By: Dadapeer Agraharam Shaik , Department of Computer Science and Technology, Student of Computer Science and technology, Madanapalle Institute of Technology and Science, Angallu,517325, Andhra Pradesh.
Abstract:
Artificial Intelligence (AI) can thus be said to have proven to be useful when it comes to increasing the levels of security and protection in a system. AI use in cybersecurity does come with several issues that need to be ironed out to enhance applying AI in securing organizations. This article discusses the major problems involved in incorporating AI into CS frameworks and tools such problems include matters concerning data quality and access, the tendency of the AI model’s odd, vulnerability to adversarial attacks, continual learning, and adaptation, and what could be considered ethical issues and privacy issues. It is essential to comprehend these issues in order to create viable AI-cantered cybersecurity that could obstruct the constantly changing threat environment.
Keyword’s: AI, Cybersecurity, Privacy, Machine Learning, Threat Detection.
1.Introduction
Cybersecurity is nowadays an essential aspect of contemporary societies, and AI plays a crucial role in present and future utilization in cybersecurity practices. The artificial intelligence capability to study large amount of information and define tendencies as well as make estimations regarding possible threats has further transformed the efficiency of security precautions in the sphere of cyber security. Given the fact that attackers are getting bolder and more innovative and attacks are becoming a daily occurrence some of the traditional security solutions prove inefficient so a shift to AI is essential. Nevertheless, the use of AI in cybersecurity comes with some problems. There are several challenges that have to be met hence forward including but not limited to the following; Sufficiency of high quality data; The profound nature of models that lay down AI; and Adversarial threats. Third, as created threats are ever-evolving and diverse, it imposes the learning process continuously, thus raising questions about such systems’ ethics and privacy. This article analyses these issues giving proper understanding of the obstacles that could be met when implementing artificial intelligence based security solutions to networks and how these problems could be mitigated to improve the overall network security.
2.Challenges in Implementing Privacy-Preserving Techniques in AI-Powered Cybersecurity
Key Challenges
- Computational Overhead: Indeed, it is mostly well known that most privacy preserving techniques come with the catch that they have to grossly limit system speed and size.
- Interoperability and Compatibility: When applying several heterogeneous privacy preserving strategies the inclusion of them into synoptic systems is challenging because these strategies are incompatible most of the time.
- Regulatory Compliance: Balancing the enforcement of some of laws such as GDPR when implementing new enhanced AI cybersecurity systems is a challenge.
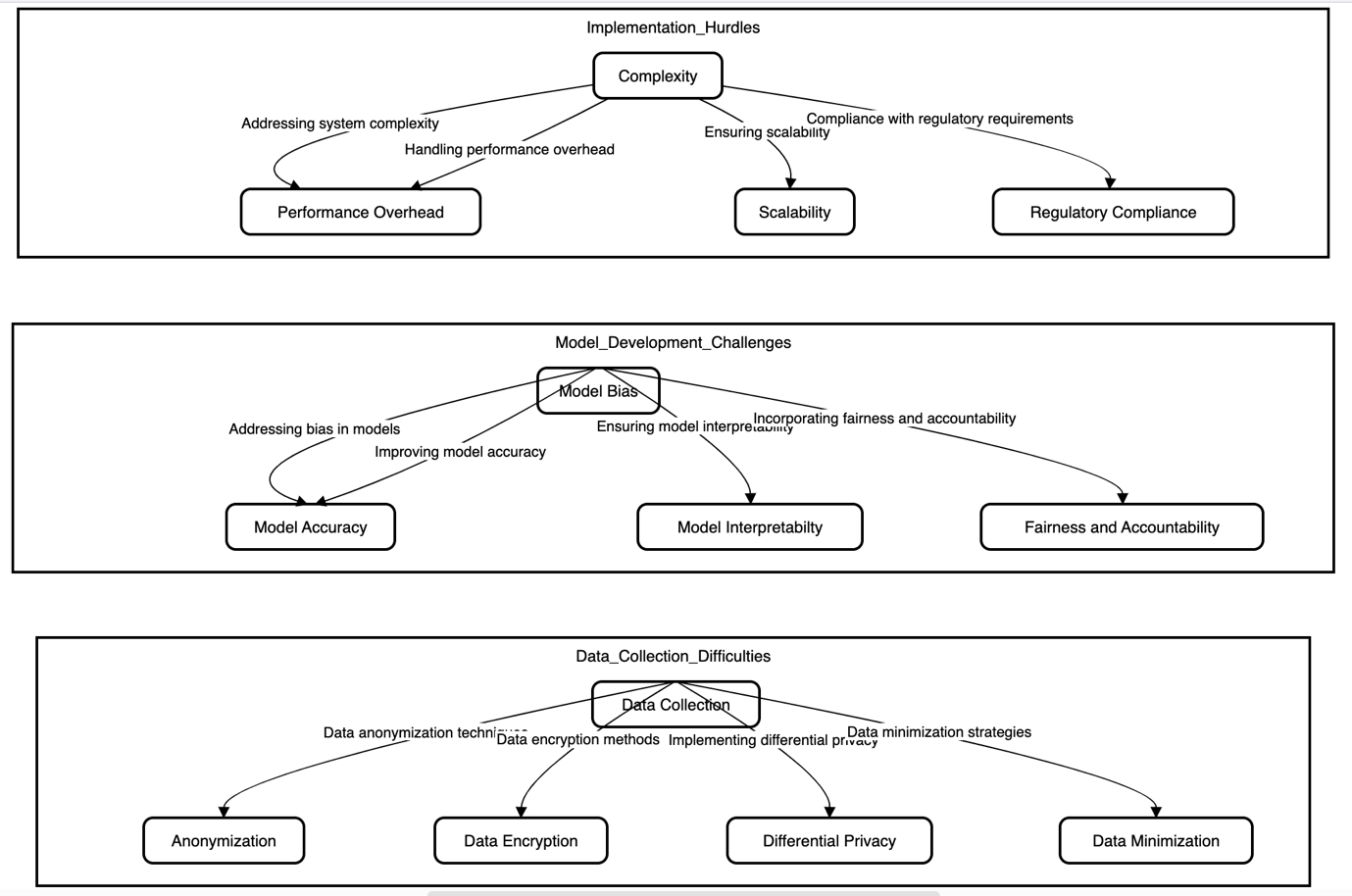
Future Research Directions
- Hybrid Privacy-Preserving Techniques: Integrating the methods to the protection approaches where used (for example differential privacy, homomorphic encryption, federated learning) to get better balance in the approaches or to improve overall effectiveness of the approaches.
- Enhanced Computational Efficiency: New emergent techniques or improvement needed for decreasing the time used to make the numerical calculations performed, where individual’s privacy is adequately protected.
- Interdisciplinary Collaboration: This can be done through a synergy meaning multidisciplinary cooperation of cybersecurity experts, data protection officers, AI developers, cryptologists, lawyers who all focus on creating data privacy.
- Ethical and Legal Considerations: Analysing and looking at how these techniques are beneficial for the society, What assumptions are there while developing them, Ethical considerations within the privacy-preserving techniques in AI-based security. Amendments to existing new and changing legislation.
- Education and Awareness: Aware developing, policy making and the final ordinary user about privacy, ethics of AI and general, safe handling of data.
As for these challenges and the illustrated directions of research, it is possible to proceed with the advancement of the more effective AI security solutions that safeguard people’s privacy. [1]
3.Enhanced Cybersecurity through Predictive Analytics
Predictive analytics is becoming a significant weapon in the cybersecurity defense. This enables firms to have an early warning system in place, which can help them anticipate potential threats and secure their systems and data[2].
Major Benefits of Predictive Analytics for Cybersecurity
- Early Threat Identification: By studying distributed logs and dark web traffic, predictive analytics can detect signs that show how an attack is building up.
- Pattern Recognitions: Unearthing new attack campaigns and activities by finding linkages and patterns across diverse datasets including human and cyber domains.
- Understanding the intentions of enemy attackers: Getting inside the head of enemies can reveal what motivates them, what they are capable of doing, or how their defence’s might impact others.
- Enhanced Response Strategies: Information generated by predictive analytics could be used to develop better protection methods as well as recovery plans that are more effective.
- Better Situational Awareness: The internal structure of a system, patching trends within it as well as security controls form the basis for security posture analysis using predictive analytics.
Challenges and Opportunities
- The Quantity and Quality of Data: Getting enough clean labelled training data predictive models remains’ a significant challenge.
- Misdiagnosis and False Positives: Due to the high complexity of AI Analytics and diverse data sets, there is a need for robust validation procedures in order to avoid false alarms.
Transparency of AI models: There is an urgent need to understand why AI-based predictions are made to ensure trustworthiness and ethicality [3].
The Future Research Focus
- Development of Data Augmentation: Developing techniques that can augment limited labelled dataset, so that better performing models can be initiated.
- model validation is done by setting very high verification standards for cyber security predictions that uses AI technology.
The task assignment and interaction between humans and AI with substantially improved threat identification and elimination[4].
- Ethical Concerns: Concerns with privacy and self-prejudices if artificial intelligence is used in the area of security[5].
It is imperative that predictive analytics be considered as one of the fundamental components of resilient cyber security on offense that can overcome such challenges as well as opportunities. [2]
Conclusion:
Thus, the involvement of artificial intelligence in the sphere of cybersecurity is a trend to improve defenses against new threats. With this technology, any form of cyber-attacks will be identified and dealt with by the computer programs. However, a few limitations are as follows; There are adversarial attacks, issues related to privacy, and there are questions of ethics which require attention. Perhaps the most critical area to address is the ability of such systems to be capable of continual learning as the new and formidable enemies do not plan to stand still. As this, to understand and optimize the AI opportunities in the future while keeping possible negative impacts under control, future technologies needs to be developed and analysed from different perspectives and by attracting specialists in different fields.
Reference:
- D. V. V. Vegesna, “Privacy-Preserving Techniques in AI-Powered Cyber Security: Challenges and Opportunities,” Int. J. Mach. Learn. Sustain. Dev., vol. 5, no. 4, pp. 1–8, Dec. 2023.
- S. Suripto, G. Utomo, K. Purwanto, K. Trinanda Putra, M. Mustar, and M. Rahaman, “Design and Analysis of Solar-powered E-bike Charging Stations to Support the Development of Green Campus,” J. Electr. Technol. UMY, vol. 6, pp. 85–93, Dec. 2022, doi: 10.18196/jet.v6i2.16543.
- V. D. Soni, “Challenges and Solution for Artificial Intelligence in Cybersecurity of the USA,” Jun. 10, 2020, Rochester, NY: 3624487. doi: 10.2139/ssrn.3624487.
- L. Triyono, – Prayitno, M. Rahaman, – Sukamto, and A. Yobioktabera, “Smartphone-based Indoor Navigation for Guidance in Finding Location Buildings Using Measured WiFi-RSSI,” JOIV Int. J. Inform. Vis., vol. 6, no. 4, pp. 829–834, Dec. 2022, doi: 10.30630/joiv.6.4.1528.
- M. Rahaman, B. Chappu, N. Anwar, and P. K. Hadi, “Analysis of Attacks on Private Cloud Computing Services that Implicate Denial of Services (DoS),” vol. 4, 2022.
- Vajrobol, V., Gupta, B. B., & Gaurav, A. (2024). Mutual information based logistic regression for phishing URL detection. Cyber Security and Applications, 2, 100044.
- Gupta, B. B., Gaurav, A., Panigrahi, P. K., & Arya, V. (2023). Analysis of cutting-edge technologies for enterprise information system and management. Enterprise Information Systems, 17(11), 2197406.
- Gupta, B. B., Gaurav, A., & Panigrahi, P. K. (2023). Analysis of retail sector research evolution and trends during COVID-19. Technological Forecasting and Social Change, 194, 122671.
Cite As
Shaik D.A. (2024) Challenges of Implementing AI in Cybersecurity, Insights2Techinfo, pp.1