By: Poojitha Nagishetti, Department of Computer Science and Engineering (Data Science), Student of Computer Science and Engineering (Data Science), Madanapalle Institute of Technology and Science, Angallu,517325, Andhra Pradesh. poojimurali2330@gmail.com
Abstract –
Cardiovascular Diseases are the leading cause of death globally: this explains why there is a call for early diagnosis of the diseases, and that treatment begins immediately to reduce their incidence. In general mastering of the Artificial Intelligence (AI) has proven useful in the healthcare sector, particularly when it comes to smart tools and approaches of estimating and diagnosing CVDs. The authors of this article discuss about the uses of Artificial Intelligence in prediction of cardiovascular diseases and points toward the Machine Learning as well as the Deep Learning. In this section, we cover various datasets and feature engineering methods employed in this domain and the kinds of predictive models. Additionally, we discuss the limitations and potential of AI advancement to greatly enhance the prediction of CVD with future directions presented, so the reader will have a broad perspective of the current situation and the possible future developments of AI-assisted cardiovascular medicine.
Keywords – Artificial Intelligence, Cardiovascular Disease, Predictive modeling, Data Integration
Introduction –
Cardiovascular diseases also known as CVDs are diseases of the heart and blood vessels that include for instance coronary artery diseases, heart failure, and irregular heartbeat. These diseases are the major causes of mortality in the world, contributing to about 17 percent in general. 700,000 persons daily and 2,327,913 persons weekly; a total of 9 million deaths per year, as per WHO. The mortality and morbidity of CVDs are still very high, and this has emphasized the importance of proper screening, risk factor assessment and use of efficient health resources in the management of CVDs to cut down the burden on the health system.
The conventional techniques of diagnosing and prognosis of CVD involve basic examinations on the patient, history and other tests like electrocardiogram and echocardiogram test, blood tests etc. Although these methods shed light on the outcome, they may be slow, costly and on some occasions, biased. However, the amount of data collected by these tests’ present problems of how clinical practitioners can analyze and accurately, let alone efficiently, interpret.
In the recent past, the development of IT in healthcare has been embraced and the enhancement of Artificial Intelligence has the potential of handling these hurdles. The application of artificial intelligence (AI) to the electrocardiogram (ECG), a ubiquitous and standardized test, is an example of the ongoing transformative effect of AI on cardiovascular medicine[1]. Although the ECG has long offered valuable insights into cardiac and non-cardiac health and disease, its interpretation requires considerable human expertise. Advanced AI methods, such as deep-learning convolutional neural networks, have enabled rapid, human-like interpretation of the ECG, while signals and patterns largely unrecognizable to human interpreters can be detected by multilayer AI networks with precision, making the ECG a powerful, non-invasive biomarker. Large sets of digital ECGs linked to rich clinical data have been used to develop AI models for the detection of left ventricular dysfunction, silent (previously undocumented and asymptomatic) atrial fibrillation and hypertrophic cardiomyopathy, as well as the determination of a person’s age, sex, and race, among other phenotypes[1]. The clinical and population-level implications of AI-based ECG phenotyping continue to emerge, particularly with the rapid rise in the availability of mobile and wearable ECG technologies. In this Review, we summarize the current and future state of the AI-enhanced ECG in the detection of cardiovascular disease in at-risk populations, discuss its implications for clinical decision-making in patients with cardiovascular disease and critically appraise potential limitations and [2]. The usage of ML and DL algorithms within AI-based systems provides innovative tools for processing big volumes of data and defining concealed relationships or making correct prognoses. These systems can complement the routine diagnostic approaches to offer the clinicians well-developed decision-support tools for successful diagnosis and prognosis of CVDs.
This article focuses on the current situation regarding the creation and utilization of AI-based systems to forecast cardiovascular diseases by describing the data sets, pre-processing methods, and models in this area. Under this category, we will be more specific on the kinds of Machine learning and deep learning models, their uses, strengths, and challenges. Further, we will describe some examples of AI success in terms of its ability to CVD prediction, and the limitations, prospects, and further development of this rapidly emerging field. Figure 1 describes the workflow of CVD prediction.
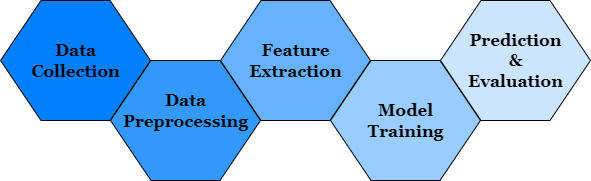
Causes of Cardiovascular Disease –
CVDs are a multifactorial disorder and are influenced risks factors such as genetics, lifestyle, physiological, environmental and health status of a person, age, and gender. Hereditary factors that include family history of CVDs and congenital diseases like; Abetalipoproteinemia, Familial hypercholesterolemia, and other genetic defects are contributing factors to the risk factors[3]. Modified diet Asian diet which is characterized by high intake of saturated fats and sodium, little exercise, smoking, and excessive alcohol consumption all play a major role in the development of CVDs. Hypertension, hyperlipidemia, and diabetes which are type 2 physiological disorders of the body significantly increase chances by virtue of directly influencing the cardiovascular system.
Other added lifestyle and pollution related risk factors include acute exposure to air pollution and stress that comes with lower Socio-economic statues weaken the ability of an individual not to develop CVDs. Certain illnesses include obesity, chronic kidney disease, chronic inflammatory diseases, and more also increase the risks of heart diseases. Also, there is an increase in number and prevalence of CVDs in elderly people because of the aging effects and accumulation of risk factors in the cardiovascular apparatus. Risk is also trigged by gender where men are more likely to be at high risk at an early age more than women but the risk of the latter surges after menopause. Stress and other mental health problems, such as depressive and anxiety disorders, increase the probability of CVD as results from psychological influence on the lifestyle and physiological function. It is therefore important to recognize these diverse characteristics of the causes if prevention and control measures and the treatment of cardiovascular diseases are to be optimized.
Emergence of AI in CVD prediction –
The application of AI in CVD is relatively new and has recently gained popularity by Big Data, computational ability, and novel ML and DL techniques. Previously, in managing CVD, clinical symptoms were diagnosed without complete reliance on objective test results, mainly due to the limited resources at the clinicians’ disposal[4]. Digitization of health records and machines that can be worn also have created big data that AI can analyze and determining the contradictions that cannot be noticed through normal observation. Simple and advanced ML techniques have offered better chances of creating more accurate predictive models; changes in DL such as CNNs and RNNs have helped improve the results of medical imaging and sequential data such as ECGs, respectively. These developmental milestones have made it possible to diagnose, manage and monitor CVDs in their early stages hence changing the entire complexion of cardiac care and enhancing patient’s well-being.
Use of AI in predicting CVD –
The analyses reveal that AI has been useful in estimating cardiovascular diseases with additions in precision, velocity, and diagnosis. The integration of AI into cardiovascular healthcare can be seen through several key applications and methodologies[5]: Based on previous section, it can be identified that the applications and approaches in cardiovascular healthcare using AI are as following.
Data Analysis and Pattern Recognition:
Machine learning and deep learning algorithms are used for the analysis of large clinical data sets to detect sophisticated patterns associated with a particular disease that cannot be easily recognized using normal statistical mechanics. This capability enables scoped schemas for defining anticipations of CVD risk factors and plausible results depending on various factors such as patient’s demographic data, prior medical history, habitual behaviors, and genetics.
Predictive Modeling:
The current AI solutions can help in evaluating the risk of the future CVD events, including acute myocardial infarctions, strokes, or heart failure. These models are developed from large data sets that consist of different health indices to give risk profiling. Another feature of artificial intelligence is that, unlike most other models, AI models can revisit the collected data and refine the prediction models.
Early Detection:
AI systems can identify indicators of CVDs before the manifestation of conspicuous symptoms that could be observed in physical examinations. For instance, AI can be used to read ECGs and identify some rhythmic, or other, cardiac disturbances at their preliminary stages. Recognizing them at an early stage signifies those interferences can be made, thus avert the advancement of CVDs.
Medical Imaging Analysis:
AI, particularly the deep learning method therefore embracing the convolutional neural networks, is very efficient in analyzing medical images including the echocardiograms, MRI scans, as well as the CT scans. These AI systems can pinpoint structural along with functional flaws in the heart along with blood vessels, and so can help in the analysis and review of ailments likes CVD, CMP as well as VHD.
Personalized Treatment Plans:
One of the beneficial effects of AI is that through the effectual utilization of patients’ characteristics analysis, treatment strategies aligned with each patient’s risk profile can be created. Such an approach might enhance the prescription of medications, diet and other aspects of the patient’s life, and the methods of observing the patient, which will ultimately improve patients’ lives. Figure 2 shows the impact on CVD management.
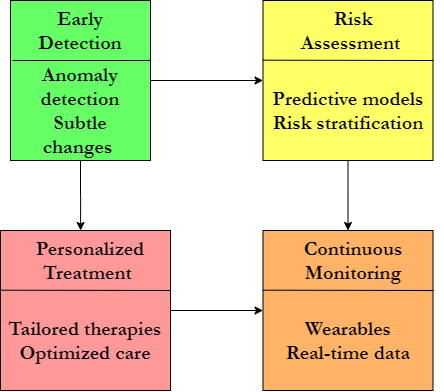
Future Directions –
While AI has greatly helped in CVD management, some issues still arise in everyday practice. The procurement and quality of the data are the main issues, as obtaining a wide variety of high-quality data is required to create reliable AI systems[5]. Another is interpretability of AI models and more so deep learning algorithms whose working mechanism is rather hard for a clinician to understand and hence give his or her trust[6]. AI systems and solutions being embedded into real working clinical environments must be done in close cooperation with both AI scientists and clinician end users. Moreover, the social and legal concerns, like protection of patients’ data, handling of prejudices within artificial intelligence tools, and meeting applicable legal requirements, become vital for the proper use of artificial intelligence in the healthcare industry[7]. As for the future of AI-based CVD prediction systems, there are still improvements in the AI algorithms, computational processing power, and data gathering methods that are still possible in the future. One must continue to build XAI methodologies that can help overcome the current difficulties, as well as, to promote interdisciplinary cooperation for strengthening AI application in cardiovascular healthcare.
Conclusion –
Thus, the implementation of AI as a part of CVD strategy is a categorical breakthrough in the sphere of medical science. There has been a tremendous improvement in the detection of CVD risks, preliminary diagnosis, and strategies of management since dawn of use of AI in health care especially in the application of machine learning and deep learning to extensive clinical data. Such technological development breaks down the rates of diagnosis and time elapsed before the necessary treatment is provided thus promoting patient success and efficiency in resource use. However, there are still issues to do with data quality, interpretability of the model, and how to incorporate the application into clinical practice. Overcoming these challenges with further research, interdisciplinary cooperation, and ethical implications will be of vital importance for the proper AI-driven solutions’ application. With technology advancing, AI provides the possibility of further developments in cardiovascular healthcare and the possibility of enhancing cardiovascular diseases’ prevention, diagnosis, and treatment in the future.
References –
- C. Van Mieghem, M. Sabbe, and D. Knockaert, “The Clinical Value of the ECG in Noncardiac Conditions,” Chest, vol. 125, no. 4, pp. 1561–1576, Apr. 2004, doi: 10.1378/chest.125.4.1561.
- D. Lee and S. N. Yoon, “Application of Artificial Intelligence-Based Technologies in the Healthcare Industry: Opportunities and Challenges,” Int. J. Environ. Res. Public. Health, vol. 18, no. 1, Art. no. 1, Jan. 2021, doi: 10.3390/ijerph18010271.
- X. Zhan, M. Humbert-Droz, P. Mukherjee, and O. Gevaert, “Structuring clinical text with AI: Old versus new natural language processing techniques evaluated on eight common cardiovascular diseases,” Patterns, vol. 2, no. 7, Jul. 2021, doi: 10.1016/j.patter.2021.100289.
- L. Triyono, – Prayitno, M. Rahaman, – Sukamto, and A. Yobioktabera, “Smartphone-based Indoor Navigation for Guidance in Finding Location Buildings Using Measured WiFi-RSSI,” JOIV Int. J. Inform. Vis., vol. 6, no. 4, pp. 829–834, Dec. 2022, doi: 10.30630/joiv.6.4.1528.
- K. C. Siontis, P. A. Noseworthy, Z. I. Attia, and P. A. Friedman, “Artificial intelligence-enhanced electrocardiography in cardiovascular disease management,” Nat. Rev. Cardiol., vol. 18, no. 7, pp. 465–478, Jul. 2021, doi: 10.1038/s41569-020-00503-2.
- P. Pappachan, Sreerakuvandana, and M. Rahaman, “Conceptualising the Role of Intellectual Property and Ethical Behaviour in Artificial Intelligence,” in Handbook of Research on AI and ML for Intelligent Machines and Systems, IGI Global, 2024, pp. 1–26. doi: 10.4018/978-1-6684-9999-3.ch001.
- A. J. Fathima and M. M. N. Fasla, “A comprehensive review on heart disease prognostication using different artificial intelligence algorithms,” Comput. Methods Biomech. Biomed. Engin., vol. 27, no. 11, pp. 1357–1374, Aug. 2024, doi: 10.1080/10255842.2024.2319706.
- Sharma, A., Singh, S. K., Kumar, S., Preet, M., Gupta, B. B., Arya, V., & Chui, K. T. (2024, January). Revolutionizing Healthcare Systems: Synergistic Multimodal Ensemble Learning & Knowledge Transfer for Lung Cancer Delineation & Taxonomy. In 2024 IEEE International Conference on Consumer Electronics (ICCE) (pp. 1-6). IEEE.
- Chui, K. T., Gupta, B. B., Jhaveri, R. H., Chi, H. R., Arya, V., Almomani, A., & Nauman, A. (2023). Multiround transfer learning and modified generative adversarial network for lung cancer detection. International Journal of Intelligent Systems, 2023(1), 6376275.
Cite As
Nagishetti P. (2024) AI-Based Systems for Predicting Cardiovascular Diseases, Insights2Techinfo, pp.1