By: Indu Eswar Shivani Nayuni, Department Of Computer Science & Engineering(Data Science), Student Of Computer Science & Engineering(Data Science) ,Madanapalle Institute of Technology and Science, Angallu(517325),Andhra Pradesh . indunayuni1607@gmail.com
Abstract
The use of generative AI models as highly innovative solutions for fake news detection employing their ability to generate and analyze data. They were introduced to provide better accuracy and effectiveness in terms of detecting false information, which is rapidly set as a high priority due to the growing fast pace of the media. Algorithms of fake news detection are promising in enhancing fake news identification performance, but they depend on constant technology progress, compliance with ethical issues, and working collaboration with humans. Over time they continue to be built and with them comes the potential of a transformative in impact on the accuracy of the information available .
‘Fake news’ which has recently gain popularity in the current world especially through the use of social media has a negative impact on the way people perceive information and more specifically poses threats to social stability and democratic institutions. That is where current misinformation techniques are superior to past ones, and where traditional detection methods fail. Thankfully, there is generative AI with features that can effectively captured and analyse false forms of news and information. This paper unpacks how generative AI models perform best in fake news detection and at handling the content analysis of fakes, authentication of the sources of fakes, identification of deepfakes, information network analysis, and real-time monitoring of fakes. Thus, using these technologies, businesses, media, and governments can enhance the effectiveness and accuracy of the identifiable misinformation. Nevertheless, if generative AI is to be effectively used, there are several factors that need to be addressed these include the quality of the data that is fed to the model, biases that may be inherent in the model and whose implication are not fully understood, and the ethical consideration that may come with the use of the model as well as the question of model interpretability. In the future evolution of generative AI, it provides a last chance to fortify the measures against fake news and the credibility of the information found online.
Keywords: highly innovative solutions, provide better accuracy, constant technology progress, authentication of the sources of fakes, measures against fake news
Introduction
Another important question that appeared in relation to highly developed digital communities – the problem of fake news – the dissemination of purposefully false information acting against people’s trust and the stability of the society. It is thus beyond doubt that the dispensation of misinformation affects not just an individual’s decision-making process but also democracy as a democratic subject. Most of the methods for fake news detection have challenges in work, primarily attributed to the inability to analyze the great volume of existing fake posts on various websites. These matters are being awared with the support of the rather contemporary concept of generative artificial intelligence (AI). Compared to the other type of the artificial intelligence, the generative AI is capable of producing new data and argued that it quickly goes through large dataset by detecting complex relationships. For this reason, generative AI is particularly helpful in countering falsehoods and half-truths that end up proliferating the internet.[1] Thus, using generative AI, an organization enhances the probability for detecting fake news, authorizing the source, and holding manipulated media. It also provides support in the monitoring and analysis of information networks as an independent academic discipline, which means an understanding of what is known as the ‘spread’ of fake news as well as the most effective approaches to its elimination. [2]When speaking about the adoption of the generative AI in fake news detection, the challenges should be disclosed the above-mentioned issues as the benefits of its usage are tremendous. They are fakes news that make the audience lose faith in the media, and propagate disinformation on the current societal issues. Official truths, checkers, and news verifiers are deficient in their potential to respond to information that, contrary to its nature, is becoming increasingly contained. This is the problem that has been solved by the Generative AI models and is recognized as the future of faking news identification.The new opportunities for eliminating fake news are such sophisticated models which are produced for generating and analyzing intricate data patterns. This article is devoted to the use of generative AI in case of fake news detection and to the methodology, strengths, and weaknesses of these models. When initiating the pre-discourse over the topic of generative AI concerning AI technology and information accuracy, the authors wish to offer a holistic vision of the future of media verification.[3]
General characteristics and benefits of generative AI in the context of the battle against fake news
Here are features and capabilities that are unique to generative AI, yet which offer the possibility of fighting fake news. Here are some of the main features that enhance its effectiveness against misinformation:Here are 5 of the most important features that made it effective against the spread of false information:
1. Natural Language Processing (NLP)
- Context Understanding: There are many generative AI models including GPT (Generative Pre-trained Transformer), these models have little problem in detecting fake news from true news because of the context and connection that the model has of a message and the relationship of the words even if they may range phonetically similar.
- Sentiment Analysis: These models are capable of determining the polarity of the text and flag those which contain ‘appeal to emotion’ and other fallacies that are used in generating fake news.
2. Pattern Recognition
- Anomaly Detection: AI algorithms can read and are generative and the algorithms can pick out features which are likely to be fake news with reference to other similar data points.
- Linguistic Style Analysis: AI models can interpret the style of the articles so as to determine if they are authentic or fake news from the writers.
3. Content Generation and Verification
- Creating Counter-Narratives: It also provides that Generative AI can be able to generate contents with facts that can counter fake news to the public.
- Fact-checking: There is another benefit – using AI models such texts can be filtrated, as it is possible to compare the statements made in articles with some reliable data sources and databases.
4. Image and Video Analysis
- Detecting Deepfakes: The generative AI can diagnose the pictures and videos in an endeavour to locate what puzzles there may be as regards to the orientation of faces, light in addition to the synchronisation of sound.
- Image Forensics: And the use of the AI models can also be helpful in identifying the authenticity of the image based on the metadata of the image and other characteristics of the image.
5. China is assertive in the networks and in observing the spread.
- Tracing Information Spread: They can use generative AI to forecast which patterns in the info dissemination in social networks are created by which nodes participating in fake news dissemination.
- Bot Detection: It understands and can distinguish bot or automated accounts, those that spread fake news, may be deleted, or tagged so as to reduce the potentiality of influence.
6. Scalability and Real-Time Monitoring
- Handling Large Data Volumes: AI models are generative in a way that they can accommodate a large quantity of data which means it will be possible to feed it several news sources, as well as several social media accounts.
- Instant Detection: AI systems shall help analyze the situation and notify about it; it is also reasonable to introduce counter response to misinfo and its distribution.
7. Continuous Learning and Adaptation
- Learning from New Data: Even while using the Generative AI models, it is possible to employ the algorithm to the new data, search for new approaches applied by the villains in the spreading of fake news and enhance its characteristics and effectiveness.[4]
- Evolving Algorithms: Another process that can be named in the improvement of the AI algorithms is that; once the algorithms are already in the making; these are further modified to scan on a more sophisticated perspectives to determine whether there is convergence or divergence.
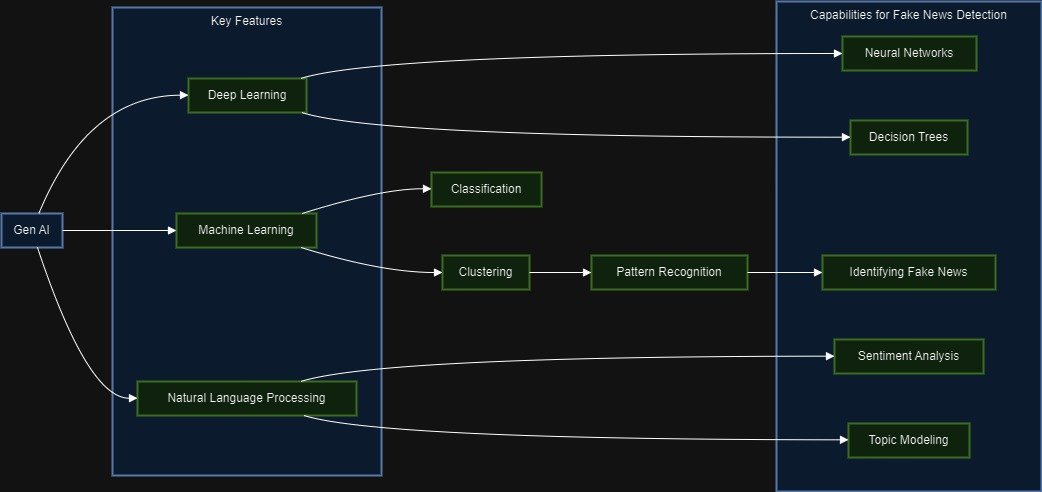
Analysis of Fake News Detection Using Generative AI models
In this paper, the authors present the case study for Fake News Detection with the help of Generative Artificial Intelligence models.
1. Data Augmentation
The generative models of the likes of GANs elaborate datasets so as to fill in the existing datasets. It assists with training more equipped fake news detection models as it offers a broader range of examples of different patterns of the tendency. [2]Reduces error rate and enhances model’s ability to detect new forms of false information.
2. Content Verification
Content With their assistive and memory abilities, generative AI can discuss the content of an article for the purpose of comparison with other authoritative sources. Verification of content has the effect of pointing out contradictions and these are some of the signs of fake news. Increases the capability of distinguishing between the real and fake news since it brings factual accuracy into the picture.
3. Style and Language Analysis
These include the language style, tone and language patterns of the news articles that generative models uses to generate articles. Fake news can also have unique features in terms of their presentation and lately, these patterns can be teach to AI. Enhances the ability of detection by the utilisation of quantitative measure, that is the linguistic signals.
4. Source Authentication
AI models able to determine credibility of the sources, based on the previous data and comparing it with the data of the most credible sources. Contains sources that are often given to spreading fake news. Improves the credibility of news by providing a confirmation of the reliability of the sources of the information provided.[5]
5. Deepfake Detection
Deepfake With the use of GANs, deepfakes can be easily detected since it is possible to compare details that are present in a set of videos or images. Is capable of identifying some of the doctored media, which are common in creating fake news and information.
Helps in minimising the effect of highly misleading images and thus works in favor of the credibility of the news outlets.
6. Social Network Analysis
They mimic how the information propagates in social networks to analyse the patterns of fake news. Identifies other conventional dissemination patterns of fake news such as the ability to spread widely without traceable and credible sources. Allows for the identification of false information in advance using information about network activity[6].
7. Anomaly DetectionIt is possible to act out definite models that are apt to diagnose inconsistencies in the news; for example, in terms of phraseology or shifts of tone. Mark as incorrect content that cannot be associated with any ‘normality’ type that is likely to be genuine information including fake news. Ever adds another form of filtering, by promoting posts that are not within the usual norms.
8. Multimodal Analysis
Generative AI interacts with data from different domains like writings, visuals and many others like films. Required to categorise the various forms of content in news stories into those that are similar or dissimilar. Increase the likelihood of presenting a number of discovered problems by using several format of the analysis.
Generative AI Methods for Fake News Detection
AI Techniques to Dominate the Real-time Identification of Fake News and Disinformation
GANs (Generative Adversarial Networks)
- Purpose: GANs can synthesize fake data as well as detect the paradox within content that seemingly can signal the existence of fake news.
- Method: It copies and emulates fake news characteristics; this makes it possible to note it because of mimicry of misinformation patterns.
Autoencoders
- Purpose: Autoencoder can be used in support of the detection of anomalies as it learns the important features of the data.
- Method: Therefore, autoencoders are able to distinguish between normal patterns in articles and fake or manipulate de ones due to deviations they are able to detect.
VAEs (Variational Autoencoders)
- Purpose: Using the fact that VAEs are able to learn the distribution of data, VAEs are applied to the detection of anomaly.
- Method: They indicate in their data stream news content that differs significantly from the standard models of a specific type of ‘fake news’.
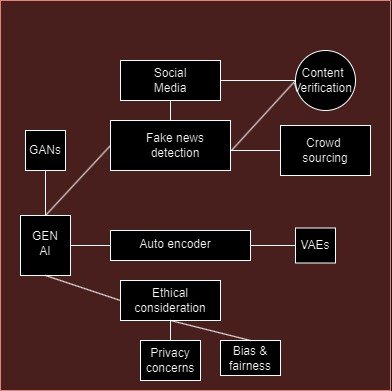
Social Media Analysis
Jot down of points to be elaborated on one of the major components of Generative AI in the aspect of Fake News detection.
Social Media Analysis
- Purpose: Watching the social networking sites in which the fake news is so swiftly and energetically disseminated.
- Method: Besides, AI observes the way the news is shared and how fast; any deviations are apparent fake news[4].
1. Content Verification Purpose:
- The necessity to raise the question of the actual credibility of the news.
- Method: The news stories are then compared to other databases and credible fact check erring services to see if there are mismatches[7].
2. Crowdsourcing
- Purpose: Using human interaction in the determination of the content of the news.
- Method: The adoption of the community to reinforce the validation and afford imprimatur to the news articles, as truthful[8].
3.Ethical Considerations
- Privacy Concerns: Security of the personal data used for the training of an AI[9].
- Bias & Fairness: In what follows, we present how the biases can be eliminated from the AI models to be able to detect and mark the fake news and feed independently[10-12].
Conclusion
Hence, the generative AI models can be said to be an innovative attempt at dealing with fake news as they present tools in supplement of the detection facilities. By the models’ capacity of data processing and generating big data, the models improve the identification of false and fraudulent info, which is useful during these tough times when fake news appear often. But their efficiency is tied to the gradual enhancement of the technologies, their ethic issues, and the presence of the human regulation to make them more useful instead of being a substitution for traditional practices in verification. In the long run, generative AI has the potentiality to make a significant contribution in the fight against fake news; consequently, the change of an efficient distribution of information is shot in the shin.
Reference
- D. Xu, S. Fan, and M. Kankanhalli, “Combating Misinformation in the Era of Generative AI Models,” in Proceedings of the 31st ACM International Conference on Multimedia, in MM ’23. New York, NY, USA: Association for Computing Machinery, Oct. 2023, pp. 9291–9298. doi: 10.1145/3581783.3612704.
- D. I. Mahmood, “Disinformation and Democracies: Understanding the Weaponization of Information in the Digital Era,” Policy J. Soc. Sci. Rev., vol. 2, no. 02, Art. no. 02, Dec. 2023.
- P. Pappachan, Sreerakuvandana, and M. Rahaman, “Conceptualising the Role of Intellectual Property and Ethical Behaviour in Artificial Intelligence,” in Handbook of Research on AI and ML for Intelligent Machines and Systems, IGI Global, 2024, pp. 1–26. doi: 10.4018/978-1-6684-9999-3.ch001.
- R. Raman et al., “Fake news research trends, linkages to generative artificial intelligence and sustainable development goals,” Heliyon, vol. 10, no. 3, Feb. 2024, doi: 10.1016/j.heliyon.2024.e24727.
- A. M. Widodo et al., “Port-to-Port Expedition Security Monitoring System Based on a Geographic Information System,” Int. J. Digit. Strategy Gov. Bus. Transform. IJDSGBT, vol. 13, no. 1, pp. 1–20, Jan. 2024, doi: 10.4018/IJDSGBT.335897.
- M. Moslehpour, T. Kurrahman, and J. Sulistiawan, “How does Cultural Intelligence Influence Teamwork Capability? The Role of Leader Emergence and Relational Identification,” Eng. Econ., vol. 35, no. 1, Art. no. 1, Feb. 2024, doi: 10.5755/j01.ee.35.1.33012.
- M. Masood, M. Nawaz, K. M. Malik, A. Javed, A. Irtaza, and H. Malik, “Deepfakes generation and detection: state-of-the-art, open challenges, countermeasures, and way forward,” Appl. Intell., vol. 53, no. 4, pp. 3974–4026, Feb. 2023, doi: 10.1007/s10489-022-03766-z.
- B. Martens, L. Aguiar, E. Gomez-Herrera, and F. Mueller-Langer, “The Digital Transformation of News Media and the Rise of Disinformation and Fake News,” Apr. 20, 2018, Rochester, NY: 3164170. doi: 10.2139/ssrn.3164170.
- L. Triyono, R. Gernowo, P. Prayitno, M. Rahaman, and T. R. Yudantoro, “Fake News Detection in Indonesian Popular News Portal Using Machine Learning For Visual Impairment,” JOIV Int. J. Inform. Vis., vol. 7, no. 3, pp. 726–732, Sep. 2023, doi: 10.30630/joiv.7.3.1243.
- A. Loth, M. Kappes, and M.-O. Pahl, “Blessing or curse? A survey on the Impact of Generative AI on Fake News,” Apr. 03, 2024, arXiv: arXiv:2404.03021. doi: 10.48550/arXiv.2404.03021.
- Sahoo, S. R., & Gupta, B. B. (2021). Multiple features based approach for automatic fake news detection on social networks using deep learning. Applied Soft Computing, 100, 106983.
- Casillo, M., Colace, F., Gupta, B. B., Santaniello, D., & Valentino, C. (2021). Fake news detection using LDA topic modelling and K-nearest neighbor classifier. In Computational Data and Social Networks: 10th International Conference, CSoNet 2021, Virtual Event, November 15–17, 2021, Proceedings 10 (pp. 330-339). Springer International Publishing.
Cite As
Nayuni I.E.S. (2024) Generative AI Models for Fake News Detection, Insights2Techinfo, pp.1