By: Indu Eswar Shivani Nayuni, Department Of Computer Science & Engineering(Data Science), Student Of Computer Science & Engineering(Data Science) ,Madanapalle Institute of Technology and Science, Angallu(517325),Andhra Pradesh . indunayuni1607@gmail.com
Abstract
As it will be recalled from the discussions herein, it is expected that generative AI has the potential of encouraging the utilization of new solutions previously unaffordable to most enterprises in improving operational efficiency, revenue, and client satisfaction. However, the implementation of this type of sophisticated technology involves some problems that have to be solved, for example: data protection problems, the problem of bias, as well as the eternal question – how to incorporate this AI into legacy systems and other traditional business processes. This article outlines and describes the primary areas in which AI and machine learning experience essential challenges when in their generative form and how they can be resolved. From the real-world cases discussed in the article steps that can be taken from the industry practice are elaborated, and the essential guidelines that must be followed if enhanced performance of generative AI is to be realised and business development is to be promoted. Furthermore, it assesses the demand for ethical purposes and good governance concerning AI’s utility and employs it so that enterprises will be ready to operate in an environment that is constantly seeing higher levels of AI adoption.
Keywords: utilization of new solutions, operational efficiency, revenue, client satisfaction, traditional business process, business development, higher level of adoption.
Introduction
Small and medium enterprises in the twenty first century are using complex technologies to make it and grow. Of all those technologies, generative AI technology offers a client more capabilities to not only analyse data but also come with new ideas and solutions that revolutionize the business operation procedures.[1] For example, dynamic pricing and product development, strategic management of customer relations, optimization of work with suppliers, and other aspects are possibilities that only generative AI can offer. However, there is a question to their effectiveness – though generative AI has a great future ahead, and put a new pace to the developing of modern business, its implementation is not painless. The issues arise in the implementation include security, fairness of the algorithm, high cost of implementation, and technical difficulty in other systems of the company. They include risks that might slow down the utilization of generative AI therefore organisations end up with inefficients returns. These are issues this article addresses and includes practical strategies and tips to help organisations address when getting it right with the implementation of AI solutions. As these aspects are the critical inhibitors to the generative AI, they should be addressed proactively, companies will be all set for the further stepped-up success in the AI world of the future.
Analysis
The use of generative AI in business processes is its perfect revolution that, however, has its specific and enormous challenges that need to be addressed[2]. Understanding these issues is crucial for an organisation that is intending to adopt and apply AI technologies. And these represented in fig 1.
1. Data Privacy Security Issues
Among the topics that should be named when discussing generative AI systems, there is data; these systems must import megabytes and gigabytes of data to function adequately, and the problem of data security is not solved. The handling and misuse of the data fusion is always disastrous to the companies’ reputations; hence, data governance must be a top priority. However, besides following the letter and the spirit of such legal requirements as GDPR or CCPA to conduct business, it is necessary to go much further. The preventive measures that can help to minimize the risks and obtain the customers’ trust are the methods of anonymization of the data, as well as their proper processing.
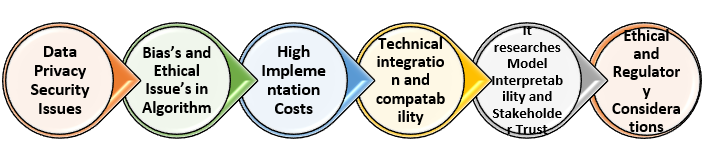
2. Bias’s and Ethical Issue’s in Algorithm
Indeed, the most cogent risk most organizations especially those intending to implement generative AI fall into is that of algorithmic bias. The model which is trained with a biased data set simply amplifies the bias and often in certain cases even aggravates it to the extent of becoming ‘unfair’ and could land the developer of the model into legal repercussions. To this, organizations need to cascade to having even more diverse and inclusive data and these data sets also need to have diversity bias detection and the usage of AI auditing is encouraged as well. In addition, the issue of accountability of an AI system has to be defined clearly, especially for those more focused and narrower AI systems that can directly impact a person’s life taking decisions about employment or credit. This framework for ethical management of AI needs to be established as the one that provides the basics of ethical operation of the AI systems. [3]
3. High Implementation Costs
The main challenge that organizations face when it comes to adopting generative AI is the cost of implementing the system together with the cost of training employees and procuring human capital to work the new system. Most of the time these costs are expensive a fact that can inhibit many firm especially the small and medium sized enterprises. Risk may be reduced by the use of pilot projects in a gradual process of implementation that should naturally show ROI necessary to continue spending. Further, organizations should consider the cost-savings strategies, which may include search for the vendors who are able to provide efficient and calibrated AI services, using open sources for the AI tools[4].
4. Technical integration and compatability
There is also the Technical Integration and Compatibility Issues that have been known to have significant impact on Oil and Gas industry[5].
Interfacing generative AI to business systems a technical exercise that can be quite challenging. Compatibility problems may be experienced and normal operations are interfered with as well as productivity is affected. First of all, it is crucial to estimate AI solutions’ compatibility with businesses’ existing systems before their integration. Adherence of the technical specifications can be another way of improving the level of integration and training of key technical personnel is another approach that can work. Also, there must be proper management of change since the incorporation of AI will disrupt the standard operations and the employees should be readied to work with the new systems[6].
5. It researches Model Interpretability and Stakeholder Trust
Despite being powerful tools, generative AI models and some of the deepest learning algorithms on which they are based are black boxes. There is always a question mark over the operations of an AI system, which is often referred to as the ‘black box’, this has been found to make stakeholders, including customers, employees, and even the regulators to be skeptical. To address the challenge of lack of trust, businesses ought to pursue explainability in AI, this is through technologies and methods that help explain AI decisions. For AI-decision adoption, it is crucial to avoid decisional opacity and explain how and on the basis of which factors decisions are made[7].
6. Ethical and Regulatory Considerations
But as with any emerging technology the legal framework that applies to the deployment of AI is bound to grow in its complexity over time[8]. There is a need for companies to keep abreast with the developments in the regulations concerning Al and guarantee that their actions in the sector are legal. Even ethical issues are crucial in this point, because improper usage of AI implies critical consequences for company’s image and possible lose in customers’ confidence. Therefore, one needs to create a framework consisting of ethics, internal audit, and communications with stakeholders so that businesses can operate in the existing ethical and regulatory environment to get the best out of AI[9]SS.
Methodologies
When it is a question of using generative AI at business processes, it is important to define the key criteria and consider all the issues that are related to the application of such systems.[10] Below are the key methodologies recommended for overcoming these barriers: Here is a list of research methodologies that are held as crucial for overcoming these barriers are in the following table.
Table 1: represents methodology in business using gen ai
Barrier | Methodology | Tools/Approaches |
Telecommunications Data Privacy and Security | – Data Anonymization – Data Quality Assurance – Consent Management | – Data Masking Software (e. g. Informatica, IBM Data Privacy Passports) – Data Governance Platforms (for example Collibra, Talend) |
Algorithmic bias and ethical issues. | – Diverse Training Data – Bias Detection Algorithms – Ethical Review Committees | – Bias detection tools (for example AI fairness 360, Fairlearn) – Other frameworks may include for instance: Ethical AI Guidelines by the European Commission |
High Implementation Costs | – Cost-Benefit Analysis – Pilot Programs – Incremental Scaling | – Cost-Benefit-Analysis-Software (e. g. @RISK,TreeAge) – Project Management Tools such as; Jira & Asana. |
Technical Integration and Compatibility Problems | – Compatibility Assessments – Interoperability Standards – Change Management Strategies | – Frameworks – Integration (for example MuleSoft, Apache Camel). – Process Support Tools (e. g Prosci ADKAR Model, Kotter’s 8-Step Process) |
Understanding & making certain decisions can be crucial for stakeholder | – Explainable AI (XAI) – Stakeholder Engagement – Documentation and Reporting | – Post hoc AI interpretable models (e. g. , LIME, SHAP) – Stakeholder Communication Platforms (for instance; Microsoft Teams, Slack, etc. ) |
Ethical and Regulatory Compliance | – Ethical AI Guidelines – Minerals and energy audits and assessments – Stakeholder Consultation | – Record Retention and Legal Hold Software (e. g. , OneTrust, TrustArc) – Ethics Committees or Consultations from Outside the institution |
Conclusion
Therefore, one is to conclude that the discussed difficulties cannot be viewed as ultimate barriers to the integration of the generative AI into the framework of the modern businesses despite of the fact that the implementation of the generative AI into the framework of the modern businesses is accompanied by quite obvious difficulties. All the same, there are some measures that businesses can adopt in order to enhance the effectiveness of AI adoption the following being some of them: Improved data governance: bias removal steps and cost containment measures Ethical issues As mentioned earlier based on the methodologies reported in the article, organisations implementing generative AI are in a favorable position of realizing on its benefits depending on the ideas provided in this article but at the same time implement this technology with caution to minimize on some of the unethical aspects or infringement of the laws as provided by the different countries.
Thus, the companies that will be able to address these challenges and implement the relevant tools and techniques in the course of AI advancement, will be referred to as the winners in the given field. Thus, the continuation of business development is rooted in its adaptability and imagination thus, generative AI is an instrument that may turn into a competitive advantage for those ready to embrace the new technology. Climbing these barriers would provide companies with even higher levels of productivity, innovative solutions and revenues for the determination of further continuity in AI enabled environment.
References:
- M. Hüther, “Digitalisation: An engine for structural change – A challenge for economic policy,” IW Policy Paper, Working Paper 15/2016E, 2016. Accessed: Aug. 14, 2024. [Online]. Available: https://www.econstor.eu/handle/10419/148408
- S. Feuerriegel, J. Hartmann, C. Janiesch, and P. Zschech, “Generative AI,” Bus. Inf. Syst. Eng., vol. 66, no. 1, pp. 111–126, Feb. 2024, doi: 10.1007/s12599-023-00834-7.
- P. Pappachan, Sreerakuvandana, and M. Rahaman, “Conceptualising the Role of Intellectual Property and Ethical Behaviour in Artificial Intelligence,” in Handbook of Research on AI and ML for Intelligent Machines and Systems, IGI Global, 2024, pp. 1–26. doi: 10.4018/978-1-6684-9999-3.ch001.
- H. Abdi, “Profit-based unit commitment problem: A review of models, methods, challenges, and future directions,” Renew. Sustain. Energy Rev., vol. 138, p. 110504, Mar. 2021, doi: 10.1016/j.rser.2020.110504.
- X. Wang, Y. Chen, and W. Zhu, “A Survey on Curriculum Learning,” IEEE Trans. Pattern Anal. Mach. Intell., vol. 44, no. 9, pp. 4555–4576, Sep. 2022, doi: 10.1109/TPAMI.2021.3069908.
- M. Rahaman, S. Chattopadhyay, A. Haque, S. N. Mandal, N. Anwar, and N. S. Adi, “Quantum Cryptography Enhances Business Communication Security,” vol. 01, no. 02, 2023.
- E. Ferrara, “Fairness and Bias in Artificial Intelligence: A Brief Survey of Sources, Impacts, and Mitigation Strategies,” Sci, vol. 6, no. 1, Art. no. 1, Mar. 2024, doi: 10.3390/sci6010003.
- E. Meskys, J. Kalpokiene, P. Jurcys, and A. Liaudanskas, “Regulating Deep Fakes: Legal and Ethical Considerations,” Dec. 02, 2019, Rochester, NY: 3497144. Accessed: Aug. 14, 2024. [Online]. Available: https://papers.ssrn.com/abstract=3497144
- A. Koy and A. B. Çolak, “The Intraday High-Frequency Trading with Different Data Ranges: A Comparative Study with Artificial Neural Network and Vector Autoregressive Models,” Arch. Adv. Eng. Sci., vol. 2, no. 3, Art. no. 3, 2024, doi: 10.47852/bonviewAAES32021325.
- S. Chatterjee, S. K. Ghosh, and R. Chaudhuri, “Knowledge management in improving business process: an interpretative framework for successful implementation of AI–CRM–KM system in organizations,” Bus. Process Manag. J., vol. 26, no. 6, pp. 1261–1281, Jan. 2020, doi: 10.1108/BPMJ-05-2019-0183.
- Li, K. C., Gupta, B. B., & Agrawal, D. P. (Eds.). (2020). Recent advances in security, privacy, and trust for internet of things (IoT) and cyber-physical systems (CPS).
- Chaudhary, P., Gupta, B. B., Choi, C., & Chui, K. T. (2020). Xsspro: Xss attack detection proxy to defend social networking platforms. In Computational Data and Social Networks: 9th International Conference, CSoNet 2020, Dallas, TX, USA, December 11–13, 2020, Proceedings 9 (pp. 411-422). Springer International Publishing.
Cite As
Nayuni I.E.S (2024) Overcoming Barriers: Generative AI enters Post-modern Business Models, Insights2Techinfo, pp.1