By: Himanshu Tiwari, International Center for AI and Cyber Security Research and Innovations (CCRI), Asia University, Taiwan, nomails1337@gmail.com
Introduction:
Chatbots streamline communication, automate procedures, and improve user experiences across businesses in the evolving AI landscape. Federated learning is revolutionizing chatbot model development and optimization as technology advances. This article explores federated learning in chatbots, its fundamentals, its many benefits, and its potential for conversational AI.
Knowledge of Federated Learning:
Federated learning is a decentralized machine learning method that revolutionizes model training. This strategy trains chatbot models on many decentralized edge devices or servers with local data samples. Federated learning allows devices to learn locally without exchanging raw data, unlike centralized techniques. Chatbots can learn from varied datasets while protecting user privacy by aggregating the updated models from each device into a global model[1].
Chatbot Federated Learning Benefits:
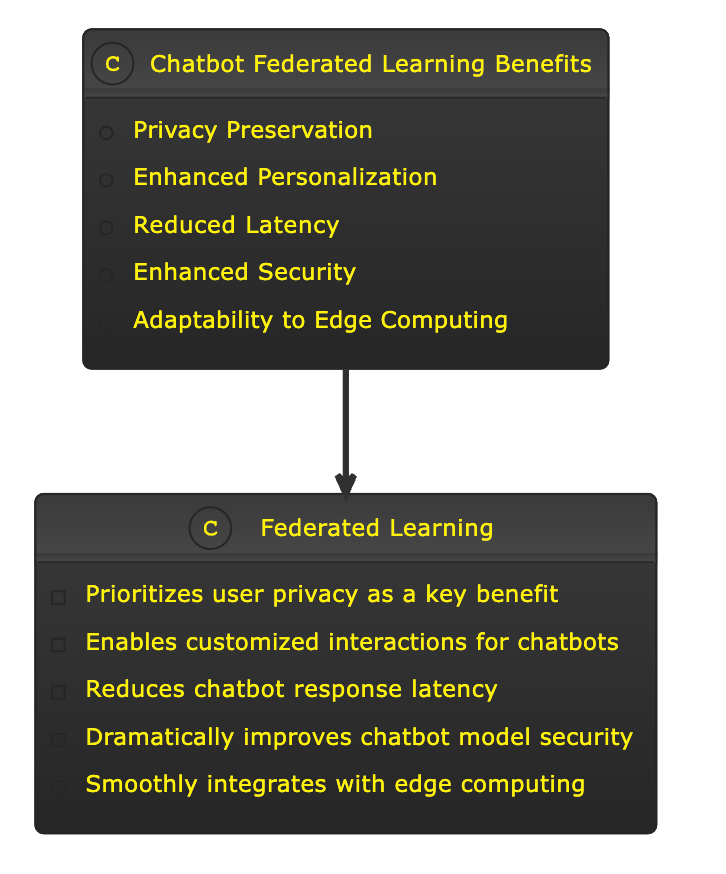
1. Privacy Preservation: Federated learning prioritizes user privacy as a key benefit. Centralizing data in standard machine learning models compromises privacy. Federated learning lets you train models locally without sharing sensitive data. Data protection and privacy standards are increasingly important, thus this fits[2].
2. Enhanced Personalization: Federated learning enables chatbots to provide customized interactions. The model learns from diverse datasets across devices to understand more user behaviors and preferences. This allows chatbots to dynamically adapt to users’ preferences, making conversations more personalized and engaging[1][2].
3. Reduced Latency: Federated learning’s decentralized nature reduces chatbot response latency. Localized model updates on devices can be done in real time, reducing server communication. Low latency is especially useful in real-time customer service[1][3].
4. Enhanced Security: Federated learning dramatically improves chatbot model security. Keeping raw data on the device reduces the danger of data breaches during model training or updates. Healthcare and finance, where data confidentiality is crucial, require this increased protection[3].
5. Adaptability to Edge Computing: Federated learning smoothly integrates with the growing trend of edge computing. Decentralised learning lets edge chatbots adapt and improve without a central server. In intermittent or limited internet connectivity situations, this adaptability is crucial[4].
Issues and Considerations:
Federated learning has many benefits, but its drawbacks must be addressed. Data representativeness among decentralized devices, transmission overhead, and model accuracy aggregate optimization may be problems. Federated learning in chatbots must overcome these hurdles to reach its full potential[5].
Conclusion:
In conclusion, federated learning changes chatbot model creation and optimization. Federated learning meets changing AI ethics and user expectations by prioritizing privacy, personalization, and adaptability. Federated learning helps chatbot developers and organizations create more efficient, safe, and user-centric chatbot experiences as conversational AI advances. Federated learning guides us toward a future where chatbot technology balances creativity and privacy as AI evolves.
References:
- Li L, Fan Y, Tse M, Lin KY. A review of applications in federated learning. Computers & Industrial Engineering. 2020 Nov 1;149:106854.
- Mammen PM. Federated learning: Opportunities and challenges. arXiv preprint arXiv:2101.05428. 2021 Jan 14.
- Wei K, Li J, Ding M, Ma C, Yang HH, Farokhi F, Jin S, Quek TQ, Poor HV. Federated learning with differential privacy: Algorithms and performance analysis. IEEE Transactions on Information Forensics and Security. 2020 Apr 17;15:3454-69.
- Guidi B. When blockchain meets online social networks. Pervasive and Mobile Computing. 2020 Feb 1;62:101131.
- Truex S, Baracaldo N, Anwar A, Steinke T, Ludwig H, Zhang R, Zhou Y. A hybrid approach to privacy-preserving federated learning. InProceedings of the 12th ACM workshop on artificial intelligence and security 2019 Nov 11 (pp. 1-11).
- Xu, Z., He, D., Vijayakumar, P., Gupta, B., & Shen, J. (2021). Certificateless public auditing scheme with data privacy and dynamics in group user model of cloud-assisted medical WSNs. IEEE Journal of Biomedical and Health Informatics.
- Liu, R. W., Guo, Y., Lu, Y., Chui, K. T., & Gupta, B. B. (2022). Deep network-enabled haze visibility enhancement for visual IoT-driven intelligent transportation systems. IEEE Transactions on Industrial Informatics, 19(2), 1581-1591.
- Zhou, Z., Li, Y., Li, J., Yu, K., Kou, G., Wang, M., & Gupta, B. B. (2022). Gan-siamese network for cross-domain vehicle re-identification in intelligent transport systems. IEEE Transactions on Network Science and Engineering.
- Zhang, Q., Guo, Z., Zhu, Y., Vijayakumar, P., Castiglione, A., & Gupta, B. B. (2023). A deep learning-based fast fake news detection model for cyber-physical social services. Pattern Recognition Letters, 168, 31-38.
Cite As
Tiwari H. (2023) Unleashing the Power of Federated Learning in Chatbots: A Revolutionary Approach, Insights2Techinfo, pp.1