By: KUKUTLA TEJONATH REDDY, International Center for AI and Cyber Security Research and Innovations (CCRI), Asia University, Taiwan, tejonath45@gmail.com
Abstract:
In the digital age, where phishing attacks pose a constant threat to online security, the need for effective detection techniques is paramount. Signature-based signatures, a widely used method, seek to detect fraudulent attempts by comparing incoming email messages or known phishing signatures This article examines signature-based detection methods, including speed, accuracy, and resource efficiency The benefits of vision are discussed in detail. But it also sheds light on its limitations, particularly its inability to prevent new or previously unseen phishing attacks. The article highlights the importance of a multi-layered cybersecurity strategy, combining signature-based approaches with advanced methods such as behavioural assessment and user education programs, de building strong defences in the growing environment of phishing threats is essential for individuals and organizations looking to protect their sensitive information in the digital realm.
Introduction:
In today’s digital age where communication is primarily through emails and online messaging, phishing attacks have become an ever-present threat Sending fraudulent emails or messages to trick recipients into revealing sensitive information Cybersecurity experts have developed various strategies countermeasures against these threats, one of which is signature-based detection [1]. This method includes comparing incoming emails or messages with known phishing signatures, unique patterns or characteristics found in phishing emails, to detect and prevent phishing attempts. In this article we will discuss the use of signature based identification, and benefits, phishing attacks. We’ll go deeper into counter fighting and its limitations [2].
How Signature-Based Detection Works:
At its core, signature-based signatures rely on a large database of known phishing signatures. These signatures are basically digital fingerprints of phishing emails, showing specific patterns, keywords, or elements commonly found in fraudulent messages When you receive an email or message, security systems analyse it information and compare it to signatures in a database [1]. If a match is found, the email will be flagged as a phishing attempt and appropriate action will be taken to prevent the recipient from falling victim to the attack [3].
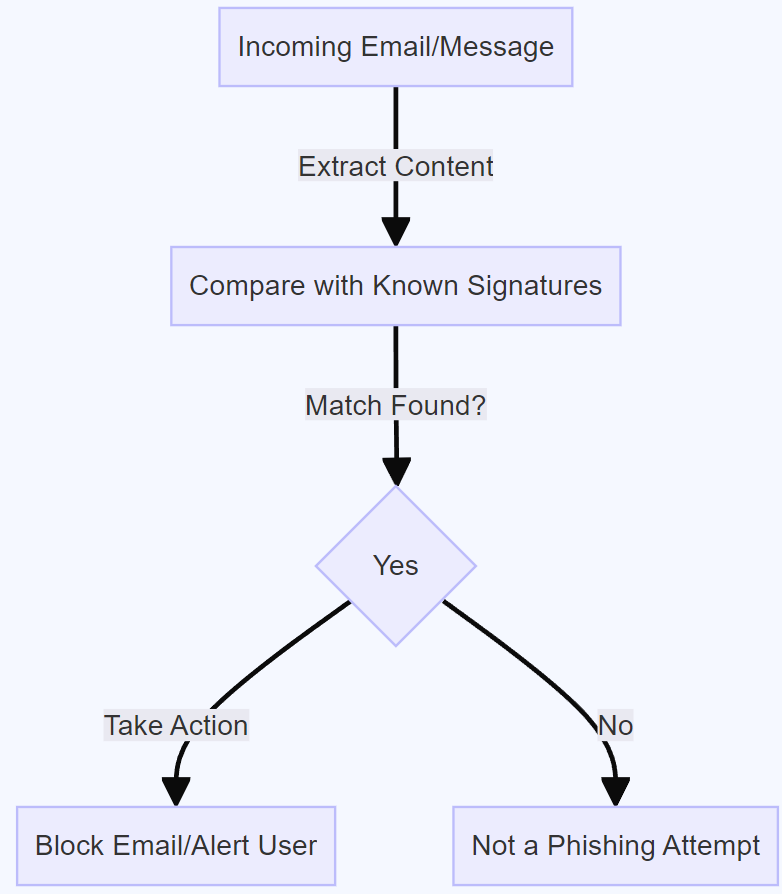
Advantages of Signature-Based Detection:
Speed and efficiency: One of the main advantages of signature recognition is speed and efficiency in known phishing schemes. By using pre-defined signatures, security systems can rapidly scan incoming emails and messages to identify and prevent abuse capture attempts in real time [4].
Accuracy: Signature-based detection provides greater accuracy when it comes to detecting known phishing attacks [2]. Because the signature is based on specific patterns and characteristics, there is little room for false positives, ensuring that emails that qualify as phishing attempts are not mistaken.
Resource efficiency: Signature-based registration is more economical because it requires less computing power to compare incoming emails with pre-defined signatures This functionality makes it suitable for use in assemblies and devices without significantly straining system resources [5].
Limitations of Signature-Based Detection:
Ineffective against new phishing attacks: The most important limitation of signature-based detection is that it cannot detect new or undiscovered phishing attacks because it relies on a database of known signatures, so it can’t detect phishing emails by patterns or other techniques. Cybercriminals are constantly evolving their tactics, trying sophisticated new attempts to catch people that identity-based systems can’t [6].
Dependency on Regular Updates: To remain relevant and effective, signature-based recognition systems require regular updates. Security professionals should constantly update the signature database as new phishing attacks emerge. Failure to store signatures makes the system vulnerable to changing phishing techniques, reducing its overall effectiveness [2].
Limited resources: Handwriting-based fonts focus only on email and message content. It does not consider other contextual factors, such as the behaviour of the sender or the response style of the receiver. This limited approach allows us to overlook subtle clues to entrapment attempts, especially spearhead attacks where cybercriminals tailor their messages to specific individuals or companies.
Conclusion:
While signature-based algorithms play an important role in early detection and prevention of known compromise attempts, it is important to be aware of its limitations As cybercriminals continue to refine their methods are the new fraud techniques, so relying solely on signature-based signatures is a complete defence against phishing attacks Giving is not enough To enhance cybersecurity measures, organizations must and individuals take a multi-layered approach, using advanced techniques such as behavioural analytics, machine learning algorithms, user education programs etc. Combining these strategies, we more robust protection against the ever-evolving landscape of phishing threats can be created, effectively protecting our digital communications and sensitive information.
Reference:
- Abdullah, R. S., Abdollah, M. F., Noh, Z. A. M., Mas’ Ud, M. Z., Selamat, S. R., & Yusof, R. (2013). Revealing the criterion on botnet detection technique. International Journal of Computer Science Issues (IJCSI), 10(2), 208.
- Belo, O., Mota, G., & Fernandes, J. (2016). A signature based method for fraud detection on e-commerce scenarios. In Analysis of Large and Complex Data (pp. 531-543). Springer International Publishing.
- Sihwail, R., Omar, K., & Ariffin, K. Z. (2018). A survey on malware analysis techniques: Static, dynamic, hybrid and memory analysis. Int. J. Adv. Sci. Eng. Inf. Technol, 8(4-2), 1662-1671.
- Zeidanloo, H. R., Shooshtari, M. J. Z., Amoli, P. V., Safari, M., & Zamani, M. (2010, July). A taxonomy of botnet detection techniques. In 2010 3rd International Conference on Computer Science and Information Technology (Vol. 2, pp. 158-162). IEEE.
- Khan, W. Z., Khan, M. K., Muhaya, F. T. B., Aalsalem, M. Y., & Chao, H. C. (2015). A comprehensive study of email spam botnet detection. IEEE Communications Surveys & Tutorials, 17(4), 2271-2295.
- Thakur, K., Ali, M. L., Obaidat, M. A., & Kamruzzaman, A. (2023). A Systematic Review on Deep-Learning-Based Phishing Email Detection. Electronics, 12(21), 4545.
- Wang, L., Li, L., Li, J., Li, J., Gupta, B. B., & Liu, X. (2018). Compressive sensing of medical images with confidentially homomorphic aggregations. IEEE Internet of Things Journal, 6(2), 1402-1409.
- Stergiou, C. L., Psannis, K. E., & Gupta, B. B. (2021). InFeMo: flexible big data management through a federated cloud system. ACM Transactions on Internet Technology (TOIT), 22(2), 1-22.
- Gupta, B. B., Perez, G. M., Agrawal, D. P., & Gupta, D. (2020). Handbook of computer networks and cyber security. Springer, 10, 978-3.
- Bhushan, K., & Gupta, B. B. (2017). Security challenges in cloud computing: state-of-art. International Journal of Big Data Intelligence, 4(2), 81-107.