By: K. Sai Spoorthi, Department of computer science and engineering, Student of computer science and engineering, Madanapalle Institute of Technology and Science, 517325, Angallu, Andhra Pradesh.
Abstract
High-technology rate introduces a new paradigm shift in the scientific field where Generative AI was one of the most promising resources in the increase of scientific discovery. This paper intends to identify the role of Generative AI in various fields of science; ability of a Generative AI system to handle large chunks of data; planning experiments and estimating the outcomes of an experiment are some of the features of Generative AI. This paper discusses the rationale and use of Generative AI CCF: Sample application scenarios familiar from fields such as drug development or climatology have described how Generative AI can be employed and will create benefits such as increased efficiency and merging of domains. However, along with the integration of Generative AI comes ethic issues, the ethic of data and knowledge, ethic of owning Generative AI as well as ethic of using Generative AI in research studies. According to the following challenges, it is crucial to overcome these obstacles to make Generative AI helpful and unique in the scientific setting. It is most appropriate to use ethical consideration to steer or guide the process of conducting scientific research using Generative AI The working must be interdisciplinary to fully optimise its use in scientific research.[1]
Key words: Generative Artificial Intelligence, data analysis, scientific research, drug discovery, climatology, predictive modelling
Introduction
The enhancement of technologies was leading to the new age science with Generative AI as a crucial aspect to the next level research. This invaluable instrument is powerful enough to work with the large-scale data and sophisticated calculations to create new hypotheses, to simplify the layouts of the experiments and even forecast results with higher level of success. Thus, generative AI comes into focus as a critical partner to help conduct research and streamline the analysis of the overwhelming amount of information, at the same time increasing the accuracy of conclusions. This essay shall discuss on how generative AI can be used in several fields of science, with the advantages as well as the drawbacks of generating AI. Lastly, it targets at understanding how the incorporation of these modern computational tools into conventional research approaches can lead to new innovations to change the future of cross-disciplinary research.
Generative AI and its relevance to scientific research
Originally, the aspects of scientific investigations have received notable improvements during the past few years with the help of generative AI technologies. Sophisticated ML techniques offer the opportunity to compress the task of interpreting big data, as well as searching for patterns and therefore generating hypothesizes at a much faster pace. Such capability is most useful in disciplines such as drug discovery where generative models may extend the new molecular design possibilities of the possible therapeutic agents and can afford considerable amount of time in research and development. Also, generative AI contributes to applying various systems and processes from the standpoint of simulation and perceiving the effects of such actions from the computational perspective. Therefore, the introduction of generative AI into the scientific processes has increased not only the efficacy of discovery but also the overall access to powerful analytical tools in the hands of subsequent researchers with different expertise to perform new assignments and enlarge the scientist’s society’s cumulative base of existing knowledge. The generative AI has emerged as one of the most important trends in the focus areas of modern science and technology in the recent past as it assists scholars at the stage of hypothesis development, data analysis, or result reproduction. Their and approach of managing large data to derive correct conclusions has added the practice of precise estimations to domains that earlier could not define the correlation within aspects that comprise climatology or epidemiology. It could be recalled that such models needed an iterative learning process, which makes testing and adjustments faster, thus cutting on time and other expenses typical in research and development. Hence adopting generative AI is not only a way of increasing speed at which a particular scientific discipline evolves but also collaboratively since now knowledge and approaches available in AI can be passed through walls and can be easily shared among the scientific society without restrictions.
Case studies showcasing successful implementations in various scientific fields
As a part of exploring success stories of generative AI across multiple scientific fields, the study appreciable examples of the application of these concepts. For instance, scientists who work in drug discovery have used generative models to forecast molecular geometries involved in drug discovery which is far much faster than the conventional practices. Another study reported that candidates emerging from the AI-driven algorithms led to a 30% increase in the successful drug development, as shown in fig1, in the drug discovery pipeline. Also, in the discipline of climatology generative AI has been instrumental in enhancing complex prognosis for weather patterns and the resultant projections are accurate. It has not only helped in disaster preparedness but also contributed to advising the policymakers amply for creating prevent mechanisms [2].Altogether, these examples contribute to highlighting the prospective and capacities of generative AI and revealing that it can be efficiently used for solving different challenging scientific problems and for inspiring the interdisciplinary collaboration.
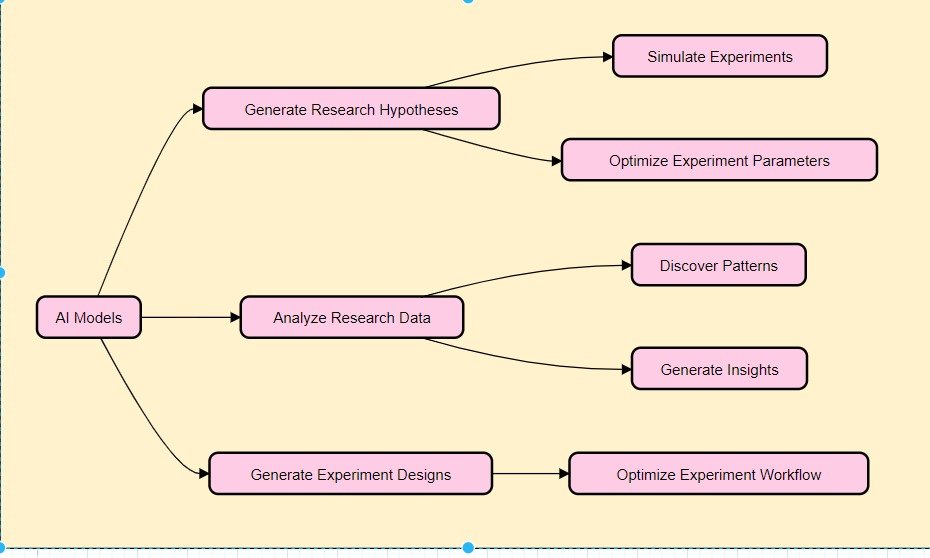
Ethical Considerations and Challenges
It is hence unreasonable to categorize the questions of ethicality regarding the application of generative AI within scientific research as insignificant since there are numerous questions of ethicality pertinent to this matter of importance. Regarding organization front, one concern in this direction that can be viewed is that the algorithms that underlie the platforms are not a priori and can remain as the subject of many biases that are likely to distort the range of scientific research. This bias may be credited to the sets of data used in training these models that probably reflect the societal prejudices or System imperfections and hence come up with such findings that are in the research A case can be made that research Labs are not exempted from such biased models. [3]Nevertheless, it is crucial for the definition to speak about the facet of intellectual property; as AI is increasingly incorporated into knowledge creation, the problem of who is the author and who is the owner? These challenges require correct ethical measures that should regulate interactions in generative AI to ensure that the drive for further investigations does not overshadow the conflict over ethical principles in scientific research.
Examination of ethical dilemmas and potential biases in generative AI applications
The integration of generative AI in scientific research entails several ethical and biases issues worthy of consideration. Along with these technologies however come matters concerning data compliance and production of fuzzy data that result in the setting of the policies and standards. For example, risk guidelines from different countries aim at managing risks; yet, mainly, responsibility assignment is in the primary AI service providers’ hands rather than users, and one of the disseminations channels This limitation is ethically questionable and pertinent to the impact of the science and society partnership because the relation between generated outputs and the input data may be affected by bias. However, the shared requirement to link the innovative imperative with moral objectives shows that it is crucial to refine the management models based on transparency and collaboration within the framework of the research space. At the same time, the solutions to these dilemmas are inevitable if generative AI is to be employed not as a guise for deception but as a form of leveraging resource for innovation.
Summary of findings and future
Thus, it can be noted because of scientific works that the use of generative AI has in approaching phenomenal discoveries, thereby proving that a change in the approach used in science is possible. Some advances have been made like the rate at which through predictive modelling, compounds for the development of new drugs can be identified. Besides, speaking about the reforms in the field of data analytics and analyses, it is necessary to mention generative AI, through which data and analyses have improved, providing researchers with an opportunity to find some relations in big data that may be unnoticed in any other way.[4] Thus, today, necessary conditions for scientists’ cooperation have appeared to be more active, with AI as an effective stimulator for research.[5] Therefore, for the further development of the generative AI personnel, attention to the ethical component, as well as the definition of the problems regarding data credibility and the replicability of research outcomes when using generative AI should be addressed. Besides, to optimize the dependability of the results, it is crucial to combine individuals’ skills with artificial intelligence. [6]Explaining the mentioned aspects in detail will disclose the further evolution of the generative AI concept and also reflect the general evolution directions towards reaching the innovations and scientific advancements.
Conclusion
When integrating the benefits and issues highlighted regarding the utilisation of generative AI in the scientific community as well as the discovery process embraced by science, one can appreciate the fact that the former entails considerable potential that needs to be properly managed. The executives of generative models may revolutionize the methodologies due to the possibility of fast material exploration and improved predictive maintenance, especially in the field of battery technology. However, as the gizmos of the concept widen, the drawbacks that are inherent in data deficiency and composite questions of ethics cannot be neglected. This is even more so the case when one considers GDA data augmentation, a method that still has available benchmark and theoretical support to aid in closing data gaps. To construct such a future, effective collaborations of scholars from different disciplines and concentrated attempts to build strong cyberinfrastructures will prove essential. Finally, the future of generative AI in scientific discovery depends less on the technology and more on how it is managed to be responsible in attaining ethical norms while analysing big data.
References
- D. Leslie, “Does the sun rise for ChatGPT? Scientific discovery in the age of generative AI,” AI Ethics, Jul. 2023, doi: 10.1007/s43681-023-00315-3.
- H. Wang et al., “Scientific discovery in the age of artificial intelligence,” Nature, vol. 620, no. 7972, pp. 47–60, Aug. 2023, doi: 10.1038/s41586-023-06221-2.
- M. Al-kfairy, D. Mustafa, N. Kshetri, M. Insiew, and O. Alfandi, “Ethical Challenges and Solutions of Generative AI: An Interdisciplinary Perspective,” Informatics, vol. 11, no. 3, Art. no. 3, Sep. 2024, doi: 10.3390/informatics11030058.
- R. Lopez, A. Gayoso, and N. Yosef, “Enhancing scientific discoveries in molecular biology with deep generative models,” Mol. Syst. Biol., vol. 16, no. 9, p. e9198, Sep. 2020, doi: 10.15252/msb.20199198.
- L. Triyono, R. Gernowo, P. Prayitno, M. Rahaman, and T. R. Yudantoro, “Fake News Detection in Indonesian Popular News Portal Using Machine Learning For Visual Impairment,” JOIV Int. J. Inform. Vis., vol. 7, no. 3, pp. 726–732, Sep. 2023, doi: 10.30630/joiv.7.3.1243.
- M. Rahaman et al., “Utilizing Random Forest Algorithm for Sentiment Prediction Based on Twitter Data,” 2022, pp. 446–456. doi: 10.2991/978-94-6463-084-8_37.
- Mamta, & Gupta, B. B. (2020). An efficient KP design framework of attribute‐based searchable encryption for user level revocation in cloud. Concurrency and Computation: Practice and Experience, 32(18), e5291.
- Gupta, B. B., & Srinivasagopalan, S. (Eds.). (2020). Handbook of research on intrusion detection systems. IGI Global.
Cite As
Spoorthi K.S. (2024) Generative AI in Scientific Research and Discovery, Insights2Techinfo, pp.1