By: Himanshu Tiwari, International Center for AI and Cyber Security Research and Innovations (CCRI), Asia University, Taiwan, nomails1337@gmail.com
In the era of virtual streaming, movie streaming platforms have revolutionized the way we consume content. With hundreds of thousands of users Globally, these platforms continuously try to offer better content material guidelines and personalization to decorate personal delight. However, this aim comes with widespread privacy and data security worries. To deal with this, federated Learning has emerged as a powerful solution, permitting film streaming systems to improve recommendation algorithms even while keeping consumer privacy.
What is Federated Learning?
Federated Learning is a Platform knowledge approach that permits version training throughout decentralized area gadgets or servers at the same time as keeping statistics localized. It permits algorithms to be trained on the information of individual customers without sharing their non-public data with an important server. This technology is a game-changer for film streaming structures looking to stabilize personalized content guidelines with stringent privacy policies[1].
Benefits of Federated Learning in Movie Streaming
- Privacy Preservation:
- One of the primary benefits of federated Learning is its potential to protect personal facts. Withtraditional recommendation structures, consumer information is regularly gathered and stored centrally, raising concerns about statistics breaches and misuse. Federated Learning guarantees that user records stay on the user’s device and best-aggregated version updates are shared. This approach respects customers’ privacy and complies with data protection rules.
- Enhanced Personalization:
- Movie streaming systems intend to offer customers particularly personalized content material hints.Federated Learning empowers those systems to create greater correct and tailored advice fashions without compromising user privacy[2]. By analyzing user interactions domestically, after aggregating those insights, platforms can offer content that better suits personal choices
- Data Efficiency:
- Federated Learning often requires huge quantities of records to make correct predictions. Federated Learning allows platforms to leverage records from a wide range of users while not having to switch them to an important server[2]. This consequences in greater green version training and higher hints, even for users with constrained statistics.
- Reduced Latency:
- With federated Learning, model updates happen regionally, reducing the latency related to transferring data to a significant server[2][1]. Users enjoy faster and greater responsive content material hints, improving their typical streaming experience.
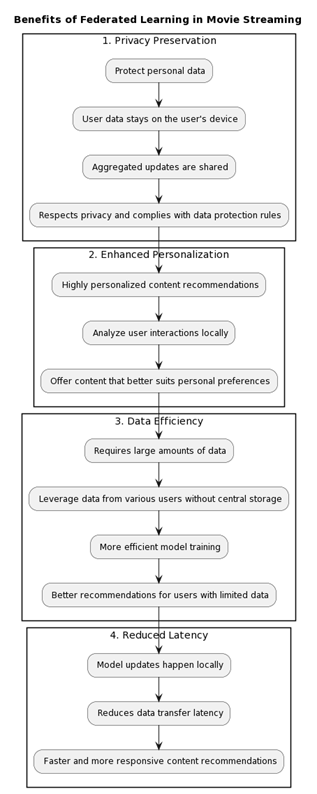
Challenges and Considerations
While federated Learning offers numerous benefits for movie streaming systems, it is not without its challenges[3]:
- Model Aggregation:
- Aggregating version updates from various user gadgets may be complicated. Algorithms should be designed to make sure that the global model as it should be represents consumer alternatives while maintaining individual consumer privacy[3][4].
- Communication Overhead:
- Federated Learning calls for communique among gadgets or servers to share version updates. Efficient conversation protocols are crucial to decrease the overhead and bandwidth necessities.
- Security:
- Federated Learning techniques can be susceptible to attacks, which include model inversion or membership inference. Strong safety features and sturdy encryption protocols are vital to protect the federated mastering technique.
- User Participation:
- For federated Learning to be effective, users want to choose and participate willingly. Educating users about the advantages of this era and obtaining their consent is critical[4].
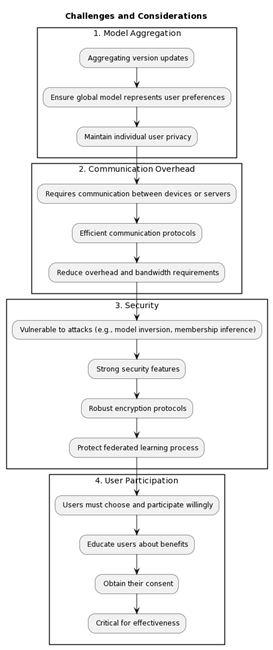
Conclusion
Federated learning has the potential to revolutionize film streaming platforms by improving person enjoy and information privacy. By leveraging this era, platforms can provide greater accurate and customized content material guidelines while respecting the privacy of their customers. As the digital landscape continues to conform, federated Learning is likely to become an essential part of the streaming industry, ensuring secure and satisfying streaming enjoyment for all users. As the era advances, we are able to assume even more upgrades in both personalization and privacy on movie streaming platforms.
Reference
- Zhang C, Xie Y, Bai H, Yu B, Li W, Gao Y. A survey on federated learning. Knowledge-Based Systems. 2021 Mar 15;216:106775.
- Neumann D, Lutz A, Müller K, Samek W. A Privacy Preserving System for Movie Recommendations using Federated Learning. arXiv preprint arXiv:2303.04689. 2023 Mar 7.
- Yu Z, Hu J, Min G, Lu H, Zhao Z, Wang H, Georgalas N. Federated learning based proactive content caching in edge computing. In2018 IEEE Global Communications Conference (GLOBECOM) 2018 Dec 9 (pp. 1-6). IEEE.
- Liu M, Ho S, Wang M, Gao L, Jin Y, Zhang H. Federated learning meets natural language processing: A survey. arXiv preprint arXiv:2107.12603. 2021 Jul 27.
- Casillo, M., Colace, F., Gupta, B. B., Santaniello, D., & Valentino, C. (2021). Fake news detection using LDA topic modelling and K-nearest neighbor classifier. In Computational Data and Social Networks: 10th International Conference, CSoNet 2021, Virtual Event, November 15–17, 2021, Proceedings 10 (pp. 330-339). Springer International Publishing. https://link.springer.com/chapter/10.1007/978-3-030-91434-9_29
- Gupta, B. B., & Sahoo, S. R. (2021). Online social networks security: principles, algorithm, applications, and perspectives. CRC Press.
- Yadav, K., Gupta, B. B., Chui, K. T., & Psannis, K. (2020). Differential privacy approach to solve gradient leakage attack in a federated machine learning environment. In Computational Data and Social Networks: 9th International Conference, CSoNet 2020, Dallas, TX, USA, December 11–13, 2020, Proceedings 9 (pp. 378-385). Springer International Publishing.
- Srivastava, D., Chui, K. T., Arya, V., Peñalvo, F. J. G., Kumar, P., & Singh, A. K. (2022). Analysis of Protein Structure for Drug Repurposing Using Computational Intelligence and ML Algorithm. International Journal of Software Science and Computational Intelligence (IJSSCI), 14(1), 1-11.
Cite As:
Tiwari H. (2023) Enhancing User Experience and Data Privacy with Federated Learning in Movie Streaming Platforms, Insights2Techinfo, pp.1