By: Poojitha Nagishetti, Department of Computer Science and Engineering (Data Science), Madanapalle Institute of Technology and Science, Angallu,517325, Andhra Pradesh, poojimurali2330@gmail.com
Abstract –
Asthma is a chronic respiratory problem that impacts millions of people worldwide, where the use of big data analytics and artificial intelligence applications have been the main changes. These are some of the territories where these technologies are making ground-breaking improvements to asthma, from enabling early diagnosis and prediction of the disease’s exacerbations to individualized treatment plans. Anticipatory care uses big data of medical records, self-assessment tools, and environmental conditions to predict the progression of diseases and need for hospitalization. While the functionality of AI in a plethora of regions of asthma comprises of the accurate diagnosis of the disease, identifying the right patient, and providing precise treatment for such individuals. This article presents the state of the art & outlook of predictive analytics & artificial intelligence in management of asthma.
Introduction –
Asthma is a long-term common chronic illness of the respiratory organs that provides the need for medical care for more than 300 million people worldwide and results in increased healthcare expenditures. The familiar manifestations of asthma involve airway narrowing due to inflammation and increased airway sensitivity; the asthma signs are wheezing, shortness of breath, chest estimation, and coughing. However, current treatments remain insufficient in effectively managing symptoms and controlling the frequency of exacerbation episodes; many patients end up presenting at the emergency rooms or being admitted to the hospital.
Prior asthma management strategies mainly included routine observation of clinical signs, symptoms’ self-reports and spirometry in patients. However, these are frequently applied in a reactive manner and are incapable of indicating when intensifications will arise or even customizing therapy into patient specific. Such a deficit requires the search for new proactive approaches based on data and technologies.
The use of predictive analytics and artificial intelligence as still nascent, offer great solutions suggestive of how Asthma can be handled[1]. Predictive analytics are defined as the process in which past and current information is used to make future estimations and AI technologies such as machine learning and natural language processing can also help make sense of large sets of data to improve healthcare decision-making and tailor care.
Technology and specifically, the process of using predictive analytics and AI to handle asthma care is the topic of this article, addressing how such technologies can enhance the capacity to diagnose the disorder early electronics, predict asthmatic attacks, and help to design a fitting treatment plan for patients[2]. It deals with the issues and concerns implied in the utilization of these technologies in clinical practice as well as the ethical issues that come with its use, and prospects of asthma management in contextual to data-intensive patient care.
Overview of Asthma –
Asthma is a chronic inflammatory disease that affects the airways making the airflow to the lung’s variable, it can either improve on its own or with the help of medicine. It also exhibits signs like whistling sound when breathing, difficulty breathing, chest constrictions, and coughing especially during the night or in the wee hours of the morning. These symptoms are precipitated by things like allergens, respiratory infections, exercise, cold air, and environment irritants.
Asthma is a condition that is accepted to exhibit diversity hence is different in people it affects. Those affected may experience a mild form of the disease characterized by occasional attacks with mild symptoms or severe form that results into frequent and severe attacks that reduce the quality of life. Asthma varies in phenotype depending on the age it was first diagnosed, if the patient is allergic, or if he/she has other diseases.
Epidemiology in Asthma it is estimated that over 300 million people suffer from the condition and Asthma is ranked as one of the most prevalent chronic illnesses in the entire world. Incidence of asthmatic symptoms is not same all over the world and depends on the genetic make-up of the population and its susceptibility to disease, exposure to environment, and its economic status[3]. Although asthma can affect individuals of all ages it is most diagnosed in children. Emergency visits and hospitalisations are relatively common and there is a need for constant medication making the disease a real burden to the health systems.
The actual process of asthma is the chronic inflammation of the airways which induces airway hypersensitivity, excessive secretion of mucus, and alteration in airway structure and composition. There is a strong immunogenetic basis to inflammation, which involves a response to certain genes and chemical sensitization and encourages the release of eosinophils, mast cells, and T-helper cells. This immune response leads to production of a cytokines and leukotrienes which trigger the signs and symptoms of asthma.
Predictive Analytics in Asthma Management –
Predictive analytics presupposes the utilization of historical and/or current information to find out certain tendencies and make prognosis of certain tendencies. In asthma care, big data integrated predictive models allow to consider EHRs, patient-reported data, environmental and hereditary factors and make prognosis about exacerbations, hospitalizations, and disease evolution. These predictions help clinicians to anticipate, provide timely interventions, modify patients’ management, and avoid adverse consequences.
Another use of the concept of predictive analytics can be seen in the creation of risk adjustment models. These models help to split the patients according to their risk to get an asthma attack. For instance, a model might take information about changes in lung function, physical activity, and exposure to certain factors from the wearable devices and warn patients and doctors about the risks.
Artificial Intelligence in Asthma Management –
Apart from that, it is also evident that the respiratory diseases and the chronic conditions, and particularly asthma, can be managed through artificial intelligence, hence benefitting the patients[4]. Some of the AI technologies are Machine learning, natural language processing, and computer vision can analyse terabytes
of complex data that involves human record, scans, ones, forensics’ data, genomics data, and data captured from the human body using wearable devices.
In diagnostics, the use of deep learning algorithms in the evaluation of lung tests, MRI scans, CT scans, voice analysis, etc., can in a more advanced and at a preliminary level distinguish between asthma and other lung ailments[5]. Such diagnostics from AI lowers the odds of wrong diagnosis and at the same time make a sure that right treatment of a patient is done[6]. Furthermore, the usage of artificial intelligence also allows creating the individual therapeutic schedules based on such data as response to specific drugs, patient’s lifestyles, or exposure of the patient to various factors in the environment. This approach in delivering treatment depends on the patient needs and therefore it implies that a smaller percentage of the patients will have an episode of worsening of the condition.
Telemedicine is also another advantage of AI because signs, prescriptions, and exposure to the physical environment can also be conducted through Smart gadgets and apps[7]. The information and data are taken and analysed immediately and backed by using AI procedures to identify when signs of Asthma compensation begin to deteriorate to apply corrections. The approach of management of the condition, hence enhancing the general well-being of the patient, not only achieves a favourable improvement in the state of health for the individual in question but is also effective in the duration of the care in avoiding the complications that result in hospitalization.
Thus, it is seen that the asthma management is one of the big progresses that can be done by the help of AI to bring the Proactive and individualized and enhanced form of the chronic disease. Figure 1 shows the overview of Exacerbation Prediction Model.
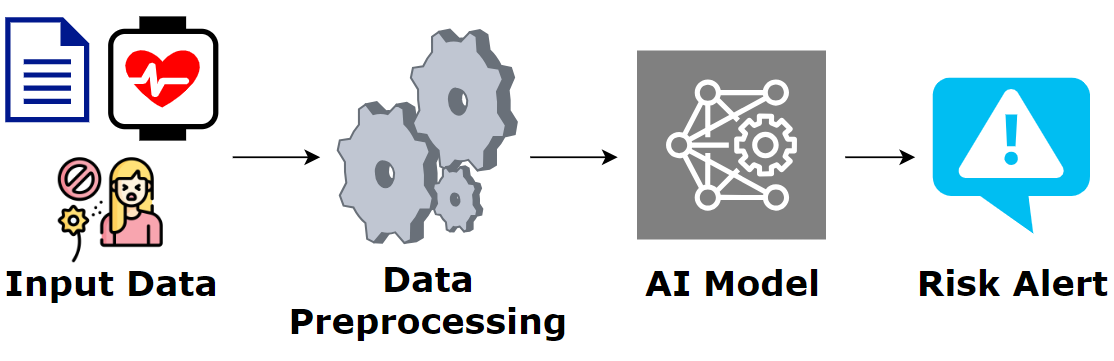
Challenges and Ethical Considerations –
Still, there is much promise in applying PA and AI in asthma care; however, several limitations must be considered[8]. The problems of data quality and data access are still topical; these issues arise from the fact that predictive models are built on rather extensive and diverse data sets. Furthermore, the implementation of AI tools seems to be time-consuming because they are introduced into clinical practice, and health care professionals need to be trained and adapt to their use.
There are also ethic issues which cannot be overlooked. There is always a great problem concerning data privacy and security, and bias in algorithms used to make decisions in healthcare[9]. Thus, to avoid adverse effects and retain patients’ trust, it is crucial to use AI tools that are transparent and non-bias, used alongside clinical decision-making processes.
Future Directions –
The further advancement of asthma outcome prediction and active utilization of predictive analytics and AI should be a focus of general practice. With the ongoing progression of technology, we will observe the model’s adopting data in real time from the possible sources like genomics, Microbiomes, and even social determinants of health[10]. These new models shall in fact help introduce dignified and precise asthma care, hence easing the impact of the disease on patients’ lives.
Also, future engagement of technology developers, clinicians, and patients will be mandatory to deliver effective AI tools that are easy to use and will promote fairness among patients. There should also be a focus on the availability of these technologies especially to he who needs it most through the availability of health data for all the benefit of incorporating artificial intelligence and predictive analytical models.
Criteria | AI-Driven Diagnostics | Traditional Methods |
Accuracy | Higher accuracy due to advanced algorithms and data analysis | Variable accuracy; often dependent on subjective interpretation and standard testing procedures |
Early Detection | Can detect asthma and exacerbations earlier through continuous monitoring and analysis | Typically reactive; relies on patient-reported symptoms and periodic testing |
Data Integration | Integrates diverse data sources (e.g., wearable sensors, EHRs) for comprehensive analysis | Primarily based on clinical assessments and patient history, which may not capture all relevant data |
Personalization | Provides personalized treatment recommendations based on individual data (e.g., genetics, lifestyle) | General treatment guidelines based on population averages and clinical experience |
Real-Time Monitoring | Continuous monitoring with real-time data analysis to detect changes and predict exacerbations | Limited to scheduled visits and intermittent monitoring, which may miss critical changes |
Intervention | Enables proactive interventions by predicting exacerbations and adjusting treatment plans accordingly | Often reactive, addressing issues only after symptoms or exacerbations occur |
Patient Engagement | Enhances patient engagement with real-time feedback and actionable insights through mobile apps and devices | Limited patient engagement; relies on self-reporting and adherence to treatment plans |
Cost | Initial high setup cost for AI tools and technologies; potential long-term savings through reduced hospitalizations | Lower initial cost but may incur higher long-term costs due to frequent emergency visits and hospitalizations |
Conclusion –
The use of PA and AI in asthma care reinvents how this chronic disease is managed in the modern society. These technologies can help recognize the disease at an early stage, foresee worsening of the condition, and individualize the management of the disease, which could eventually help the patients’ condition and decrease the negative effects of asthma on the patients and healthcare structures. However, to employ it fully, it is paramount to deal with difficulties connected with data concerns, ethical issues, and its implementation into practice. Achieving more accurate prediction, treatment customization and early intervention on Asthma management will be the vision of Asthma in the next future with more creative innovations and efficient collaborations.
References –
- N. Kang, K. Lee, S. Byun, J.-Y. Lee, D.-C. Choi, and B.-J. Lee, “Novel Artificial Intelligence-Based Technology to Diagnose Asthma Using Methacholine Challenge Tests,” Allergy Asthma Immunol. Res., vol. 16, no. 1, pp. 42–54, Dec. 2023, doi: 10.4168/aair.2024.16.1.42.
- S. Fontanella, A. Cucco, and A. Custovic, “Machine learning in asthma research: moving toward a more integrated approach,” Expert Rev. Respir. Med., vol. 15, no. 5, pp. 609–621, May 2021, doi: 10.1080/17476348.2021.1894133.
- C. Kallis, R. A. Calvo, B. Schuller, and J. K. Quint, “Development of an Asthma Exacerbation Risk Prediction Model for Conversational Use by Adults in England,” Pragmatic Obs. Res., vol. 14, pp. 111–125, Dec. 2023, doi: 10.2147/POR.S424098.
- H. Y. Seol et al., “Artificial intelligence-assisted clinical decision support for childhood asthma management: A randomized clinical trial,” PLOS ONE, vol. 16, no. 8, p. e0255261, Aug. 2021, doi: 10.1371/journal.pone.0255261.
- M. Moslehpour, A. Firman, C.-H. Lin, İ. Bilgiçli, T. K. Tran, and T. T. H. Nguyen, “The moderating impact of government support on the relationship between tourism development and growth, natural resources depletion, sociocultural degradation, economic environment, and pollution reduction: case of Indonesian economy,” Environ. Sci. Pollut. Res., vol. 30, no. 19, pp. 56863–56878, Apr. 2023, doi: 10.1007/s11356-023-26231-x.
- K. C. H. Tsang, H. Pinnock, A. M. Wilson, and S. A. Shah, “Application of Machine Learning Algorithms for Asthma Management with mHealth: A Clinical Review,” J. Asthma Allergy, vol. 15, pp. 855–873, Jun. 2022, doi: 10.2147/JAA.S285742.
- A. Hakizimana, P. Devani, and E. A. Gaillard, “Current technological advancement in asthma care,” Expert Rev. Respir. Med., vol. 0, no. 0, pp. 1–14, doi: 10.1080/17476348.2024.2380067.
- M. Moslehpour, A. Firman, J. Sulistiawan, P.-K. Lin, and H. T. T. Nguyen, “Unraveling the Puzzle of Turnover Intention: Exploring the Impact of Home-Work Interface and Working Conditions on Affective Commitment and Job Satisfaction,” Behav. Sci., vol. 13, no. 9, Art. no. 9, Sep. 2023, doi: 10.3390/bs13090699.
- I. Setiawan et al., “Utilizing Random Forest Algorithm for Sentiment Prediction Based on Twitter Data,” 2022, pp. 446–456. doi: 10.2991/978-94-6463-084-8_37.
- Z. Su, L. He, S. P. Jariwala, K. Zheng, and Y. Chen, “‘What is Your Envisioned Future?’: Toward Human-AI Enrichment in Data Work of Asthma Care,” Proc ACM Hum-Comput Interact, vol. 6, no. CSCW2, p. 267:1-267:28, Nov. 2022, doi: 10.1145/3555157.
- Gupta, B. B., & Panigrahi, P. K. (2022). Analysis of the Role of Global Information Management in Advanced Decision Support Systems (DSS) for Sustainable Development. Journal of Global Information Management (JGIM), 31(2), 1-13.
- Gupta, B. B., & Narayan, S. (2021). A key-based mutual authentication framework for mobile contactless payment system using authentication server. Journal of Organizational and End User Computing (JOEUC), 33(2), 1-16.
Cite As
Nagishetti P. (2024) Revolutionizing Asthma Care with Predictive Analytics and AI, Insights2Techinfo, pp.1