By: Yadvi Nanda1, Pushkar Kaushik1
1CSE, Chandigarh College of Engineering and Technology, Chandigarh, India
Abstract:
The rapid growth of the electric smart meter (ESM) industry, especially in smart cities, forecasts the installation of billions of smart meter bases globally by 2023. However, the complexity of the interconnected ecosystem poses challenges in efficiently managing the vast data generated. This article explores the application of advanced predictive analytics and machine learning techniques to address financial and operational challenges faced by the utility sector. The focus is on improving data collection, validation, and timely service activation in the electric smart meter domain.
I. Introduction:
In the context of smart cities[1], the electric smart meter[2] (ESM) industry is undergoing rapid expansion, especially anticipating the installation of billions of smart meter bases globally by 2023. This growth, while promising, brings forth challenges in managing the intricate ecosystem of hardware[3] and software that generates vast amounts of data. Despite the potential for innovative business models, the utility sector grapples with financial and operational hurdles in the face of advancing technologies. Key issues such as efficient data collection, validation, and timely service activation are critical. In response to these challenges, this article delves into the exploration of machine learning techniques and decision-support systems to revolutionize smart meter operations.
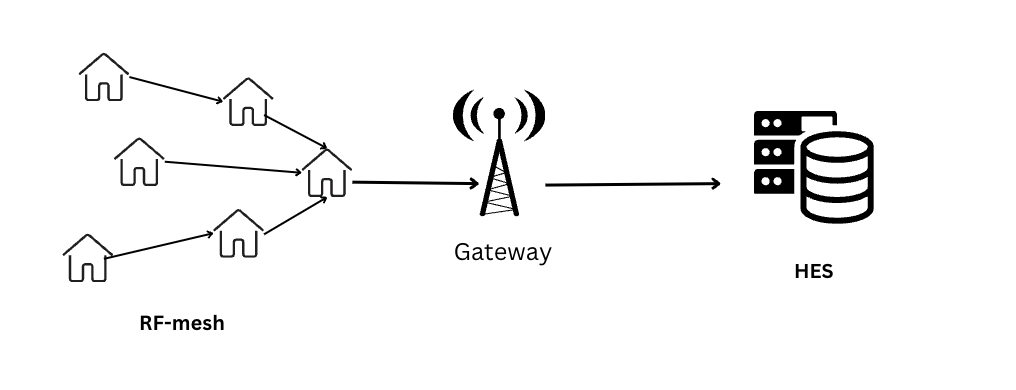
II. Current Challenges in ESM Systems Operations:
Traditional solutions for utility systems rely heavily on manual processes and standard troubleshooting methods, often identifying[4] system faults only after a client reports an issue. This reactive approach leads to delays in issue resolution and escalates operational expenses. The imperative to optimize operations and reduce costs in managing utility ESM systems necessitates a shift towards more effective and proactive solutions.
III. Proposal of a Predictive Analytics Model:
The research introduces a predictive analytics model[5], incorporating a Machine Learning Mechanism. This targets the enhancement of ESM system predictions, particularly in determining the necessity of deploying a technician[7] to address a defect. By continuously analyzing ecosystem communication quality, the mechanism presents a strategic approach to significantly reduce travel expenses during ESM network operations and maintenance.
IV. Real-world Application and Case Study:
This proposed model is not confined to theoretical frameworks; it offers a tangible solution with documented real-world applicability. A case study from a production ESM network is presented, showcasing the practicality and effectiveness of the proposed approach. Moreover, the potential benefits extend beyond electric smart meters[8], impacting other utility segments like water, gas, and heat, indicating the versatility and scalability of the proposed mechanism.
VII. Conclusion and Future Recommendations:
The article concludes by consolidating the key components of the proposed predictive analytics mechanism and presenting empirical validation. It underscores the critical importance of optimizing ESM network[13] operations and reducing travel costs, highlighting the tangible benefits of the proposed mechanism. The conclusion serves as a springboard for future research, suggesting avenues for further refinement and expansion of the mechanism’s application across diverse utility[14] segments. In summary, the integration of advanced predictive analytics[15] and machine learning holds substantial promise for enhancing the efficiency of electric smart meter operations, ultimately contributing to the betterment of the entire utility sector. The proposed model stands as a significant stride in addressing the complexities of the emerging smart ecosystem.
References
- Singh, R., Singh, S. K., Kumar, S., & Gill, S. S. (2022). SDN-Aided Edge Computing-Enabled AI for IoT and Smart Cities. SDN-Supported Edge-Cloud Interplay for Next Generation Internet of Things, 41-70.
- Siryani, J., Tanju, B., & Eveleigh, T. J. (2017). A machine learning decision-support system improves the internet of things’ smart meter operations. IEEE Internet of Things Journal, 4(4), 1056-1066.
- Kumar, S., Singh, S. K., Aggarwal, N., Gupta, B. B., Alhalabi, W., & Band, S. S. (2022). An efficient hardware supported and parallelization architecture for intelligent systems to overcome speculative overheads. International Journal of Intelligent Systems, 37(12), 11764-11790.
- Kaur, P., Singh, S. K., Singh, I., & Kumar, S. (2021, December). Exploring Convolutional Neural Network in Computer Vision-based Image Classification. In International Conference on Smart Systems and Advanced Computing (Syscom-2021).
- Mengi, G., Singh, S. K., Kumar, S., Mahto, D., & Sharma, A. (2021, September). Automated Machine Learning (AutoML): The Future of Computational Intelligence. In International Conference on Cyber Security, Privacy and Networking (pp. 309-317). Cham: Springer International Publishing.
- Singh, I., Singh, S. K., Singh, R., & Kumar, S. (2022, May). Efficient loop unrolling factor prediction algorithm using machine learning models. In 2022 3rd International Conference for Emerging Technology (INCET) (pp. 1-8). IEEE.
- SINGH, S., KARTIK, J. A. S. G., & KUMAR, S. The Role of Site Reliability Engineering in Sustainable Development. space, 2(10), 11.
- Aggarwal, K., Singh, S. K., Chopra, M., Kumar, S., & Colace, F. (2022). Deep learning in robotics for strengthening industry 4.0.: opportunities, challenges and future directions. Robotics and AI for Cybersecurity and Critical Infrastructure in Smart Cities, 1-19.
- Sharma, A., Singh, S. K., Badwal, E., Kumar, S., Gupta, B. B., Arya, V., … & Santaniello, D. (2023, January). Fuzzy Based Clustering of Consumers’ Big Data in Industrial Applications. In 2023 IEEE International Conference on Consumer Electronics (ICCE) (pp. 01-03). IEEE.
- Saini, T., Kumar, S., Vats, T., & Singh, M. (2020). Edge Computing in Cloud Computing Environment: Opportunities and Challenges. In International Conference on Smart Systems and Advanced Computing (Syscom-2021).
- Heckerman, D., Geiger, D., & Chickering, D. M. (1995). Learning Bayesian networks: The combination of knowledge and statistical data. Machine learning, 20, 197-243.
- Shi, Z., Sun, R., Lu, R., Chen, L., Chen, J., & Shen, X. S. (2015). Diverse grouping-based aggregation protocol with error detection for smart grid communications. IEEE Transactions on Smart Grid, 6(6), 2856-2868.
- Ericsson, A. B. (2015). 5g systems: Enabling industry and society transformation. In Erisson White Paper (pp. 3-14).
- Siryani, J., Mazzuchi, T., & Sarkani, S. (2015). Framework using Bayesian Belief Networks for Utility Effective Management and Operations. 2015 IEEE First International Conference on Big Data Computing Service and Applications (p. 7). San Francisco Bay, CA: IEEE.
- Misirli, A. T., & Bener, A. B. (2014). Bayesian Networks For EvidenceBased Decision-Making in Software Engineering. IEEE TRANSACTIONS ON SOFTWARE ENGINEERING, 533-554.
- Poonia, V., Goyal, M. K., Gupta, B. B., Gupta, A. K., Jha, S., & Das, J. (2021). Drought occurrence in different river basins of India and blockchain technology based framework for disaster management. Journal of Cleaner Production, 312, 127737.
- Gupta, B. B., & Sheng, Q. Z. (Eds.). (2019). Machine learning for computer and cyber security: principle, algorithms, and practices. CRC Press.
- Singh, A., & Gupta, B. B. (2022). Distributed denial-of-service (DDoS) attacks and defense mechanisms in various web-enabled computing platforms: issues, challenges, and future research directions. International Journal on Semantic Web and Information Systems (IJSWIS), 18(1), 1-43.
- Almomani, A., Alauthman, M., Shatnawi, M. T., Alweshah, M., Alrosan, A., Alomoush, W., & Gupta, B. B. (2022). Phishing website detection with semantic features based on machine learning classifiers: a comparative study. International Journal on Semantic Web and Information Systems (IJSWIS), 18(1), 1-24.
Cite As
Nanda Y., Kaushik P. (2024) Enhancing Smart Meter Operations Through Advanced Predictive Analytics, Insights2Techinfo, pp.1