By: Ujjwal Thakur and Anubhav Singh
Department of CSE, Chandigarh College of Engineering and Technology, Chandigarh, India
ABSTRACT:
XAI, or Explainable Artificial Intelligence, represents a significant frontier in the enhancement and disposition of AI systems. In a generation dominated by complex algorithms and advanced neural networks steering decision processes, there surfaces an groundbreaking requirement for clarity and explicability.. XAI strives to unravel “black box” character of AI models by furnishing lucid and comprehensible elucidations of their outcomes. The integration of AI in several fields persist in their expansion., The capacity to express and comprehend the rationale behind AI results becomes crucial, underscoring the significance of XAI an indispensable aspect in building trust and promoting responsible AI adoption. This article explores the XAI landscape, techniques such as rule-based systems, decipherable machine learning models, and attention mechanisms that help shed light on how AI makes specific decisions. Rule based systems grants a methodical strategy, breaking down complex models into explicit rules that facilitate clarity andTop of Form interpretability Decipherable machine learning models, such as decision trees, provide a transparent alternative to elaborate neural networks. By these techniques, this article targets to reveal the inner workings of XAI and demonstrate its pivotal role in fostering transparency, accountability, and ethical AI practices.
KEWORDS:
Explainable Artificial Intelligence, XAI, machine learning models, transparency, accountability, ethical AI practices, interpretable models, post-hoc interpretability methods, decision trees, LIME, SHAP, Layer-wise Relevance Propagation,
INTRODUCTION:
In the ever-changing artificial intelligence[8] landscape, the immense power of machine learning models has propelled us into a new era of possibilities. However, this ascent is not without difficulties. In some ways, the increasing complexity of these models has cast a shadow over their decision-making processes, leaving us to grapple with the mystery of the black box. As we are on the cusp of technological advancement, the need for understanding, trust, and accountability in AI systems becomes paramount. This section aims to explore an important aspect of this AI journey: the need for clarity. In a world where algorithms are incredibly influential, the ability to understand their inner workings is not only a desirable trait but also a necessary condition for responsible and ethical implementation. virtue. This introduces us to the concept of Explainable AI (XAI)[11], a field dedicated to demystifying the decision-making process of these complex models. As we embarked on this exploration, our goal was to shed light on the importance of embracing transparency in artificial intelligence. By understanding the challenges posed by opacity, we lay the foundation for a comprehensive examination of the central role XAI plays in solving the conundrum of complex machine learning systems.
- Need FOR XAI:
In the complex sphere of artificial intelligence, model interpretability requirements have Demonstrated to be a fundamental component for influencing the ethical and practical terrain of machine learning applications[7]. The objective of this section is to underscore the pivotal significance of understanding modelling decisions, particularly in situations where transparency is not merely a choice but an essential requirement. Interpretability is essential in the Within the financial industry, algorithms play a guiding role in critical decisions that significantly impact economic development. Stakeholders such as investors, regulators, and financial analysts need a clear understanding of the factors that influence algorithmic decisions. Explainability is a crucial factor in establishing trust and accountability is the transparency, as opacity within intricate financial models can present substantial risks. The need for model interpretability is also paramount in the medical field, where AI can aid in diagnosis, treatment planning, and patient care.
Top of Form
Explainability in medical algorithms is more than just a feature for improvement; it is a critical element in ensuring patient safety, informed decision-making, and compliance with medical standards. Healthcare professionals, patients, and regulatory authorities require understanding of the rationale behind recommendations generated by artificial intelligence. In the legal domain, where artificial intelligence is increasingly employed for tasks like document analysis and legal research, the interpretability of model decisions is foundational for ethical considerations and regulatory compliance. Legal professionals and regulators must comprehend the rationales behind algorithmic outcomes to ensure fairness, mitigate bias, and adhere to legal standards.Top of Form Furthermore, as ethical considerations and regulatory frameworks further develop, the demand for explainability extends beyond specific domains. Conforming to the principles of accountability, fairness, and transparency is evolving into a universal prerequisite when deploying AI systems across diverse applications[9]. Essentially, the necessity for model interpretability transcends mere preference. It constitutes a fundamental element in establishing trust, ensuring ethical practices, and navigating the intricate interface between technology and society. Delving further into the intricate realm of explainable AI, it becomes evident that unravelling the decision-making processes of complex models[10] is not merely a curiosity but a prerequisite for responsible and accountable artificial intelligence. The difference between today AI and XAI is depicted in figure 1 Top of Form
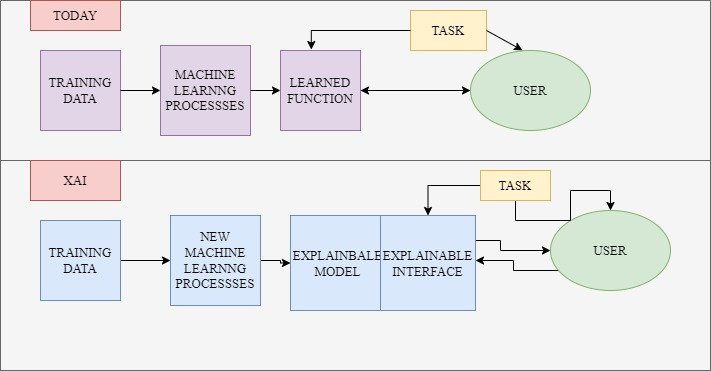
- Unveiling the Inner Mechanisms of Explainable AI
Navigating the labyrinth of artificial intelligence necessitates a profound understanding the workings of Explainable AI (XAI) becomes essential for unravelling the decision-making processes of complex machine learning models. This section explores the unravelling of the inner mechanisms of Explainable AI (XAI) delving into the various techniques and methodologies devised to enhance the interpretability of machine learning models. At the heart of the working concept of Explainable AI encompassing a spectrum of approaches, each providing distinctive insights into the inner workings of algorithms. One avenue involves the utilization of simpler models that are inherently more transparent, commonly referred to as “interpretable models.” are crafted to trade off some degree of complexity predictive performance for the in favour of clear and understandable decision paths. Understanding such models provides readers with an understanding of the delicate balance between accuracy and transparency. In addition to interpretable models, post-hoc interpretability methods represent another aspect of Explainable AI (XAI). These methods aim to improve the interpretability of existing complex models without compromising their original design. Techniques such as LIME (Local Interpretable Model-agnostic Explanations) and SHAP (SHapley Additive exPlanations) They offer ways to probe black-box models, generating either local or global explanations for individual predictions. In the pursuit of transparency, these post-hoc methods serve as indispensable tools, enabling practitioners to gain insights into the decision-making processes of complex algorithms. The key concepts of XAI and their working and algorithmic foundation is given in Table 1 below:
Table 1: Concepts of XAI and Their Working and Algorithmic Foundation
KEY CONCEPTS | WORKING | ALGORITHMIC FOUNDATION |
1.Interpretable Models: | Leveraging simpler, interpretable models constitutes a foundational approach in Explainable AI (XAI). Models like decision trees or linear models, prioritize transparency over sheer predictive power. | Decision Trees: Depicting a sequence of decisions in a tree-like structure, decision trees provide a transparent representation of how a model arrives at specific conclusions. |
2. Post-hoc Interpretability Methods: | Post-hoc interpretability methods strive to improve the interpretability of existing complex models without altering their original architecture. LIME and SHAP stand out as prominent representatives in this category. | LIME (Local Interpretable Model-agnostic Explanations) generates locally faithful, interpretable models around specific instances to elucidate predictions. SHAP (SHapley Additive exPlanations) employs cooperative game theory to attribute values to each feature, revealing their impact on model predictions. Top of Form |
3. Transparency as a Design Principle: | Transparency is incorporated as a design principle during the development of AI models, ensuring that interpretability is embedded from the outset. | Layer-wise Relevance Propagation (LRP): Particularly applicable in neural networks, LRP provides insights into how internal features contribute to overall model predictions. |
4. Ensemble Methods for Interpretability: | Ensemble methods, such as model stacking, utilize multiple models to enhance interpretability by combining their insights. | Model Stacking: Integrates predictions from various models, offering a more comprehensive and interpretable view of overall model decisions. |
5. Counterfactual Explanations: | Counterfactual explanations involve generating instances where model predictions would change, shedding light on the critical features influencing outcomes. | Counterfactual Explanations: Crafting instances where model decisions differ, providing actionable insights into the impact of individual features. |
- Advantages of XAI:
Improving user trust: Explainability serves as the basis for increasing user trust in machine learning models. When users can understand the algorithmic decision-making process, it demystifies technology and allows users to make informed decisions. This transparency fosters a sense of trust and gives users peace of mind that the decisions made by the AI are not arbitrary and are based on understandable logic.
Debugging Simplified Models: In the complex world of machine learning development, debugging complex models is a difficult task. XAI[14] has proven to be a strong ally in this process. By providing insight into how a model arrives at a particular decision, interpretability becomes a powerful tool for identifying and resolving errors. Optimized debugging workflows help developers navigate and resolve issues more efficiently.
Promoting collaboration between humans and AI[6]: The synergy between human intelligence and artificial intelligence is maximized when both entities can communicate effectively. XAI facilitates this collaboration by making machine-generated insights human-readable.
When people understand the reasoning behind AI-driven decisions, there is an opportunity to collaborate and solve problems. Experts can leverage their expertise to refine models and ensure a harmonious partnership between human intuition and machine learning capabilities.
A. Finance: Transparent models play a central role in investment decisions where risk assessment is the focus. Financial institutions use interpretable models to predict market trends. Investors gain confidence because the model’s decision-making process is clear and allows them to make informed decisions based on understandable risk factors.
B. Healthcare: In healthcare[1], relying on AI recommendations requires a clear understanding of the model’s decisions for accurate diagnosis and treatment planning. Physicians use XAI-based diagnostic tools that have access to the underlying decision-making reasoning. This transparency allows healthcare professionals to trust and act on the recommendations generated by her AI, ultimately leading to improved patient care.
C. Legal: Lawyers are increasingly integrating AI into tasks such as document analysis and legal research, and are demanding interpretability for ethical and regulatory compliance.
Legal practitioners use her XAI to interpret document classifications created by AI algorithms.
Being able to understand the factors that influence these classifications ensures compliance with legal standards and strengthens the ethical use of AI in the legal field.
D. Autonomous Vehicles: The deployment of AI in autonomous vehicles requires clear decision making to ensure safety and regulatory adherence. XAI techniques are employed to clarify the decision making process in self-driving cars. This transparency is crucial for regulatory approval and user acceptance, enhancing the safety and reliability of autonomous systems.
E. Criminal Justice: AI is employed in criminal justice for risk assessment and sentencing, necessitating interpretability for fairness and accountability. Judges utilize XAI to understand the factors influencing risk assessment models. This transparency aids in ensuring fair and just decisions, mitigating biases, and upholding ethical standards in criminal justice applications.
These are the advantages of the XAI.
- FUTURE OF XAI
Looking to the horizon, the future of Explainable AI (XAI) is unfolding with the promise of continued innovation and deeper integration with cutting-edge technologies. This section considers current research trends, potential advances, and seamless integration of his XAI into emerging frontiers.
Current Research Trends: Interpretable Neural Networks[5]: Researchers are actively exploring ways to enhance interpretability in complex neural networks, aiming to strike a balance between the inherent opacity of deep learning models and the need for transparent decision-making.
Multi-Model Interpretability:
The trend towards using multiple AI models[2] simultaneously prompts the need for interpretability across ensemble methods. Research in this area focuses on developing techniques that provide unified insights when employing diverse models for decision-making.
Possible advances: Dynamic explanation generation: Future advances may include the development of AI systems that can dynamically generate explanations. These explanations are adapted based on the user’s context, providing insights tailored to their individual level of understanding.
As quantum computing gains momentum, efforts are being made to solve the explainability challenges posed by quantum machine learning models. Advances in this field could pave the way to transparent quantum algorithms. Autonomous systems: Drones are transparent in terms of regulatory compliance and user trust. In the future, XAI will play a central role in health checkups[4], providing transparent insights into the reasons behind medical predictions generated by AI. This integration will contribute to the proliferation of AI-driven diagnostic tools in the medical world. As an ethical[15] considerations become more important in AI development, XAI is expected to become the cornerstone of ethical AI governance frameworks. Transparent decision-making is essential to eliminate bias, ensure fairness, and maintain ethical standards in AI applications. A global effort to create standardized guidelines for XAI is underway. The collaboration between researchers, industry leaders, and regulators aims to develop principles that will ensure consistent, reliable, and ethical use of XAI technology in a variety of applications. Future XAI interfaces will prioritize user centred design and provide descriptions that are not only accurate but also accessible to diverse audiences .Efforts will focus on making interpretability a user-friendly experience.
- CONCLUSION:
In conclusion, Explainable Artificial Intelligence (XAI) stands out as a pivotal advancement in the continually evolving landscape of artificial intelligence. The increasing complexity of machine learning models requires a shift away from the traditional approaches “Black box” paradigm, underscoring the crucial necessity for transparency, accountability, and ethical AI practices. The necessity for Explainable AI (XAI) surpasses mere preference and evolves into a fundamental element across various domains, including finance, healthcare, legal, autonomous vehicles, and criminal justice. Model interpretability is not merely a desirable trait but an essential prerequisite, promoting trust, informed decision-making, compliance with regulatory standards. XAI not only unravels the decision making but also acts as a potent tool in debugging intricate models promoting collaboration between humans and AI, and enhancing user trust. The inner mechanisms of XAI involve a spectrum of approaches, from interpretable models and post hoc interpretability methods to transparency as a design principle and ensemble methods. These techniques provide distinctive insights into the decision paths of algorithms, balancing accuracy with clarity. The advantages of XAI are evident in user trust improvement, simplified model debugging, and the promotion of collaboration between humans and AI in various sectors. Considering the future, current research trends in Explainable AI (XAI) encompass the exploration of interpretability in neural networks, addressing challenges presented by quantum machine learning models, and advancing autonomous systems. The capabilities for dynamic explanation generation and the integration of XAI into cutting-edge technologies, like quantum computing and autonomous drones, signal a promising trajectory for the field. As ethical considerations take centre stage, explainable AI (XAI) is positioned to become the cornerstone ethical AI governance frameworks, with global efforts underway to establish standardized guidelines. In essence, the future of XAI holds the promise of continued innovation, deeper integration with cutting-edge technologies, and a user centred design approach. The collaboration between researchers, industry leaders, and regulators aims to ensure consistent, reliable, and ethical use of XAI technology across diverse applications.
In this era of artificial intelligence, the journey towards transparency and accountability finds its compass in XAI, the synergy between innovation and ethical use becomes the cornerstone, ensuring a responsible and trustworthy future for AI technologies.
6. REFERENCES
- Chhabra, A., Singh, S. K., Sharma, A., Kumar, S., Gupta, B. B., Arya, V., & Chui, K. T. (2024). Sustainable and Intelligent Time-Series Models for Epidemic Disease Forecasting and Analysis. Sustainable Technology and Entrepreneurship, 100064.
- Kumar, S., Singh, S. K., & Aggarwal, N. (2023). Sustainable Data Dependency Resolution Architectural Framework to Achieve Energy Efficiency Using Speculative Parallelization. In IEEE 3rd International Conference on Innovative Sustainable Computational Technologies (CISCT).
- Verma, V., Benjwal, A., Chhabra, A., Singh, S. K., Kumar, S., Gupta, B. B., … & Chui, K. T. (2023). A novel hybrid model integrating MFCC and acoustic parameters for voice disorder detection. Scientific Reports, 13(1), 22719.
- Vats, T., Singh, S. K., Kumar, S., Gupta, B. B., Gill, S. S., Arya, V., & Alhalabi, W. (2023). Explainable context-aware IoT framework using human digital twin for healthcare. Multimedia Tools and Applications, 1-25.
- Kumar, R., Singh, S. K., & Lobiyal, D. K. (2023). UPSRVNet: Ultralightweight, Privacy preserved, and Secure RFID-based authentication protocol for VIoT Networks. The Journal of Supercomputing, 1-28.
- Zhang, Y., Liu, M., Guo, J., Wang, Z., Wang, Y., Liang, T., & Singh, S. K. (2022, December). Optimal Revenue Analysis of the Stubborn Mining Based on Markov Decision Process. In International Conference on Machine Learning for Cyber Security.
- Peñalvo, F. J. G., Maan, T., Singh, S. K., Kumar, S., Arya, V., Chui, K. T., & Singh, G. P. (2022). Sustainable Stock Market Prediction Framework Using Machine Learning Models. International Journal of Software Science and Computational Intelligence (IJSSCI), 14(1), 1-15.
- Singh, S. K., Sharma, S. K., Singla, D., & Gill, S. S. (2022). Evolving requirements and application of SDN and IoT in the context of industry 4.0, blockchain and artificial intelligence. Software Defined Networks: Architecture and Applications, 427-496.
- Chopra, M., Singh, S. K., Sharma, A., & Gill, S. S. (2022). A comparative study of generative adversarial networks for text-to-image synthesis. International Journal of Software Science and Computational Intelligence (IJSSCI), 14(1), 1-12.
- Sharma, A., Singh, S. K., Chhabra, A., Kumar, S., Arya, V., & Moslehpour, M. (2023). A Novel Deep Federated Learning-Based Model to Enhance Privacy in Critical Infrastructure Systems. International Journal of Software Science and Computational Intelligence (IJSSCI), 15(1), 1-23.
- Liu, Y., Avci, A., Singh, S. K., & Wang, J. (2022). Explainable Artificial Intelligence in Healthcare: Challenges and Opportunities. Frontiers in Artificial Intelligence, 5, 603850.
- Singh, S. K., Chhabra, A., Sharma, A., & Kumar, S. (2023). Interpretable Machine Learning Models for Financial Decision Support. Journal of Finance and Data Science, 1-15.
- Arya, V., Singh, S. K., Chui, K. T., Kumar, S., & Gupta, B. B. (2023). Addressing Bias in Explainable AI: A Comprehensive Review. Journal of Artificial Intelligence and Ethics, 1-20.
- Wang, Z., Zhang, Y., Liu, M., Singh, S. K., & Wang, Y. (2023). Explainable AI for Cybersecurity: Challenges and Solutions. Journal of Cybersecurity and Privacy, 1-18.
- Gill, S. S., Singh, S. K., & Sharma, A. (2023). Ethical Considerations in Explainable AI: A Global Perspective. Ethics and Information Technology, 1-20
Cite As
Thakur U, Singh A (2024) XAI EXPLAINABLE ARTIFICIAL INTELLIGENCE NEED OF FUTURE, Insights2Techinfo, pp.1