By: KUKUTLA TEJONATH REDDY, International Center for AI and Cyber Security Research and Innovations (CCRI), Asia University, Taiwan, tejonath45@gmail.com
Abstract:
This article explores the integration of deep learning chatbots as a proactive solution for detecting persistent threats, phishing attacks in real time in the digital age Traditional methods are often inflexible in the evolving cybercriminal strategies, thus requiring alternative approaches. A smaller form of artificial intelligence, deep learning, empowers cybersecurity systems by continuously learning and adapting to new paradigms. The role of chatbots in natural language processing, image recognition, and user interaction monitoring is investigated, and shows that they can detect subtle signs of phishing attempts. The advantages of this approach so is adapting to emerging threats, 24/7 vigilance, and counterfeit mitigation. Finally, the integration of deep learning chatbots is proving to help create stronger defences against ever-changing phishing attacks.
Introduction:
In our digital age, the ongoing threat of phishing attacks poses a significant challenge to individuals and organizations. To stay ahead of this evolving cyber threat, there is growing interest in integrating deep learning chatbots to improve real-time phishing attack detection capabilities. This article seeks communication bridges the gap between deep learning technology and cybersecurity, and demonstrates how chatbots can bolster sustainable security. changing phishing threats [1][2].
Understanding phishing attacks:
Phishing attacks use a variety of deceptive techniques to trick individuals into revealing sensitive information, using sophisticated social engineering techniques that make them difficult to detect through traditional security systems.
The role of deep learning:
Deep learning, a subset of artificial intelligence (AI), exhibits an impressive ability to recognize patterns and make complex decisions based on extensive datasets. When consumed by deep learning algorithm’s role, cybersecurity experts can develop advanced systems that identify subtle indicators of phishing attacks [2][3].
Real-time detection:
Traditional phishing detection methods often rely on passive codes and known signatures, making them less effective against dynamically changing phishing methods. Deep learning chatbots excel in real time research, continue to learn, and adapt to new systems and techniques used by cybercriminals.
Chatbot Assistance in Action:
Natural Language Processing (NLP): Deep learning chatbots equipped with advanced NLP can analyse messages, emails, or website content and understand context. This allows them to identify unusual and suspicious language patterns associated with phishing attempts [4].
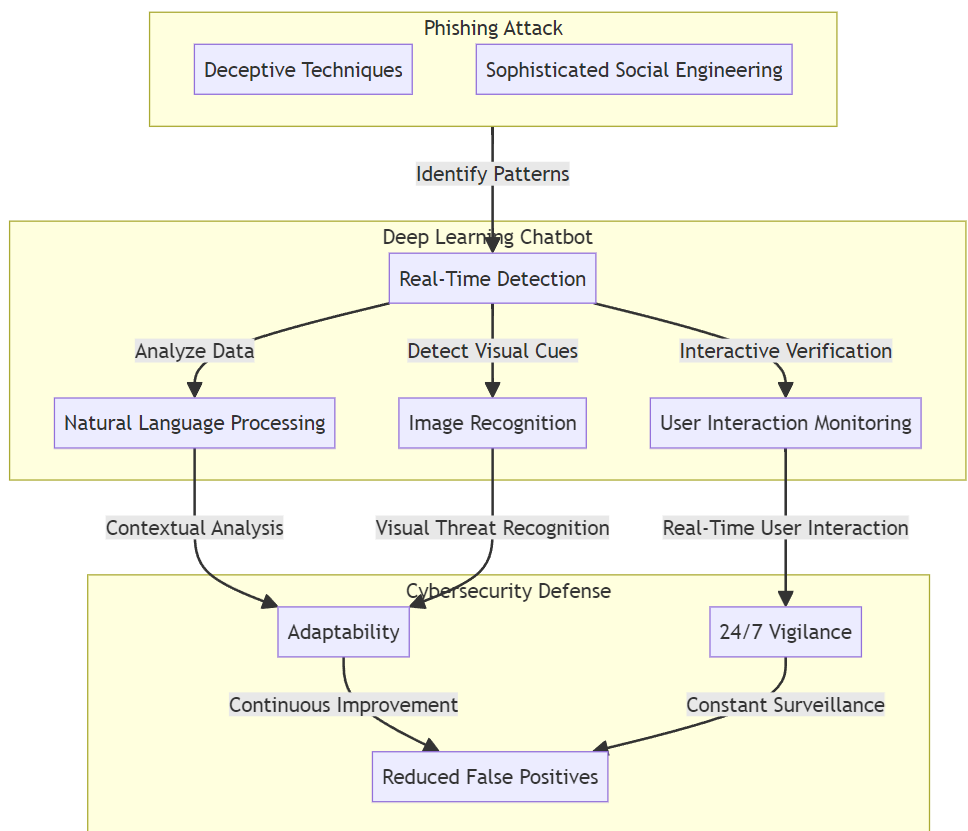
Image recognition: Phishing attacks increasingly incorporate detection features such as fake login pages or manipulated images. Deep learning algorithms can be trained to recognize these visual cues, improving the chatbot’s ability to identify potential threats.
Managing human interaction: Chatbots can interact with users in real time, ask clarifying questions or even verify a request. This form of communication helps confirm the authenticity of the communication, and adds an extra layer of security.
Benefits of Deep Learning Chatbot Assistance:
Adaptability: Deep learning models constantly improve their performance as they are exposed to new data, ensuring they adapt to emerging phishing techniques.
24/7 Vigilance: As chatbots work around the clock they provide a constant watch for phishing attacks even after traditional business hours.
Reduced False Positives: The improved learning capabilities of deep learning models help reduce false positives, reducing the risk of valid connections being incorrectly flagged so the so
Conclusion:
As sophisticated phishing attacks are on the rise, the demand for comprehensive cybersecurity measures is strong. Deep learning combined with chatbot support appears as a promising solution for real-time phishing attack detection, using the power of AI to outpace cybercriminals While using the power of deep learning algorithms combined with the interactive nature of a chatbot, organizations can enhance their cybersecurity protection and ever- changing digital. is a more stable position on the threat landscape.
References:
- Abdelhamid, S., Mallari, T., & Aly, M. (2023, June). Cybersecurity Awareness, Education, and Workplace Training Using Socially Enabled Intelligent Chatbots. In The Learning Ideas Conference (pp. 3-16). Cham: Springer Nature Switzerland.
- Τσίγγανος, Ν. (2023). Utilizing deep learning and natural language processing to recognise chat-based social engineering attacks for cyber security situational awareness.
- Gupta, M., Akiri, C., Aryal, K., Parker, E., & Praharaj, L. (2023). From ChatGPT to ThreatGPT: Impact of generative AI in cybersecurity and privacy. IEEE Access.
- Gundu, T. (2023, July). Chatbots: A Framework for Improving Information Security Behaviours using ChatGPT. In International Symposium on Human Aspects of Information Security and Assurance (pp. 418-431). Cham: Springer Nature Switzerland.
- Siyongwana, G. M. (2022). The enforcement of end-user security compliance using Chatbot (Doctoral dissertation, Cape Peninsula University of Technology).
- Kumar, A., Shankar, A., Behl, A., Arya, V., & Gupta, N. (2023). Should I share it? Factors influencing fake news-sharing behaviour: A behavioural reasoning theory perspective. Technological Forecasting and Social Change, 193, 122647.
- Sharma, A., Singh, S. K., Badwal, E., Kumar, S., Gupta, B. B., Arya, V., … & Santaniello, D. (2023, January). Fuzzy Based Clustering of Consumers’ Big Data in Industrial Applications. In 2023 IEEE International Conference on Consumer Electronics (ICCE) (pp. 01-03). IEEE.
- Zhou, Y., Song, L., Liu, Y., Vijayakumar, P., Gupta, B. B., Alhalabi, W., & Alsharif, H. (2023). A privacy-preserving logistic regression-based diagnosis scheme for digital healthcare. Future Generation Computer Systems, 144, 63-73.
- Sharma, A., Singh, S. K., Badwal, E., Kumar, S., Gupta, B. B., Arya, V., … & Santaniello, D. (2023, January). Fuzzy Based Clustering of Consumers’ Big Data in Industrial Applications. In 2023 IEEE International Conference on Consumer Electronics (ICCE) (pp. 01-03). IEEE.
Cite As
REDDY K.T (2023) How Deep Learning Chatbots Empower Cybersecurity Against Phishing Attacks, Insights2Techinfo, pp.1