By: Tarun Vats, Sudhakar Kumar
The Digital Twin (DT) combines all data (operation data, tests, etc.), models (engineering models, design drawings, analyses, etc.), and other information (orders, inspections, requirements.) generated during the life cycle of a physical asset to maximize economic potential. The DTs job is to predict and improve performance. Simulation and/or data-based methods are employed for this goal.
Simulations and Digital twins
Despite the fact that both employ digital models to reproduce a system’s numerous activities, a DT is a true virtual environment, making it significantly more helpful for research. The main difference is of scale: A digital twin can run as many useful simulations as it wants to look at different processes, whereas a simulation usually looks at just one.
There are a few more distinctions to make. Simulations, for example, rarely appreciate real-time data. Digital twins, on the other hand, are based on a two-way knowledge flow that starts when sensors send pertinent data to the system processor and ends when the processor’s perception are shared with the original source object [1].
The types of digital twins
There are several types of digital twins that consider the magnification of a product.
- Asset twins-A resource is defined as a collection of more than one component that work together to produce an asset. This type of twins enables study of the relationships between numerous variables, yielding a wealth of operational data that can be collected and transformed into meaningful intelligence.
- Unit (System) twins-Next degree of magnification, which allow to see how diverse assets interact to create a fully functional system.
- Parts (Component) twins-Component twins, the tiniest example of a working component, are the fundamental unit of the digital twin. Part twins deal with components that are not very important.
- Process twins- Process twins help in the discovery of precise timing schemes that affect overall effectiveness [2].
History of digital twin technology
Digital twins aren’t a brand-new concept. In fact, in the early 1960s, simulation was established as a fundamental tool for comprehending scientific phenomena (or perhaps earlier). Even though many physical processes and basic equations were understood, complicated systems like rockets and spacecraft could only be handled through simulation. Analytical approaches couldn’t solve the interactions and geometries because they were too intricate.
In reality, several of today’s widely used simulation tools, such as NASA’s Structure Analysis software, often known as NASTRAN, have their roots in NASA [3].
Applications
Digital twins are already extensively employed in subsequent applications:
- Urban planning – Digital twins assist civilian engineers and others participating in city planning operations using technologies like augmented reality.
- Healthcare services- People who receive medical facilities can be profiled using DTs. The same device generated data system can be used to monitor a number of health indicators and provide vital information.
- Power-generation equipment-large engines like power-generation turbines, train engines and jet engines get benefits from digital twins, particularly in terms of determining timelines for routine maintenance.
- Automobile manufacturing- Cars are made up of a variety of complicated, interconnected systems, and digital twins are widely utilized in the automotive industry to improve vehicle performance and increase production efficiency [4].
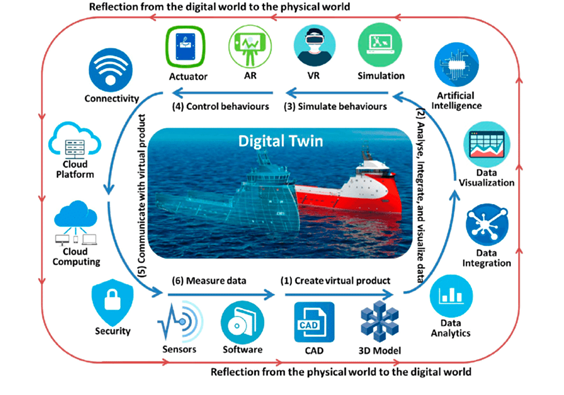
Executable Digital Twins
The execution engines of Digital Twin are wrapped in an Executable Digital Twin. They enable simulation models to be reused outside of R&D as a consequence. To do so, you must leverage existing data sets to build the Executable DT for a particular application. It must, in particular, have the necessary precision and speed. The Executable DT may be developed on the edge, on premise, or in the cloud, and can be utilized by a non-expert or a bot using a small application programming Interfaces.
Executable DTs are one critical enabler for DT democratization. Executable Digital Twins, Systems Management, and Integrated Digital Twins are just a few examples of how they might be used. [1].
Requirements
- Reliability – In precise Digital Twins need to be self-conscious and tell the consumer if they may be used outdoor their authenticate and state domain.
- Usability – As a result, from a usability standpoint, any DT solution must be accessible to non-experts as well.
- Interactivity – Accuracy and speed define the value of digital twins and simulation.
- Security -Many Digital Twin-based business models will necessitate the exchange of DT between multiple organizations. Decompile must be avoided at all costs in order to protect intellectual property.
- Synchronicity – Because each item is usually unique, Digital Twins must be customized and kept in sync with their physical counterparts.
- Deploy ability – Depending on where they were developed, such as on client premises, in the cloud, or on automation, digital twins will be used in a variety of ways. As a result, in order to reduce hurdles and effort, implementation must be straightforward [1].
Limitless upcoming opportunities
Existing operational models are certainly changing fundamentally. In a highly set-intensive business that is changing operating paradigms in a disruptive fashion, a digital remake is underway, necessitating an unsegregated material and automated picture of the equipment, assets, facilities, and processes. DTs are a critical component of this reshuffling.
Because growing amounts of cognitive capacity are continuously being allocated to their usage, the future of digital twins is practically endless. As a result, digital twins are always learning new skills and capacities, implying that they will continue to generate the insights required to improve products and processes.
References
- Hartmann, D., & van der Auweraer, H. (2020, January 3). Digital Twins. arXiv.org. Retrieved January 7, 2022,
- Glatt, M., Sinnwell, C., Yi, L., Donohoe, S., Ravani, B., & Aurich, J. C. (2021). Modeling and implementation of a digital twin of material flows based on physics simulation. Journal of Manufacturing Systems, 58, 231-245.
- Hu, W., Zhang, T., Deng, X., Liu, Z., & Tan, J. (2021). Digital twin: a state-of-the-art review of its enabling technologies, applications and challenges. Journal of Intelligent Manufacturing and Special Equipment.
- Jiang, Yuchen & Yin, Shen & Li, Kuan & Luo, Hao & Kaynak, Okyay. (2021). Industrial applications of digital twins. Philosophical Transactions of the Royal Society A: Mathematical, Physical and Engineering Sciences. 379. 20200360. 10.1098/rsta.2020.0360.
- Tao, Fei & Sui, Fangyuan & Liu, Ang & Qi, Qinglin & Zhang, Meng & Song, Boyang & Guo, Zirong & Lu, Stephen & Nee, Andrew. (2018). Digital twin-driven product design framework. International Journal of Production Research. 57. 1-19. 10.1080/00207543.2018.1443229.
Very informative article.
Amazing article
Great article for quick knowledge!
Great article