By: Jampula Navaneeth1
1Vel Tech University, Chennai, India
2International Center for AI and Cyber Security Research and Innovations, Asia University, Taiwan Email: navaneethjampula@gmail.com
Abstract
In the recent days, the cyber threats are becoming more diverse and complex so that applying traditional security measures may not suffice. However, this article focuses on how deep learning, especially neural networks, has played a revolution in improving the cybersecurity system. Such complications using these models will help warrant the detection and prevention of other novel threats like zero-day, malware, and phishing. The dynamic character of deep learning allows for threat and risk assessment as well as defence in the rapidly evolving context of cybersecurity threat. Consequently, this article highlights the need to embrace the higher artificial intelligent technologies into the overall cybersecurity framework to counter present day threats.
Keywords: Cyber Threats, Neural Networks, Deep Learning, Security, Phishing
Introduction
Organisations have found that protection solutions relying on traditional technologies are no longer adequate in view of the evolving, more sophisticated cyber threats [1]. Attackers are always devising new ways through which to penetrate the defences of organizations and indeed organizations are advised to improve their protection. However, this particular field of AI, known as deep learning, applies neural networks to the problem. Since deep learning comprises identifying a set of issues and predicting their yields based on large datasets, then it is efficient in detecting emerging threats as compared to conventional approaches [2]. In this paper, the author attempts to discuss how neural networks enhance cybersecurity while paying an emphasis on their efficiency in the procedures of anomaly detection, malware identification, and phishing prevention.
A Comprehensive Report on Neural Networks in Cybersecurity
Neural networks are algorithms that mimic in action and in its way of dealing with data the human brain. They establish relationships of interconnected nodes, or neurons that create systems for pattern detection or decision-making [3]. In the case of cyber security these networks are trained on large databases of threats and normal traffic patterns and can as such detect and respond to threats as they occur.
In contrast to the known antiviruses and firewalls that are based on definite rules and virus signatures, neural networks are able to learn. This enables them to identify zero-day attacks; these are new threats that existing systems maybe unaware of [4]. The samples of feed-forward and recurrences are used to identify conventional feature of the network traffic, user activity and system log files based on the topological deep learning models to detect the abnormal activity that is most likely to cause a cyber-attack [5].
How Deep Learning Develops New Threats
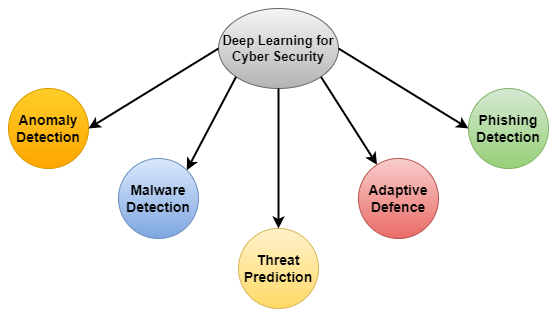
Figure 1: Deep Learning Detects Emerging Threats
- Anomaly Detection: Neural networks are almost impeccable at detecting an anomaly thereby making them excellent in cybersecurity on that aspect alone. This case, by understanding the typical behaviours existing within a system, the deep learning models can detect behaviours that are unusual. For instance, if a user begins interacting with files in ways that are drastically different from usual, for example, administration files the network the neural network would flag the user as a possible insider threat or, more likely, a compromised account [6].
- Threat Prediction: Using present and past data, deep learning models can predict some of the probable threats. These models are able to predict where and when a new attack might happen by identifying patterns of this kind in previous attacks. This predictive capability is of great significance for organizations, which plan their actions and countermeasures against cybercriminals [6].
- Malware Detection: Most antivirus systems can only detect virus ‘signatures’ and therefore, they can easily be outsmarted by new, constantly mutating forms of malware. Neural networks however can recognize a malware each time it executes as oppose to each time it is coded or programmed to look like [7]. And this is all about saying that newly emerging variants of threats, including those which are unknown and have not launched their attacks yet, can be reported.
- Phishing Detection: Security threats include the most recent attack known as phishing where attackers pretend to be an organization hence obtain sensitive information from clients [6]. Different algorithms can help to distinguish a phishing email even by the content and structure of the message. Using cues in language, layout, and the sender’s details, live neural networks can recognize phony emails effectively often even when it is a completely new attack the program has never seen before.
- Adaptive defence: Threats in cyberspace are not static; neither can the measures against them be considered fixed. The tough thing with neural networks is that they can learn about new threats and update themselves on providing connected results by training on new sets of data. This capability for adaptation with respect to new inputs means that deep learning models stay relevant, as do the notations, in the face of shifting tactics [7].
Cyber Security as a field of deep learning in the future
The threat levels in the cyber world does consistently evolve and therefore as the threat levels evolve so does the need for better defence mechanisms such as deep learning. Neural networks can become a useful instrument in identifying and combating new threats due to their ability to minimize rather than maximize risks with the help of algorithms which allows adopting the proactive rather than the reactive approach to cybersecurity in organizations.
However, deep learning in the cybersecurity system has some drawbacks in its application. In other words, it may be concluded that the neural network training data is very important, it has to be good and come from different sources. Also, a threat that seems to seriously threaten the application of neural networks is adversarial attacks, mainly when hackers introduce some discrepancies into data intentionally to mislead the artificial neural network. Nevertheless, the prospects for using deep learning in cybersecurity are great [8].
Conclusion
Deep Learning can be seen as a step up in the fight against cyber threats. Utilizing these neural networks enable organization to react quickly and accurately to threats in ways previously unfathomable. Over time, the precautions of the cybersecurity will improve accompanied by the developed technologies in its defence against constantly evolving strategies employed by hackers. What is the future of cybersecurity going to look like? It’s not about implementing walls; instead, from today’s perspective, the future is about designing and creating heave, self-learning systems that can look, learn, and mimic the human brain.
References
- J. Jang-Jaccard and S. Nepal, “A survey of emerging threats in cybersecurity,” Journal of Computer and System Sciences, vol. 80, no. 5, pp. 973–993, Aug. 2014, doi: 10.1016/j.jcss.2014.02.005.
- Deep Cybersecurity: A Comprehensive Overview from Neural Network and Deep Learning Perspective, (May 01, 2021). doi: 10.1007/S42979-021-00535-6.
- M. Pawlicki, R. Kozik, and M. Choraś, “A survey on neural networks for (cyber-) security and (cyber-) security of neural networks,” Neurocomputing, vol. 500, pp. 1075–1087, Aug. 2022, doi: 10.1016/j.neucom.2022.06.002.
- M. Rahaman, S. S. Bakkireddygari, S. Chattopadhyay, A. L. Gomez, V. Arya, and S. Bansal, “Infrastructure and Network Security,” in Metaverse Security Paradigms, IGI Global, 2024, pp. 108–144. doi: 10.4018/979-8-3693-3824-7.ch005.
- M. A. Ferrag, L. Maglaras, S. Moschoyiannis, and H. Janicke, “Deep learning for cyber security intrusion detection: Approaches, datasets, and comparative study,” Journal of Information Security and Applications, vol. 50, p. 102419, Feb. 2020, doi: 10.1016/j.jisa.2019.102419.
- M. Shahin, M. Maghanaki, A. Hosseinzadeh, and F. F. Chen, “Advancing Network Security in Industrial IoT: A Deep Dive into AI-Enabled Intrusion Detection Systems,” Advanced Engineering Informatics, vol. 62, p. 102685, Oct. 2024, doi: 10.1016/j.aei.2024.102685.
- P. Dixit and S. Silakari, “Deep Learning Algorithms for Cybersecurity Applications: A Technological and Status Review,” Computer Science Review, vol. 39, p. 100317, Feb. 2021, doi: 10.1016/j.cosrev.2020.100317.
- B. Geluvaraj, P. M. Satwik, and T. A. Ashok Kumar, “The Future of Cybersecurity: Major Role of Artificial Intelligence, Machine Learning, and Deep Learning in Cyberspace,” in International Conference on Computer Networks and Communication Technologies, S. Smys, R. Bestak, J. I.-Z. Chen, and I. Kotuliak, Eds., Singapore: Springer, 2019, pp. 739–747. doi: 10.1007/978-981-10-8681-6_67.
- Li, D., Lai, J., Wang, R., Li, X., Vijayakumar, P., Gupta, B. B., & Alhalabi, W. (2023). Ubiquitous intelligent federated learning privacy-preserving scheme under edge computing. Future Generation Computer Systems, 144, 205-218.
- Gupta, B. B., Mamta, Mehla, R., Alhalabi, W., & Alsharif, H. (2022). Blockchain technology with its application in medical and healthcare systems: A survey. International Journal of Intelligent Systems, 37(11), 9798-9832.
- Gupta, B. B., & Narayan, S. (2021). A key-based mutual authentication framework for mobile contactless payment system using authentication server. Journal of Organizational and End User Computing (JOEUC), 33(2), 1-16.
Cite As
Navaneeth J. (2024) Deep Learning Shields: How Neural Networks Detect Emerging Cyber Threats, Insights2Techinfo, pp.1