By: KUKUTLA TEJONATH REDDY, International Center for AI and Cyber Security Research and Innovations (CCRI), Asia University, Taiwan, tejonath45@gmail.com
Abstract:
Phishing attacks, which are on the rise in the digital landscape, require innovative and accurate detection solutions. This article examines the Hybrid Deep Learning (HDL)-Centric Phishing Detection System (PDS), an advanced cybersecurity technology designed to thwart phishing attempts The system uses a combination of deep learning techniques uses alongside traditional machine learning algorithms, including convolutional neural networks (CNNs) and recursion This hybrid approach with neural networks (RNNs) enables HDL-Centric PDS to handle large amounts of data, extract complex patterns, and distinguish between legitimate phishing websites The article delves into the design and operation of HDL-centric PDS, its Highlighting components such as data collection, feature extraction, training phases , real-time detection and alert generation, the benefits of the system include high accuracy, adaptability to revolving threats, efficiency and user-friendliness. However, challenges such as data quality and evolving phishing techniques require continued research and development. The article emphasizes the importance of user education and recommends strategies for increasing system effectiveness. Overall, HDL-Centric PDS stands as a promising solution in the ongoing fight against phishing attacks, ensuring a secure digital environment for individuals and organizations.
Introduction:
In these recent years, technology has advanced a lot in fact made many parts of human life easier and more convenient. But this advancement is also causes to increase in cyberthreats, with phishing attempts and becoming one of the most common and dangerous from of cyber-attack. Phishing attack is a fraudulent attempt to get sensitive data like credit card details and login credentials by pretending as a trustworthy companies or subscription alter mails.
Researchers and cybersecurity specialists are developing so many methods like Hybrid Deep learning (HDL)-Centric Phishing Detection system (PDS) to fight with these types of cyber-attacks [1]. This article is focusing on hybrid deep learning approaches, this system leverages the power of cutting-edge technology to detect and block phishing attempts. HDL-centric PDS will provide a strong defence system against sophisticated and ever-evolving phishing attacks by involving deep learning architectures like Convolutional Neural Networks (CNNs) and Recurrent Neural Networks (RNNs), and some standard machine learning techniques [1].
Understanding Phishing Detection:
Phishing detection systems are created to identify and prevent from phishing attempts. Previously these systems used to depend on rule-based methods, which has limitations, when it handling advanced or complex phishing attacks. By introducing deep learning methods, particularly hybrid deep learning has resulted to have a new level in phishing detection.
Phishing Detection Using Hybrid Deep learning:
In this we need combine Hybrid Deep learning and machine learning algorithms with the features of several deep learning technologies, including Recurrent Neural Networks (RNNs) and Convolutional Neural Networks (CNNs). This method will enhance the system capacity to differentiate between legitimate and phishing websites in the farmwork of phishing detection system by processing vast volumes of data and identifying complex patterns.
Architecture and functioning of HDL-Centric PDS:
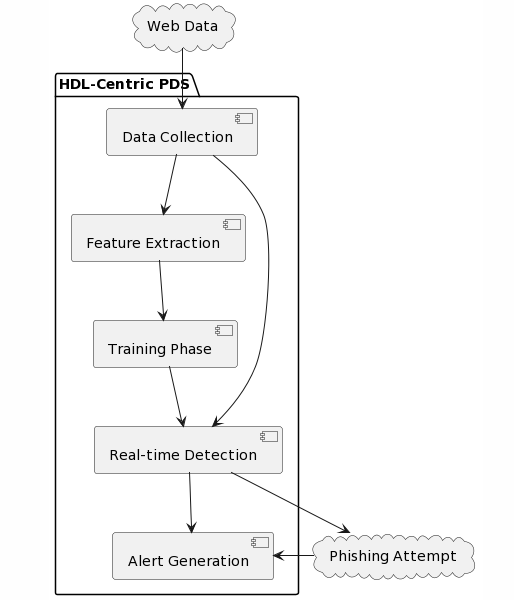
Components of the HDL-Centric PDS:
Data Collection: In this we need to collect large amount of web data, including web content, URLs, and metadata, to create a complete dataset for analysis.
Feature extraction: Hybrid deep learning models will extract meaningful features from raw data, it will capture complex patterns and characteristics of specific to phishing attacks.
Training phase: During this phase, HDL-Centric phishing detection system will train its neural networks using labelled data, enabling the system to detect and recognize phishing techniques.
Real-time analysis: In this trained model will use real time phishing detection. When a user visits a website, the system will analyse its quality and compares it with scholarly observations to identify the phishing attack.
Warning generation: Whenever the system detects the phishing attack, it will raise an alert to user and block the site by preventing potential data breaches.
Advantages of HDL-Centric PDS:
High Accuracy: Hybrid deep learning techniques will provide high and better accuracy in detecting phishing attacks.
Adaptation: The system can able to adapt with new phishing techniques for ensuring a strong defence system against latest threats.
Efficiency: HDL-Centric PDS will process data faster and it will provide real time detection and response for phishing attacks and enhances overall cybersecurity.
User-Friendly: It will work easily in the background it just requires minimal user intervention, providing a hassle-free experience for individuals and organizations.
Challenges and Limitations:
Data Quality: The effectiveness of HDL-Centric PDS is highly dependent on the quality and variety of training data. Poor data quality can lead for biased or inaccurate results.
Technical Resources: The implementation of hybrid training and deep learning models to required to significant computing resources, and making it difficult for smaller organizations with limited resources.
Evolving Phishing Techniques: As Cybersecurity continually will develop new and sophisticated exploits, the system must keep pace with these changes to keep up has worked well.
Conclusion:
Hybrid deep learning centric phishing detection system (PDS) represents a major advance in cybersecurity. Combining the power of deep learning algorithms, the system will provide phishing detection with accurate, efficient and scalable protecting individuals and organizations from potential data breaches through the challenges by analysing ongoing, upgrade, and user education in HDL-centric PDS development will help, making it an important tool in combating phishing attacks and ensuring a securing digital environment for all.
References:
- Anitha, J., & Kalaiarasu, M. (2022). A new hybrid deep learning-based phishing detection system using MCS-DNN classifier. Neural Computing and Applications, 1-16.
- Adebowale, M. A., Lwin, K. T., & Hossain, M. A. (2023). Intelligent phishing detection scheme using deep learning algorithms. Journal of Enterprise Information Management, 36(3), 747-766.
- Bountakas, P., & Xenakis, C. (2023). HELPHED: Hybrid Ensemble Learning PHishing Email Detection. Journal of Network and Computer Applications, 210, 103545.
- Lin, Y., Liu, R., Divakaran, D. M., Ng, J. Y., Chan, Q. Z., Lu, Y., … & Dong, J. S. (2021). Phishpedia: A hybrid deep learning based approach to visually identify phishing webpages. In 30th USENIX Security Symposium (USENIX Security 21) (pp. 3793-3810).
- Zhang, X., Shi, D., Zhang, H., Liu, W., & Li, R. (2018, October). Efficient detection of phishing attacks with hybrid neural networks. In 2018 IEEE 18th International Conference on Communication Technology (ICCT) (pp. 844-848). IEEE.
- Chiew, K. L., Tan, C. L., Wong, K., Yong, K. S., & Tiong, W. K. (2019). A new hybrid ensemble feature selection framework for machine learning-based phishing detection system. Information Sciences, 484, 153-166.
- Wang, L., Li, L., Li, J., Li, J., Gupta, B. B., & Liu, X. (2018). Compressive sensing of medical images with confidentially homomorphic aggregations. IEEE Internet of Things Journal, 6(2), 1402-1409.
- Stergiou, C. L., Psannis, K. E., & Gupta, B. B. (2021). InFeMo: flexible big data management through a federated cloud system. ACM Transactions on Internet Technology (TOIT), 22(2), 1-22.
- Gupta, B. B., Perez, G. M., Agrawal, D. P., & Gupta, D. (2020). Handbook of computer networks and cyber security. Springer, 10, 978-3.
- Bhushan, K., & Gupta, B. B. (2017). Security challenges in cloud computing: state-of-art. International Journal of Big Data Intelligence, 4(2), 81-107.
Cite As
REDDY K.T (2023) The Evolution of Hybrid Deep Learning in Phishing Detection, Insights2Techinfo, pp.1