By: K. Sai Spoorthi, Department of computer science and engineering, Student of computer science and engineering, Madanapalle Institute of Technology and Science, 517325, Angallu, Andhra Pradesh.
Abstract
AI in its generative form is bringing new solutions to the field of individualized medicine, especially in the ways of handling data and drug discovery and processing genomic data. This paper aims to describe the current and potential impact of generative AI for increasing the efficacy and effectiveness of patient/molecular individualized therapy, the latter’s benefits on patients, and the possibility of better use of healthcare organization resources. In this way, generative AI develops individual treatment plans, considering patient’s DNAs, lifestyles, environments, etc. AI integration in drug discovery enhances the rate at which new compounds are found, the general lead optimization, and compile time and costs. Also, predictive modelling and simulations with an AI generator also help in forecasting drug interactions and efficacy for the continued growth of tailored medicine. Also, the area of genomics is presented in this paper, which indicates the use of artificial intelligence in the interpretation of large biological data and in the making of decisions within healthcare. Looking to the generative AI’s future in personalized medicine, it appears that further advancements and developments of new healthcare solutions are on the horizon for technology, healthcare and the ethical field.
Key words: Generative Artificial Intelligence, precision medicine, GAN, VAE,
Introduction
With an enhanced development rate in recent years, generative AI emerged as one of the strategic change agents in the field of healthcare especially with regard to the field of personalized medicine. As a result, the belief that it is impossible to produce targeted treatments for patients also shifted to the approach of generative AI from the traditional medicine. It also exponentially improves efficiencies throughout the production process. Out of the paradigm shifts, this one has the capacity of improving clinical results to the highest degree, patients’ satisfaction and better utilization of resources within the health institutions.[1] To examine the relationship between generative AI and personalised medicine more closely it is necessary to look at, on the one hand, the technology enablement and on the other hand at the ethical ramifications of such an approach. Familiarizing with these dynamics will offer a conceptual foundation for evaluating how the advent of generative AI will change the possibilities of the individualized model of healthcare and, therefore, enhance the efficiency of interventions.
Generative AI and its relevance to personalized medicine
The advent of Generative AI constitutes a turn in the innovative science of pharmacogenomics as it provides unparalleled opportunity for data processing and analysis in the work of teachers and scholars. In this way, Generative AI can create profound algorithms enriched with big data sets as analytical tools to design prescription treatments based on patient profiles. This level of personalization goes even beyond the previous levels by considering thousands of parameters, DNA, lifestyle, and the environment that make a patient unique, which improves the accuracy of therapy choices greatly. Additionally, Generative AI’s capability to generate new chemical compounds and engineer ideal pharmaceutical agents proves its suitability to enhance drug discovery procedures, comprehending the specific aspects of population heterogeneity. [2]As a result, using Generative AI for the creation of efficient models proves to be appropriate. personalized medicine not only contributes to acute enhanced results in the treatment and approach of patients but also accredit with the general goals of the healthcare system regarding optimization of the work and costs.
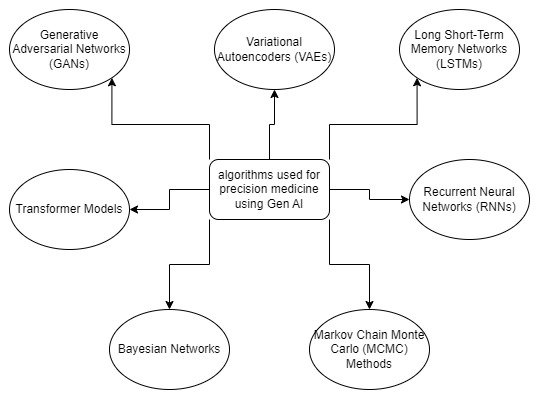
Applications of Generative AI in Drug Discovery
The progress in the generative AI is already changing the context of drug development and furthering the goals of modern medicine by unmasks the whole new of opportunities for convenient drug production. accuracy and speed of the methods aimed at the discovery of new substances. Picking up from where we left off, the paper went on to uncover how the use of deep learning algorithms can be harnessed. more accurate prediction of molecular properties and interaction, lead optimization is easier for the researchers. Automatic generation of chemical compounds can be produced with the help of generative models that approach reference targets and render the applied biological exertions drastically more efficient than trial-and-error approaches about time and costs. In addition to this, the approaches involve the use of artificial intelligence thus enabling searches of the enormous chemical space with the possibility of identifying compounds that could otherwise go unnoticed. This not only increases the frequency of additions of new innovations but also increases the probabilities of attaining efficient treatment regimens for complicated diseases, thus leading to more science-based personalized medicine solutions. Specifically in the field of personalised medicine, Gen AI has become so indispensable with the help of complex algorithms such as GAN and VAE.[3] These modes which are complex adopt any challenge and provide a solution like data scarcity, privacy issues, and the complexities of modelling complex human health data.[4] Beneficial connotations with AI and drug discovery based on how generative AI enhances one another makes future initiatives for progress and subsequent investment and research in this area relevant.
Enhancing drug design through predictive modelling and simulation
Progresses in the field of, for instance, predictive modelling and simulation offer new opportunities in drug design with a perspective of radical change. offer researchers instruments that help to improve the outcomes and productivity of the development process. By thus, using such generative AI models in their work, scientists can recreate many interactions in the biological environment and make quite accurate forecasts. how individual elements are going to react once they are incorporated with other chemical entities in the human body. This capability does not only put the speeds up but also enables the identification of potential drug compounds but also helps in the development of these drugs before the latter even being trialled in the clinic. However, when employing an external ML model in the process of drug design, the following advantages are additionally gained: enables end-users—those citizens who use their respective fields of specialty to analyse arrays of data deal with massive data —improving decision makers’ skills .As the as the overall picture of personalized medicine expands, the value of such modern approaches emerges more clearly than before, placing them right at the heart as key building blocks of the search for higher degrees of customization to provide information about treatment that focuses on the patient.
Generative AI in Genomic Data Analysis
Introducing the generative AI in the genomic data analysis is a revolutionary achievement in terms of the task performed by the researchers. interpret complex biological information. Due to the availability of sophisticated algorithms, generative AI can create massive data sets, model genetic variations and find out hidden pattern on the Genomic sequences. This capability does not only increase the level of speed in terms of research but also increase the level of accuracy when it comes to genomic analysis.[5] this makes it more suitable to apply in drug treatment and customization of therapies in the newly developing area of personalized medicine. For example, generative models can mimic the results of different genomic modifications on phenotypic consequences and to make more profound prognosis. disease processes and possible treatment outcomes depends upon the characteristics of the disease. Furthermore, as the AI systems minimise on complexity, as might be expected, due to their versatility in coping with multiple and complex forms of genomic information, they stand to play a huge role in decision-making. analytics and risk assessment, the predetermining of decision-making in the clinical field and thus, enhancing patient specifications. This evolution shows how technology Those who have been following this website will appreciate this evolution because it shows the important intersection of technology and biology to create a map for new and different ways of making treatment available to the patient.[6]a
Conclusion and Future of Generative AI in advancing personalized medicine
The incorporation of generative AI in the construction of precision medicine is a revolutionary improvement in the sphere of medicine that is worthy of discussion. It supports the development of specific interventions aimed at improving a patient’s results. With the merging of inexorable progress of technology with medicine, applications for the use of generative AI to analyse and combine humongous databases and give the evidence for client-centred approach refers to the fact that predictions as well as the nature of the interventions which are appropriate for each client becomes very clear when one is in pursuit of this degree. As an outcome, decision-making becomes contingent on the forecasted probability of an intervention being beneficial, and the likelihood of risk, machine learning algorithms help healthcare providers make better decisions by not only predicting efficacy but also the potential side effects. In addition, there are questions related to the ethical issues, such as data privacy and bias; the stakeholders should establish the proper rules that will protect and regulate the application of AI in the health sector. [7] Personalized medicine’s future is contingent on bringing together experts from technology, clinical practice, and ethical concerns for the enhancement of these instruments to create a brand-new paradigm of health care that is groundbreaking and good.
References:
- K. J. Prabhod, “Leveraging Generative AI and Foundation Models for Personalized Healthcare: Predictive Analytics and Custom Treatment Plans Using Deep Learning Algorithms,” J. AI Healthc. Med., vol. 4, no. 1, Art. no. 1, Mar. 2024.
- G. P. Patrinos, N. Sarhangi, B. Sarrami, N. Khodayari, B. Larijani, and M. Hasanzad, “Using ChatGPT to predict the future of personalized medicine,” Pharmacogenomics J., vol. 23, no. 6, pp. 178–184, Nov. 2023, doi: 10.1038/s41397-023-00316-9.
- M. Rahaman et al., “Utilizing Random Forest Algorithm for Sentiment Prediction Based on Twitter Data,” 2022, pp. 446–456. doi: 10.2991/978-94-6463-084-8_37.
- I. Ghebrehiwet, N. Zaki, R. Damseh, and M. S. Mohamad, “Revolutionizing personalized medicine with generative AI: a systematic review,” Artif. Intell. Rev., vol. 57, no. 5, p. 128, Apr. 2024, doi: 10.1007/s10462-024-10768-5.
- B. Yelmen et al., “Creating artificial human genomes using generative neural networks,” PLOS Genet., vol. 17, no. 2, p. e1009303, Feb. 2021, doi: 10.1371/journal.pgen.1009303.
- L. Triyono, R. Gernowo, P. Prayitno, M. Rahaman, and T. R. Yudantoro, “Fake News Detection in Indonesian Popular News Portal Using Machine Learning For Visual Impairment,” JOIV Int. J. Inform. Vis., vol. 7, no. 3, pp. 726–732, Sep. 2023, doi: 10.30630/joiv.7.3.1243.
- M. Almansour and F. M. Alfhaid, “Generative artificial intelligence and the personalization of health professional education: A narrative review,” Medicine (Baltimore), vol. 103, no. 31, p. e38955, Aug. 2024, doi: 10.1097/MD.0000000000038955.
- Sarin, S., Singh, S. K., Kumar, S., Goyal, S., Gupta, B. B., Arya, V., & Chui, K. T. (2024). SEIR‐driven semantic integration framework: Internet of Things‐enhanced epidemiological surveillance in COVID‐19 outbreaks using recurrent neural networks. IET Cyber‐Physical Systems: Theory & Applications.
- Gupta, B. B., Gaurav, A., Attar, R. W., Arya, V., Alhomoud, A., & Chui, K. T. (2024). A Sustainable W-RLG Model for Attack Detection in Healthcare IoT Systems. Sustainability, 16(8), 3103.
Cite As
Spoorthi K.S. (2024) Generative AI in Personalized Medicine, Insights2Techinfo, pp.1