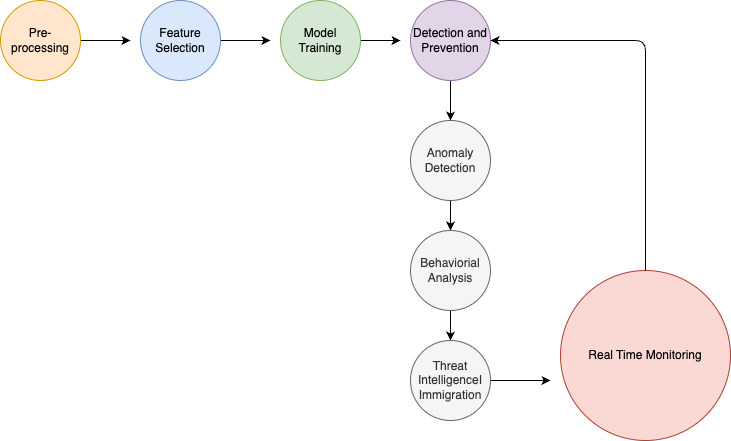
Applications of AI in Network Security
From the technical point of view, improvement of the network security measures is characterized by several significant factors. It demands the capability of scientifically evaluating the risks of the network, identifying security threats in the products of the network, dynamically supervising the condition of the network, accurately observing the signs of the abnormalities of the network, promptly and efficiently handling the network emergencies, and rapidly restoring the network after the attacks of the disasters. All these capabilities require the networks to have a sound and efficient defence mechanism since they are real-time and complex.
In terms of military attack Cyber security threats means Cyber warfare threats. One of the favourable strategies that have been acclimated by organizations is the proactive defence approach. This means identifying the existing voids and shortcoming in the conventional network protection solutions and preparations, especially in the regions like China. Thus, the strategic guideline of active defence is perspective, whereas the phrase “It can be worse if you become a victim” is a reaction of scientific work upon the attack that is not transformed into the perspective of active defence. It is with this proactive strategy toward cyber warfare that China’s national defence policy, entailing defence, has to be understood. For active defence in Computer Network Operations, there are desires to attack, defence, and self-defence; to conquer and to prevent; to win and to deter; all of these are approached in China’s policy of peaceful development in Network Technology.
While Ai proliferates in the methods of network attack and protection, it is essential to define the most effective opportunities of integrating AI with the existing security mechanisms. Thus, those who possess the best technologies, data, and models in this respect obtain an important competitive edge that results in superior cyberspace fight capability and establishing dominance in a cyber conflict.
To sum up, it is necessary for China to strengthen the strategic use of AI in the security of networks, with the need to resist new threats and take proactive measures. This entails the matters of concern as the practical context of AI in the attack and defence of the network entail; data management, confrontation approaches, and evaluation. It is considered the imperative for the advancement of AI from ideas to products.
Thus, intelligence perception and response capabilities shall enhanced to identify new security threats in cyberspace due to AI. This means that China’s sovereign position in cyberspace is defended as well as the main generic interests guaranteed whereas security and development are preserved in the context of a transformative role played by artificial intelligence.[2]
Future Prospects
6G technology will not have centralized cloud-based AI but will implement edge intelligence in every point. This shift results in edge-based federated learning, that improves network security in a world with more devices and data, and efficient communication. Therefore, the concept of the 6G architecture is introduced as connected intelligence, and it will make use of AI at different layers of the hierarchy. For example, by AI at the small cell level, DoS attacks can be avoided on cloud servers at the grass-root level.
The base station of a mesh network obtains multiple paths for connectivity and thus, several base stations can classify device behavior with the help of AI classification algorithms[3]. Overall, it is these stations which by use of weighted average schemes define the authenticity of a device. This behavior-based approach reduces the overhead owing to Key exchange, which is quite a frequent occurrence with tiny cells and multiple access technologies resulting in handovers[4]. Sophisticated control schemes can be also incorporated at the sub-network and wide area network levels with the help of federated learning. The trust scores that form within the sub-graphs in the created sub-network can be taken out and used only when communication is needed to occur with the exterior.
The learning-based intrusion detection approaches are efficient in the case of the attacks on both the control and user plane management system since edge devices already have the data required for intelligent service satisfying the above requirement[5]. Platforms such as Zero-touch Service Management (ZSM) include domain analytics and intelligence services for handling networks’ management with little or no human intervention, which mainly rely on AI capabilities. Security components of these frameworks include assessment of the AI model and AI engines in API security to improve the security of the ZSM architecture.
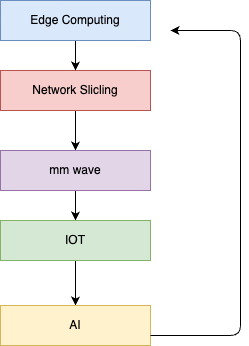
Reference
- J. Banerjee, S. Maiti, S. Chakraborty, S. Dutta, A. Chakraborty, and J. S. Banerjee, “Impact of Machine Learning in Various Network Security Applications,” in 2019 3rd International Conference on Computing Methodologies and Communication (ICCMC), Mar. 2019, pp. 276–281. doi: 10.1109/ICCMC.2019.8819811.
- Y. Zeng, “AI Empowers Security Threats and Strategies for Cyber Attacks,” Procedia Comput. Sci., vol. 208, pp. 170–175, Jan. 2022, doi: 10.1016/j.procs.2022.10.025.
- Y. Siriwardhana, P. Porambage, M. Liyanage, and M. Ylianttila, “AI and 6G Security: Opportunities and Challenges,” in 2021 Joint European Conference on Networks and Communications & 6G Summit (EuCNC/6G Summit), Jun. 2021, pp. 616–621. doi: 10.1109/EuCNC/6GSummit51104.2021.9482503.
- M. Rahaman et al., “Port-to-Port Expedition Security Monitoring System Based on a Geographic Information System,” Int. J. Digit. Strategy Gov. Bus. Transform., vol. 13, pp. 1–20, Jan. 2024, doi: 10.4018/IJDSGBT.335897.
- M. Rahaman et al., “Utilizing Random Forest Algorithm for Sentiment Prediction Based on Twitter Data,” 2022, pp. 446–456. doi: 10.2991/978-94-6463-084-8_37.
- Sharma, A., Singh, S. K., Kumar, S., Thakur, R., Gupta, B. B., & Arya, V. (2024). IoT-enabled smart farming with Industry 5.0. Journal of High Speed Networks, (Preprint), 1-20.
- Rahaman, M., Lin, C. Y., Pappachan, P., Gupta, B. B., & Hsu, C. H. (2024). Privacy-Centric AI and IoT Solutions for Smart Rural Farm Monitoring and Control. Sensors, 24(13), 4157.
Cite As
Reddy D.R.C. (2024) AI in Network Security: What You Need to Know, Insights2Techinfo, pp.1