By: K. Sai Spoorthi, Department of computer science and engineering, Student of computer science and engineering, Madanapalle Institute of Technology and Science, 517325, Angallu, Andhra Pradesh.
Abstract
The contents generative artificial intelligence of text, image and displayed sound is one of the most wonderful inventions of the technology. However, it has experienced a rapid increase in its application in environments in which questions of equity and prejudice are vital ethical concerns. In this research, the given consideration is made to the causes of bias in generative AI and their origins from the training data up the model designs [1]. They state that these biases should be countered applying to the following approaches, data sources’ diversity, options’ explainability, and stakeholders’ engagement. The paper thus recommends ways of ethical and social justice intuitions in the AI and course of action toward the construction of equipoise AI. Thus, the results stress the theoretical and practical importance of combating both technical and social biases simultaneously and creating interaction and collaboration between domains to adapt the justice and equity-oriented AI systems.
Key words: Generative Artificial Intelligence, Bias, Algorithmic transparency, Ethical AI, Fairness, Data diversification
Introduction
Computerization in the present age is advancing in a crescendo, and consequently, the generative AI has disrupted different areas such as generation, data synthesis and decision-making. The nature of these algorithms brings about unprecedented efficiency and design, but at the same time, they bring incredible ethical concerns among them being, bias and fairness. While algorithmic systems spend careful moments looking at big data, they draw patterns on the data sets’ social realities and historical discrimination of minorities thus eliciting critical questions on its impact on Those left behind. Solving these biases is not a simply a scientific issue; it requires an ethical and social justice approach, while also involving the communities for the effective discourse on the use of the generative AI technologies. This paper aims at analysing the balance between the formalization of AI processes and the nonspecialist’s values, pointing to the need to design fair AI systems with the goal to avoid sacrificing justice for technological advancement.
Generative AI and its significance in modern technology
They declared that the arrival Generative AI is one of the significant jumps in progress as it is an endeavour to create machines that can generate content in categories of a text, image, or sound like how the human brain does. It has led to the emergence of new level of efficiency and innovation in the many fields and essential changes in terms of approach to informatics and integration of certain functions. Nevertheless, incorporation of these innovative tools has not came without these problems touching on general ethical questions and concession on aspect of bias. For instance, questions have been raised on the level of academic integrity given that students are now using generative AI chatbots to do homework, as shown in fig 1. Other problems that are still present are the ethical, regulatory and privacy problems which prevent the officers from emulating use of these technologies hence the low organizational trust in these technologies . However, as society progressing in trying to comprehend the effects of Generative AI it is high time for these questions to be answered in order to enhance the performance and reduce the adverse effects caused by the abuses of this technology.
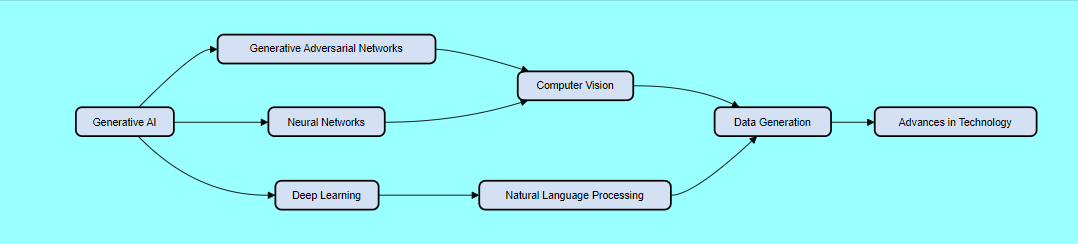
Understanding Bias in Generative AI
Hence, the bias of generative AI systems is many sided being involved in several aspects that begin from the choice of the data to be used for training the model to the use of the right kind of algorithms. Training datasets are mainly historical, thus, the AI models memorize the prejudices of societies and embed them into their products. This is quite apparent especially in language models where the omission of some groups can result in formation of languages leaning towards that stereotype and inaccuracy. Also, some algorithms are inclined to demonstrate some certain result by their nature, and for definite opinion or including definite kind of people it is unfair[2]. Therefore, optimisation of these biases is a complex understanding of AI as the means of creating it and as the sociocultural object. Also, with all the different stakeholders involved in the formulation of the AI systems, one is assured of enhancing the generative AI systems that are fair and in equal proportion to the population of the world hence enhancing on the equity bit regarding the advancements in such forms of AI systems.
Types of biases present in generative models and their sources
Sources of bias in generative models are numerous, which means that particular attention should be paid to them in order to achieve the best results and to avoid demonstrable prejudice within the results. One of them is the training data that may contain society’s bias and discriminations. If the data possesses ethnic or gender bias, or any such category which has been discriminated in the past, the same bias shall be reflected in the model resulting in injustice. Also, it is possible to distinguish bias which is inherent in the model architecture and design choices in which some of the parameters will inevitably act in favour of certain outcomes. These technical decisions when paired with the nature of the data come with a set of new challenges and entwine with it in a vicious cycle that tends to either magnify or reproduce the bias within the system and produce skewed or harmful results. These interrelated prejudices present a more complex problem of dealing with the data along with the general understanding of the algorithms used to analyze the information, which in turn encourages researchers and practitioners alike to participate in bias reduction activities throughout the process. Lastly, conscientiousness can create better equality for future generative systems for people of different backgrounds and bring more people into the development of AI solutions.
Strategies for Mitigating Bias and Ensuring Fairness
This way, it becomes more ‘natural’ to arrive at a decision on who should be arrested next, or who should be fired next, and so on, and so forth; to put it bluntly, the results of bias in generative AI systems are devastating In light of this, getting rid of bias in generative AI systems requires a multi-faceted approach. First of all, the issue of data diversification is important when it comes to teaching models that reflect the society in all its nature. A strong consideration of and inclusion of many types of demographic variables in the datasets can greatly reduce cases of overrepresentation and underrepresentation. Further, algorithmic transparency enhances the level of responsibility; it enables the developers to distinguish how decisions taken within these systems are arrived at. [1]Frequency checks and more especially the bias audits can regularly point out other hidden biases that may require correction. Moreover, the incorporation of diverse personnel during the development process and the participation of different stakeholders offering various opinions, tend to create better and more equal AI models. Frequent training also on bias detection on the part of the developers, users and policy makers will capability them to be on the lookout for fairness failures and address them in an early stage. Lastly, integrating of these strategies creates a less prejudiced utilization of the generative AI technologies.
Techniques and frameworks for developing fair generative AI Systems
While striving to solve the problem of a reasonable generative AI design and creating fair AI systems, one should use more efficient extensive methods and evaluate the implementation of the principles of minimalism and how they reflect the values of society in exhaustive approaches. One is the incorporation of the fairness-aware algorithms in the model training phase since they help to minimize bias that occurs in sets used for training. For example, improving the interpretability of generative AI results helps users, especially the CDSCs in retail, to make reasonable decisions based on a model’s predictions while avoiding biased content generation.[3] Also, if more stakeholders are involved at the design level, such that other facets of the bias can be identified and mitigation strategies developed to reduce their negative impacts on AI systems. Since the same issues are currently relevant to the higher education sector in terms of academic integrity while applying such tools, it is crucial to establish the policies regarding the more ethical integration of the generative AI.[4]
Conclusion
The presented investigation of bias and fairness in generative AI identifies that there is a significant field of high concern that not only demands investigation but also interaction. Based on the evidence presented in this essay, therefore, we are left with two choices: the futurist which brings with it the potential of this field’s advancement by developing generative AI and the purist that resets known prejudices, strengthens the advancement of such prejudices, produces doubts that beg ethical consideration, and perturbs conscience that cannot be ignored. Thus, it is necessary to note that the issue with bias contamination in the systems that include AI is actual as a data selection level bias, but also as the bias on the level of algorithms’ designing, which means that it should be solved on the systemic level. [5]This entails guaranteeing that there is rigid standardize with appropriate monitoring and supervision in the utilization and development of artificial intelligence, guaranteeing that there are civil ways through which AI can be developed and used, and encouraging various forms of people’s participation in technology. Moving to the future, it is necessary to keep equality apart from spinning and adding preconditions as one of the vital components of the creation of AI systems. The gestures described here are offered as laid-in move towards starting the process of building towards that vision of a fair technological regime, and as a plea for more work across humanist and other fields to generate more justice for the AI.
The findings of this study suggest that bias in Generative AI platforms is not just technical it is social since AI is society’s justice system. The main findings that have been demonstrated in different applications from the case studies that have been presented are that whenever bias is added by the training data, the results are undesirable. Thus, this work establishes a foundation for the development of the cross-disciplinary efforts involving both E-Ts and SIS for evolving the AI solutions. Further research in the future needs to on the development of other frameworks that conforms with the principles of accountability as well as the transparency so that exclusionary bias is not only militated against but also to optimize on the results.
References
- E. Ferrara, “Fairness and Bias in Artificial Intelligence: A Brief Survey of Sources, Impacts, and Mitigation Strategies,” Sci, vol. 6, no. 1, Art. no. 1, Mar. 2024, doi: 10.3390/sci6010003.
- C.-Y. Lin, M. Rahaman, M. Moslehpour, S. Chattopadhyay, and V. Arya, “Web Semantic-Based MOOP Algorithm for Facilitating Allocation Problems in the Supply Chain Domain,” Int J Semant Web Inf Syst, vol. 19, no. 1, pp. 1–23, Sep. 2023, doi: 10.4018/IJSWIS.330250.
- S. Venkatasubbu and G. Krishnamoorthy, “Ethical Considerations in AI Addressing Bias and Fairness in Machine Learning Models,” J. Knowl. Learn. Sci. Technol. ISSN 2959-6386 Online, vol. 1, no. 1, Art. no. 1, Sep. 2022, doi: 10.60087/jklst.vol1.n1.p138.
- B. D. Alfia, A. Asroni, S. Riyadi, and M. Rahaman, “Development of Desktop-Based Employee Payroll: A Case Study on PT. Bio Pilar Utama,” Emerg. Inf. Sci. Technol., vol. 4, no. 2, Art. no. 2, Dec. 2023, doi: 10.18196/eist.v4i2.20732.
- A. Rajabi and O. O. Garibay, “Towards Fairness in AI: Addressing Bias in Data Using GANs,” in HCI International 2021 – Late Breaking Papers: Multimodality, eXtended Reality, and Artificial Intelligence, C. Stephanidis, M. Kurosu, J. Y. C. Chen, G. Fragomeni, N. Streitz, S. Konomi, H. Degen, and S. Ntoa, Eds., Cham: Springer International Publishing, 2021, pp. 509–518. doi: 10.1007/978-3-030-90963-5_39.
- Raj, B., Gupta, B. B., Yamaguchi, S., & Gill, S. S. (Eds.). (2023). AI for big data-based engineering applications from security perspectives. CRC Press.
- Gupta, G. P., Tripathi, R., Gupta, B. B., & Chui, K. T. (Eds.). (2023). Big data analytics in fog-enabled IoT networks: Towards a privacy and security perspective. CRC Press.
- Chaudhary, P., Gupta, B. B., & Singh, A. K. (2022). XSS Armor: Constructing XSS defensive framework for preserving big data privacy in internet-of-things (IoT) networks. Journal of Circuits, Systems and Computers, 31(13), 2250222.
Cite As
Spoorthi K.S. (2024) Generative AI: Addressing Bias and Fairness, Insights2Techinfo, pp.1