By: Dadapeer Agraharam Shaik, Department of Computer Science and Technology, Student of Computer Science and technology, Madanapalle Institute of Technology and Science, Angallu,517325, Andhra Pradesh.
Abstract:
The use of Artificial intelligence in cybersecurity is one of the most promising developments regarding the protection of digital assets from modern threats. In particular, this article is devoted to the interaction between AI and cybersecurity analyzing threat identification and response, as well as prognosis of potential risks. To some extent, AI provides capabilities such as the automated detection of anomalies, pattern recognition from big data, and the prediction of cyber threatening activities before the attacks. With new and different cyber threats emerging every other day, AI in security plays a crucial role of ensuring organizations are protected from such cognizant threats.
Keywords : AI, IT security, threats, ML, DL, big data, prediction, machine response, outliers.
1.Introduction
Being contemporary to cope with the digital transformation of business and people’s life, cybersecurity is an inevitable component of the world of technologies now. Internet of Things and big data concepts have exposed limitations of existing security technologies such as firewalls and anti-virus programs. Cybersecurity which is one of the main trends of the modern world is at the forefront of development thanks to a breakthrough technology like AI. AI deals with the complexities of large volumes of data and very quickly presents threat detection and shuts out the window of opportunity the cyber criminals may have for attacking. The incorporation of machine learning and deep learning in the cybersecurity environment has fostered the emergence of new complex systems for learning from the prior events, for the identification of the abnormality and for the prediction of the prospective threats with a high level of accuracy. Such a shift to AI in cybersecurity results not only in an increase in the effectiveness and speed of the actions taken by security systems and analysts, but also helps to introduce a proactive approach to threats. It can be concluded that the use of AI as a measure against constantly evolving cyber threats will only increase in the future, so incorporating AI into cybersecurity architectures is incredibly valuable.
2. Opportunities and challenges of AI in Cybersecurity
Machine learning is the foundation of the relatively new and rapidly developing cybersecurity applications covered throughout this paper. Said to be within, or a branch of, AI, has prompted the recent advances in a broad spectrum of tasks as image/voice recognition, speech synthesis, and self-driving. In other words machine learning systems can be thought of as systems which modify themselves in order to solve a particular problem. This process often begins with a clean slate that is a neural network. Developers of a specific system input data to a neural network, and an algorithm defines the formation of the network according to the data’s characteristics[1]. For instance, a system that identifies the features of malware will learn how to correctly classify a file as either as ‘malware’ or ‘benign’ and which specific pattern correlates to the classification. Over time, the network creates an abstract representation of what malware “looks like. ” An abundant quantity of high quality training data, good training algorithms, and powerful computing resources are needed for this process. Thus, the resulting machine learning model, in an ideal case, recognizes both known malware and new types of malicious programs yet unknown to the system. Continual improvement in the procedures of machine learning, means reasoning does not require human input for structuring data. Focusing on the model, the authors note that the given approach does not presuppose that an expert would teach the model what it should consider to determine malware, on the contrary, the model learns itself how it should analyze and classify it. Consequently, it may discover methods of identifying malware superior to addressing the opponent’s attempts at disguise – such as the “metamorphic” malware that modifies certain segments of code during replication[2].
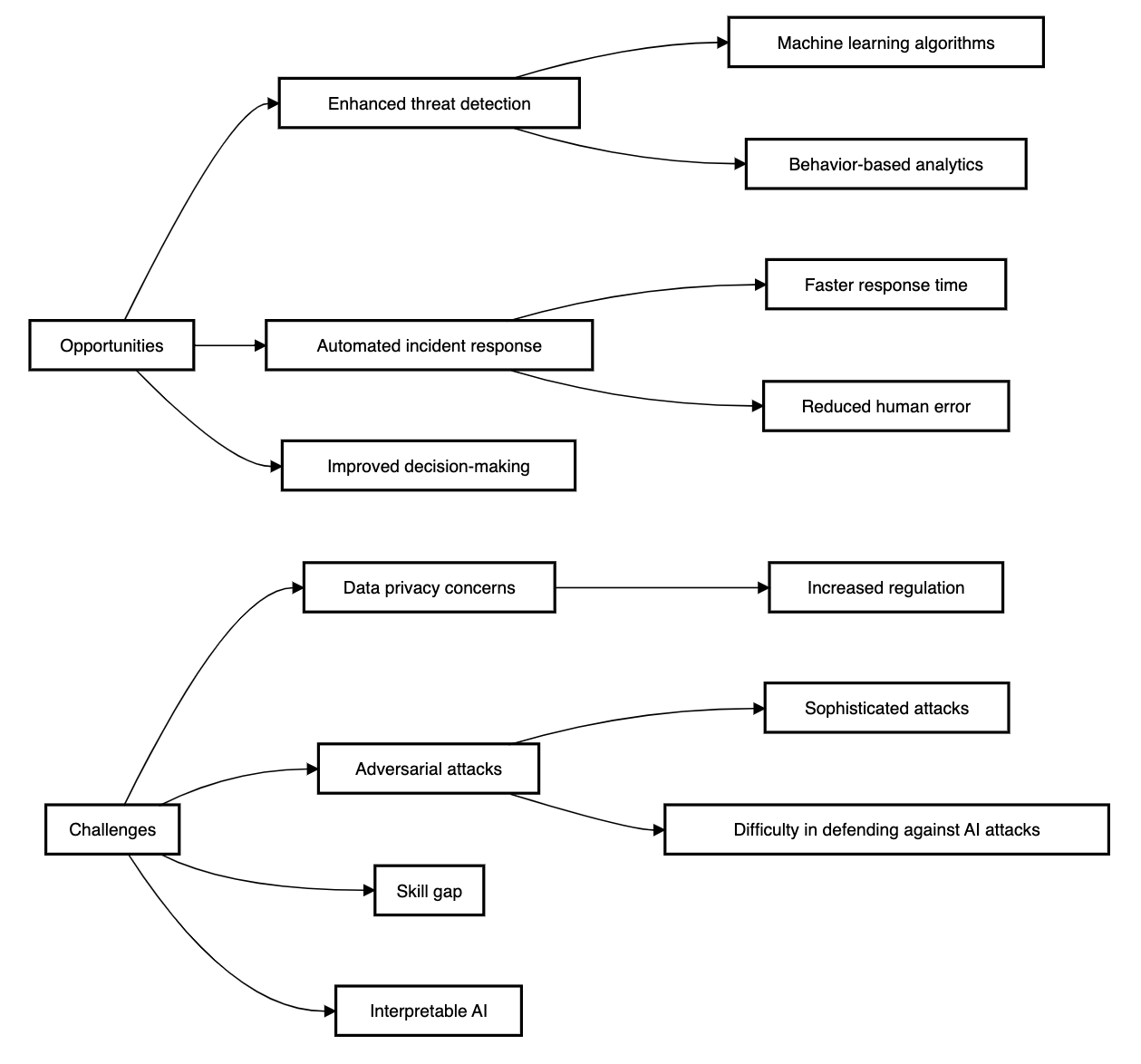
A possible application area that may also be influenced by machine learning is intrusion detection- identifying an adversary’s unauthorized presence in a friendly computer network. Current IDSs already try to search for signs, for instance when a computer wakes up at night or when a user tries to access files outside their working scope.
They are now in buzzwords in cybersecurity rapidly due to the increasing applicability of AI and machine learning. This makes it slightly challenging to determine how reliant clients are on these capabilities or when and how these capabilities are used to support marketing claims. It is rather popular for cybersecurity vendors to state that they use machine learning. For instance, CrowdStrike helps protect customers’ devices and networks and process data about approximately 250 billion events day in the production while providing machine learning models with new patterns of attacks. The company Darktrace suggests that in the so-called ‘Enterprise Immune System’, it uses several machine-learning approaches in order to enable system to counteract attacks. Machine learning has also been used for the following functions; checking for the presence of vulnerabilities in software programs, filtering spam and spear-phishing attacks, and the identification of suspicious behavior, as well as, insiders threats. In general, the examined ML systems seem to be used predominantly for comparatively specific and specialized purposes to augment the work of human Analysts’ responsible for defending InfoSec networks.
Consequently, the traditional ML techniques mainly defined for large training datasets, may not be sufficient for a system as one that performs more complicated steps that involve an action sequence; where each step depends on the result of the previous one. That is why such a system should learn more like a human and this means it has to experiment and make mistakes. This is the basics of reinforcement learning As we can see, the choice of actions does not depend on the environment a priori, but a specific action ensures the receipt of a better reward. In contrast to being given training data, a reinforcement learning agent participates in a simulated environment and is encouraged for action that helps its goal. It slowly begins to learn the sets of moves which are known as the “policies” of operation. The process can lead to terrific outcomes including the recent win of AlphaGo, a reinforcement learning system of DeepMind over Lee Sedol, the world champion in a complex game of Go. If reinforcement learning can solve it and come up with a solution and player that can beat the best chess masters, let alone Go masters and players, then there will be future cyber defenses that can learn and come up with new moves and strategies to counter and conquer the opponent and attackers in the “game”.
Contrary to what one will expect, cyber defenders now seem to have a home field advantage. [3]
3.Superseding Traditional Security Methods and Their Impediments
Current protection mechanisms use rules, which are signed and heuristic to identify and block cyber incidents. These methods are normally machine driven and are based on known threats and vulnerabilities, which call for frequent updates to the newer threats.
To be specific, one of the shortcomings of the classical approaches to the security of information systems is their inefficiency in terms of the flexibility of threats. Organized hackers are always improving their techniques in a way that is hard for the conventional measures to counteract them. This is a even more so the case in the case of zero-day attacks exploiting previously unknown vulnerabilities not identified by the security vendors. Also, traditional security measures are normally preventive which implies that they can only recognize the invasion after it has invading the system. This can, in turn, lead to heavy losses to the reputation, data, and pocket of an organization. Also, conventional security techniques produce many alerts that are false positives, which are time and money consuming to analyze. This can cause alert fatigue which entails security operatives receiving numerous alerts and as such cannot discern the real threat from the rest.
Finally, traditional security measures employed in organizations are based on the concept of protecting the outer perimeters of an organization’s network and systems. However, recent trends that involve more and more organizations shifting to cloud services and the popularity of using remote workers mean that the concept of perimeter is gradually fading, thus increasing the level of difficulty in protecting networks and systems.
In conclusion, the application of traditional approaches has numerous problems in terms of curtailing threats that are constantly emerging and are mostly applied in a reactive manner; they create a large number of false positives, and safeguard the ‘perimeter’, which is gradually becoming an ineffective approach in the modern world.[4]
Conclusion:
In conclusion, can be said that the application of AI in cybersecurity can dramatically change the world’s approach to threats and protection of electronic reserves. Thus, the growing competition in the global AI-powered security system market can be attributed to the fact that these systems employ machine learning algorithms to quickly discover threats, perform security activities autonomously, and minimize the possibility of human mistakes.
But like any other AI integration, there is always the dark side to its integration in security; some of these include opacity, biased manner and what else is ethical. These should be targeted out by developers and policy makers and it is important that AI security systems will be developed and used according to ethical and legal standards.
Taking into consideration potential future advancements and developments on the subject of artificial intelligence, one can presuppose further improvements in the sphere of AI security. This will entail sustained partnership between the industry and other partners including the government to ensure that the above technologies are deployed in the right manner that is both ethical and in ways that will allow the enhancement of security and protection of our digital resources in the future.
Reference:
- “Rahaman M, Chappu B, Chattopadhayay S, Anwar N, Adi N S, Prisilla A A, Haque A (2022) Implementation of Artificial Intelligence & Internet of Things (AI-IoT) its applications and challenges in Maritime Supply Chain Management, Data Science Insights Magazine, Insights2Techinfo, Volume 4/
- M. Rahaman, C.-Y. Lin, P. Pappachan, B. B. Gupta, and C.-H. Hsu, “Privacy-Centric AI and IoT Solutions for Smart Rural Farm Monitoring and Control,” Sensors, vol. 24, no. 13, Art. no. 13, Jan. 2024, doi: 10.3390/s24134157.
- S. Alam, “Cybersecurity: Past, Present and Future,” 2022, Accessed: Aug. 04, 2024. [Online]. Available: https://www.researchgate.net/profile/Shahid-Alam-3/publication/361765615_Cybersecurity_Past_Present_and_Future/links/65efe78baaf8d548dcc367cb/Cybersecurity-Past-Present-and-Future.pdf
- J. Bharadiya, “AI-Driven Security: How Machine Learning Will Shape the Future of Cybersecurity and Web 3.0,” Am. J. Neural Netw. Appl., vol. 9, pp. 1–7, Jun. 2023, doi: 10.11648/j.ajnna.20230901.11.
- Gupta, B. B., Perez, G. M., Agrawal, D. P., & Gupta, D. (2020). Handbook of computer networks and cyber security. Springer, 10, 978-3.
- Gupta, B. B., & Akhtar, T. (2017). A survey on smart power grid: frameworks, tools, security issues, and solutions. Annals of Telecommunications, 72, 517-549.
Cite As
Shaik D.A. (2024) AI and the Future of Cybersecurity, Insight2Techinfo, pp.1