By: Indu Eswar Shivani Nayuni, Department Of Computer Science & Engineering(Data Science), Student Of Computer Science & Engineering(Data Science) ,Madanapalle Institute of Technology and Science, Angallu(517325),Andhra Pradesh . indunayuni1607@gmail.com
Abstract
In the contemporary world one of the pressing problems that affects almost every aspect of human life and that is presented in both traditional and new media belongs to the Fake News. One of the significant limitations associated with most of the conventional approaches used in detecting misinformation is that most of them are simple and at times, even rely on manual processes and hence address few of the aspects. Therefore, this paper seeks to propose the utilization of generative AI in enhancing the identification of fake news and avert the crafting of similar news items. Since it is generative AI, the technique distinguishes between high-volume data and evaluates the complex ML algorithms and NLP besides identifying typical sentiments seen with fake news, and assessing the credibility of sources. The article is centered on_generative AI Models and deep learning, neural networks to foresee on the efficiency and accuracy of detecting misinformation. This paper also describes how these technologies have the following drawbacks; how to solve them in terms of bias, data quality, and ethical considerations. Based on case analysis and discussion in this article, it is demonstrated that generative AI might be a better solution to respond to threats in maintaining informational truthfulness in a constantly changing technological context than relying solely on the current and known methods.
Introduction
The proliferation of digital media has revolutionized the way information is shared and consumed, but it has also given rise to a significant challenge: It is an important means of countering: the spread of mis and disinformation.[1] This is the misinformation era which has a capacity of bringing down the confidence of the general public, interfering with the election results, as well as catalyzing the diffusion of fake news regarding health issues. Real-time monitoring is challenging with traditional methods of fact-checking and using keyword filters, making it very difficult for the general approaches to track modern fake material efficiently.
Generative AI has the ability to process natural language as well as learn which can be used to set up a counter plan against misinformation. It is also a superior technique in comparison with traditional methods since it can analyze massive amounts of data and also distinguish between truthful and false information with a better degree of accuracy concerning the language and the context.[2]
This article explains the part of generative AI to find misinformation and find a way, how these technology to enhance the efficiency and accuracy of verification information processes. We will explore into the generative AI model’s technical aspects, their application in reality scenarios, and the challenges of implementation. Additionally, this paper will considerations of ethical and biases of potential inherent in driven by AI misinformation detection.
In these times, where false information can spread fastly and have consequences are serious, harnessing the role of gen AI plays a important step towards safe-guarding the reliability and accuracy of data in the digital world.
Comparison: Traditional Misinformation Detection Methods vs. Generative AI
To fully appreciate the transformative impact of generative AI in detecting misinformation, it is essential to compare it against traditional methods across several key dimensions: accuracy, scalability, adaptability, speed, and implementation challenges.[3]
Dimension | Traditional Methods | Generative AI |
---|---|---|
Accuracy and Precision | Manual Fact-Checking: It is correct, however, it is not very effective, gets affected by many human factors, and can take time when dealing with many people and frequently changing transmitted data.
| Natural Language Processing (NLP): It improves precision concerning the textual background, polarity/remoteness of the text, and its language features.
|
Scalability and Speed | Manual Fact-Checking: A. High demand of resources: needs time and also demanded, not suitable when handling large quantities of information, not suitable in a circumstance where new information is released frequently and consist only of false information.
| Real-Time Processing: It can be applied by individuals that are involved in the processing of big amounts of data and/or information to notice and address specific occurrences at great speed.
|
Adaptability and Evolution | Manual Fact-Checking: Requires constant replenishing and strengthening of capacity which cannot be met at the rate information is generated and processed.
| Adaptive Learning: C60 Extensively continues to learn from new data to improve the identification of new trends.
|
Implementation and Maintenance |
Manual Fact-Checking: Very much dependent on the people resource, often manpower intensive, not suitable for a fully automated system.
|
Complex Implementation: Limited compatibility: requires specialized AI/ML knowledge for installation and configuration, also it is quite resource-intensive in terms of the first installations.
|
Ethical and Bias Considerations |
Human Bias: While using manual form of fact-checking, the assessors are likely to incorporate their bias, thus the question of impartiality can arise.
|
Algorithmic Bias: Nearly every AI model is trained using datasets, meaning that it can carry such biased information especially if trained on low-quality and/or less diverse datasets.
|
Analysis of detecting the misinformation with Gen AI
It is has several advantages over the conventional approaches when applied in the detection of the fake news with the use of the generative AI. These include: From the results of the verification procedures, the accuracy of the outcomes is highly enhanced and the processing time is much reduced significantly.[5] Therefore, this analysis is devoted to exploring how and with the help of what a generative AI improves the recognition of mis- and disinformation, related technologies, and challenges that it raises. The following diagram show the analysis of misinformation detection[6].
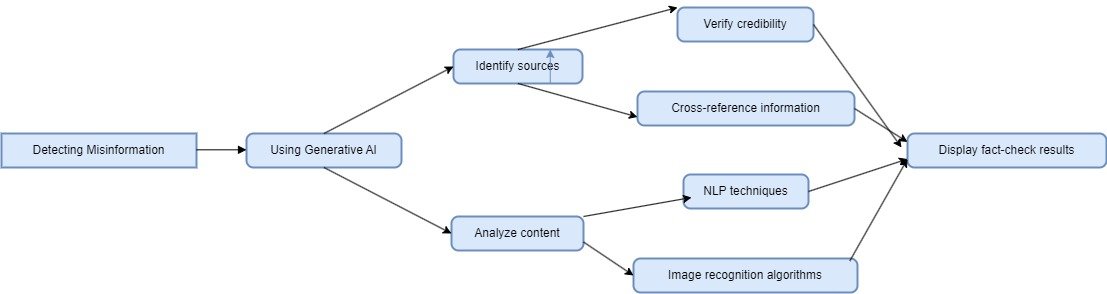
Technological Advancements
Natural Language Processing (NLP): Utilised a more advanced approach in NL – typically to obtain a deeper insight of a string of text. RI can comprehend the context and tone as well as other shades of the language that the plain keyword filtering would not capture[3].
Deep Learning Algorithms: Make complex decisions where patterns and connections in huge databases need to be combined with the help of the neural network mechanisms. [7]These can see much more complicated bots and techniques of misinformation than regular filters can see.
Contextual Analysis: Different from human eyes, AI models can able to have a glance at the environment where the post is going to be put up and identify whether the information centering the post is real or fake or false, and the intention of the author in sharing information[6].
Enhanced Accuracy and Efficiency
Improved Accuracy: Thus, it is much wiser to use AI models as the latter enhance their performance with a vast amount of information and new methods of misuse. This is because they consider the pattern and anomalies to distinguish easily between the accurate and the fake news.
Scalability and Speed: This is why generative AI can thus in the shortest time consume and analyze large volumes of data and in the process identify fake news. This scalability enables one to track wider and much bigger content as compared to the content input in the program.
Application and Real-World Impact
Broad Application: At the same time, it can be used with different media formats and post categories on platform: social networks, articles, forums, etc. This extensive application helps in identifying, and thus, fight against propaganda and fake news on a larger scale[5].
Proactive Detection: AI models are most useful in the determination of new patterns about the flow of misinformation and to inform the users about those patterns before they become hazardous. This is even more helpful in the sense that actually managing the outcomes of fake news becomes a slightly less strenuous affair. [8]
Challenges and Considerations
Bias and Fairness: This infers that, decision making through using the AI models is not bias free since AI models are able to learn bias from the training data for development of the models. This has a special consideration towards the availability of the AI system for bias and under no circumstances must the AI system ever be allowed to have bias[9].
Data Quality: So to make the AI models perform better their samples or the training data that has been provided to them has to be quality and diversity[10]. Specifically, if M is spreading wrong or bias data, then an aim of the strategy employed in combating misinformation is affected[11].
Ethical Considerations: Therefore there is some of the ethical consideration when applying the AI in the monitoring and detection of fake news such as privacy and censorship and misuse of the technology.
Conclusion
Thus, Generative AI is undoubtedly a powerful promotion in the current fight against fakes. It is built on the latter and enhances on NLP and deep learning to monitor the analysed processes of the fake news at a higher level of effectiveness. Due to this reason, the performance analysis of big data in real-time increases scalability and flexibility.
However, there remain some challenges, for instance, the ways of identifying the suitable algorithms, the quality of data retrieved to achieve the effective and responsible AI. In general, it is possible to conclude that the proposition to apply the generative AI can be seen as the certain potential strategy to increase the number of humanities and fight against the fake news presented on the Internet.
References:
- E. Ferrara, “Fairness and Bias in Artificial Intelligence: A Brief Survey of Sources, Impacts, and Mitigation Strategies,” Sci, vol. 6, no. 1, Art. no. 1, Mar. 2024, doi: 10.3390/sci6010003.
- P. Bhardwaj, K. Yadav, H. Alsharif, and R. A. Aboalela, “GAN-Based Unsupervised Learning Approach to Generate and Detect Fake News,” in International Conference on Cyber Security, Privacy and Networking (ICSPN 2022), N. Nedjah, G. Martínez Pérez, and B. B. Gupta, Eds., Cham: Springer International Publishing, 2023, pp. 384–396. doi: 10.1007/978-3-031-22018-0_37.
- P. Pappachan, Sreerakuvandana, and M. Rahaman, “Conceptualising the Role of Intellectual Property and Ethical Behaviour in Artificial Intelligence,” in Handbook of Research on AI and ML for Intelligent Machines and Systems, IGI Global, 2024, pp. 1–26. doi: 10.4018/978-1-6684-9999-3.ch001.
- B. Do, A. Dadvari, and M. Moslehpour, “Exploring the mediation effect of social media acceptance on the relationship between entrepreneurial personality and entrepreneurial intention,” Manag. Sci. Lett., vol. 10, no. 16, pp. 3801–3810, 2020.
- D. I. Mahmood, “Disinformation and Democracies: Understanding the Weaponization of Information in the Digital Era,” Policy J. Soc. Sci. Rev., vol. 2, no. 02, Art. no. 02, Dec. 2023.
- M. Moslehpour, A. Khoirul, and P.-K. Lin, “What do Indonesian Facebook Advertisers Want? The Impact of E-Service Quality on E-Loyalty,” in 2018 15th International Conference on Service Systems and Service Management (ICSSSM), Jul. 2018, pp. 1–6. doi: 10.1109/ICSSSM.2018.8465074.
- R. Gupta, K. Nair, M. Mishra, B. Ibrahim, and S. Bhardwaj, “Adoption and impacts of generative artificial intelligence: Theoretical underpinnings and research agenda,” Int. J. Inf. Manag. Data Insights, vol. 4, no. 1, p. 100232, Apr. 2024, doi: 10.1016/j.jjimei.2024.100232.
- A. M. Widodo et al., “Port-to-Port Expedition Security Monitoring System Based on a Geographic Information System,” Int. J. Digit. Strategy Gov. Bus. Transform. IJDSGBT, vol. 13, no. 1, pp. 1–20, Jan. 2024, doi: 10.4018/IJDSGBT.335897.
- B. Martens, L. Aguiar, E. Gomez-Herrera, and F. Mueller-Langer, “The Digital Transformation of News Media and the Rise of Disinformation and Fake News,” Apr. 20, 2018, Rochester, NY: 3164170. doi: 10.2139/ssrn.3164170.
- L. Triyono, R. Gernowo, P. Prayitno, M. Rahaman, and T. R. Yudantoro, “Fake News Detection in Indonesian Popular News Portal Using Machine Learning For Visual Impairment,” JOIV Int. J. Inform. Vis., vol. 7, no. 3, pp. 726–732, Sep. 2023, doi: 10.30630/joiv.7.3.1243.
- D. Xu, S. Fan, and M. Kankanhalli, “Combating Misinformation in the Era of Generative AI Models,” in Proceedings of the 31st ACM International Conference on Multimedia, in MM ’23. New York, NY, USA: Association for Computing Machinery, Oct. 2023, pp. 9291–9298. doi: 10.1145/3581783.3612704.
- Gupta, B. B., Gaurav, A., Attar, R. W., Arya, V., Alhomoud, A., & Chui, K. T. (2024). A Sustainable W-RLG Model for Attack Detection in Healthcare IoT Systems. Sustainability, 16(8), 3103.
- Gupta, B. B., Gaurav, A., Arya, V., & Chui, K. T. (2024, June). LSTM-GRU Based Efficient Intrusion Detection in 6G-Enabled Metaverse Environments. In 2024 IEEE 25th International Symposium on a World of Wireless, Mobile and Multimedia Networks (WoWMoM) (pp. 118-123). IEEE.
Cite As
Nayuni I.E.S (2024) Detecting misinformation with AI, Insights2Techinfo, pp.1