By: Pinaki Sahu, International Center for AI and Cyber Security Research and Innovations (CCRI), Asia University, Taiwan, 0000pinaki1234.kv@gmail.com
Abstract
The use of chatbots in anomaly detection to improve cybersecurity is examined in this article. Chatbots that use machine learning allow for instantaneous anomaly notifications and real-time monitoring. Their proficiency in analysing user behaviour and adaptive learning allows them to adjust to changing threats. Although it offers several advantages, issues like privacy and false positives are also covered. Through the use of chatbots, artificial intelligence and cybersecurity may work together to give organisations a proactive means of reducing potential security concerns.
Introduction
Businesses and organisations are faced with an increasing problem in the quickly changing technological world of safeguarding their systems and data from possible attacks and abnormalities. An essential component of cybersecurity is anomaly detection, which is the process of spotting patterns that diverge from the norm and indicate possible security lapses or anomalies. With the development of machine learning (ML) and artificial intelligence (AI), chatbots have become useful instruments for anomaly identification. This article examines the function of chatbot support in anomaly detection and how it improves efficiency and security.
What is Anomaly Detection?
Finding patterns or occurrences in a system that substantially differ from expected behaviour is known as anomaly detection. Conventional approaches frequently depend on statistical models or rule-based systems. More advanced methods are necessary, nevertheless, because of the dynamic and complicated nature of today’s digital settings[1].
Machine Learning’s Function in Identifying Anomalies:
A branch of AI called machine learning has shown to be quite successful in anomaly identification. Large datasets may be analysed using ML algorithms, which can also be used to find trends and accurately identify abnormalities. Clustering, classification, and ensemble approaches are common machine learning techniques used for anomaly detection[2].
Chatbot Integration for Anomaly Detection:
An increasing number of anomaly detection systems are incorporating chatbots, driven by machine learning and natural language processing (NLP), into their operations. As intelligent assistants, these chatbots watch over and evaluate system behaviour all the time. They are ideally suited for quickly detecting abnormalities since they can process enormous volumes of data in real-time[3].
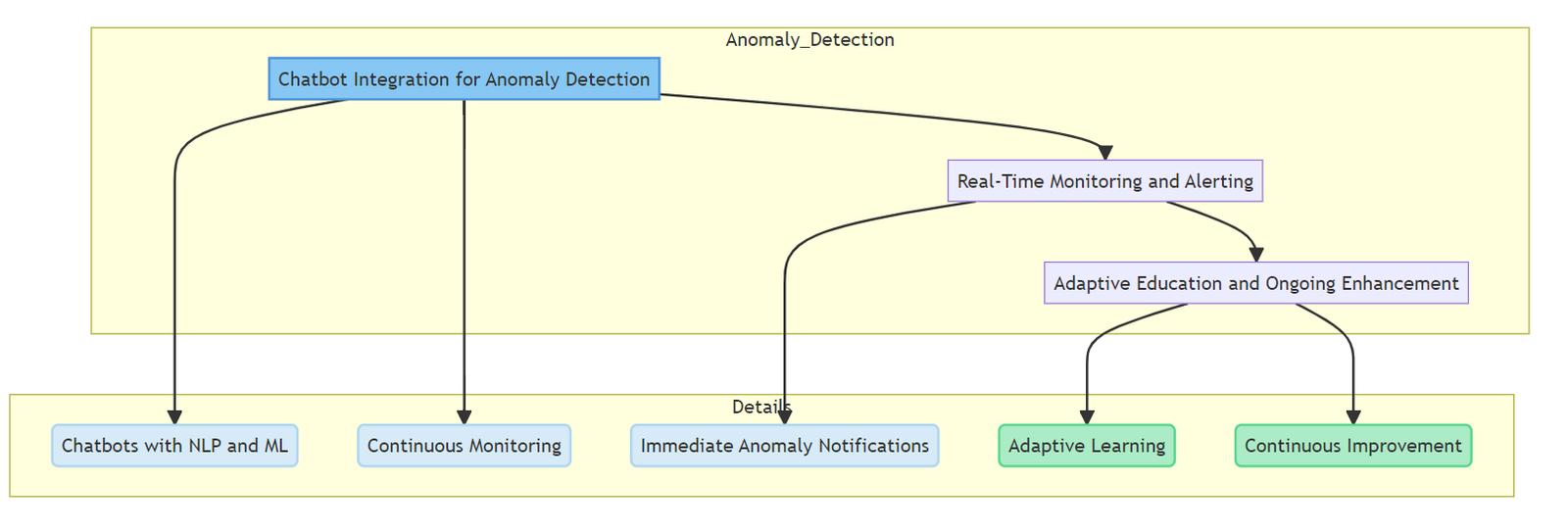
Fig.1 Chatbot Assistance for Anomaly Detection
- Monitoring and Alerting in Real-Time: Chatbots are particularly good at monitoring in real time, they can quickly analyse user activity, network data, and system records. Chatbots can detect anomalies and send out instant notifications, enabling cybersecurity professionals to quickly address possible risks. By taking a proactive stance, security events are lessened in impact and reaction times are drastically cut[2].
- User Behaviour Assessment: The capacity of chatbots to comprehend and evaluate user behaviour is one of its advantages in anomaly detection. Chatbots are able to rapidly detect deviations that can point to unauthorised access or compromised accounts since they are trained to recognise regular user behaviours and access patterns. This method of focusing on the user improves anomaly detection systems’ accuracy.
- Adaptive Education and Ongoing Enhancement: Due to machine learning, chatbots are able to learn from fresh data and adjust to changing threats. The capacity to adapt guarantees that the anomaly detection system will continue to function well even in the face of new security threats. In the ever-changing field of cybersecurity, ongoing education and development are essential.
Challenges and Considerations
Even while chatbot-assisted anomaly detection has many advantages, there are drawbacks to take into account. For businesses using this strategy, eliminating false positives, ensuring the privacy and moral use of data, and smoothly integrating chatbots into current security infrastructures are all important factors to take into account.
Conclusion
The use of chatbots to help discover anomalies is an effective instance of cybersecurity and AI coming together. Because chatbots can comprehend user behaviour, analyse large databases, and send out real-time alerts, they are an invaluable ally in the continuous fight against cyber dangers. Using chatbots for anomaly detection will be increasingly essential as technology develops to keep security measures strong and flexible. Businesses that adopt this cutting-edge strategy will be better able to protect their digital assets and maintain an advantage in the rapidly changing cybersecurity market.
References
- Ahmed, M., Mahmood, A. N., & Hu, J. (2016). A survey of network anomaly detection techniques. Journal of Network and Computer Applications, 60, 19-31.
- Sahu, P. Natural Language Processing Chatbots Assistance for Strengthening Cybersecurity in Messaging Applications.
- Mahajan, S., Wu, W. L., Tsai, T. C., & Chen, L. J. (2018, September). Design and implementation of IoT-enabled personal air quality assistant on instant messenger. In Proceedings of the 10th International Conference on Management of Digital EcoSystems (pp. 165-170).
- Rajput, R. K. S., Goyal, D., Pant, A., Sharma, G., Arya, V., & Rafsanjani, M. K. (2022). Cloud data centre energy utilization estimation: Simulation and modelling with idr. International Journal of Cloud Applications and Computing (IJCAC), 12(1), 1-16.
- Sharma, A., Singh, S. K., Badwal, E., Kumar, S., Gupta, B. B., Arya, V., … & Santaniello, D. (2023, January). Fuzzy Based Clustering of Consumers’ Big Data in Industrial Applications. In 2023 IEEE International Conference on Consumer Electronics (ICCE) (pp. 01-03). IEEE.
- Chui, K. T., Gupta, B. B., Jhaveri, R. H., Chi, H. R., Arya, V., Almomani, A., & Nauman, A. (2023). Multiround transfer learning and modified generative adversarial network for lung cancer detection. International Journal of Intelligent Systems, 2023, 1-14.
- Chui, K. T., Gupta, B. B., Torres-Ruiz, M., Arya, V., Alhalabi, W., & Zamzami, I. F. (2023). A Convolutional Neural Network-Based Feature Extraction and Weighted Twin Support Vector Machine Algorithm for Context-Aware Human Activity Recognition. Electronics, 12(8), 1915.
Cite As
Sahu P. (2023) Chatbot-Assistance for Detecting Anomalies, Insights2Techinfo, pp.1