By: Dhanush Reddy Chinthaparthy Reddy, Department of Computer Science and Artificial Intelligence, Madanapalle Institute of Technology and Science, Angallu(517325), Andhra Pradesh
Abstract
The use of Artificial intelligence or AI in security has enhanced security in organizations in their pursuits of ensuring their assets are secure. With the advancement of threats in the cyberspace, artificial intelligence can be employed in developing preventive and adaptive security measures in the networks. This paper aims at outlining how use of artificial intelligence in network security is used especially in threat identification, reaction, and prognosis. Using examples of the existing practices and the current state of AI, this paper shows how AI strengthens the security in a network, minimizes the vulnerability to cyber threats, and constitutes a solid shield against a fast-growing threat environment. The challenges that reflect the discussion are also listed: quality data used for AI’s working, biases that might exist within AI models, and the role of humans in AI-run cybersecurity operations. The study reinforces its importance in protection of networks and made a call to further develop as well as advance efforts in AI.
Keywords: Security, Networks, Artificial Intelligence
Introduction
Cyber threats are getting more complex and widespread and using traditional security measures cannot longer address the issues of vulnerability in the networks. Artificial Intelligence being the new age technology has become a reference of change in terms of risk management focusing on the defence of computer systems from cyber threats. This paper focuses on understanding the full potential of AI in securing the networks, and therefore aims at offering more thorough analysis of the way in which AI is changing the current landscape of cybersecurity. The paper starts analysing AI for real-time threat detection stating its strength in identifying threats including the unknown ones given the strength of machine learning algorithms in patter and anomaly detection. This is followed by the analysis of the application of AI in automating response mechanisms, which are the actions that can be triggered when a certain threat is identified and which can eliminate the threat within a matter of seconds and thus drastically decrease the time frame available for the attacker to operate under, all this without human interaction. Discussed herein is also potential of artificial intelligence in terms of predictive analysis: as it can predict future cyber threats by analysing existing data and trends and preparing the organizations for its protection. Finally, the paper shows that through two case studies the practical advantages and concerns connected with the utilization of AI for networks security can be presented successfully and proving existing effective usage of the concept, but at the same time underlining some issues that were met during the integration. The discussion broadens to the problematics of AI implementation, which include the problem of data quality as well as the problem of algorithm bias and the emergent problem of AI ethics in cybersecurity. As for the further development of the paper, the conclusion is dedicated to emphasizing the significance of AI for the future of network security, to call for the permanent improvement, coordination, and cautious handling of the ethical aspects to ensure AI will become the major tool for maintaining digital infrastructures.
AI-Driven Threat Detection
AI threat is another major advance in the era of cybersecurity compared to conventional threat detection and protection solutions since it is prompt and therefore more effective. The problem with the conventional security models is that they are based on ‘’signature-based models’’ that only identify threats from a rigid set of rules and patterns. But the problem here is that these such methods are not very useful in detecting new, unknown, or emerging threats [1]. AI on the other hand, deals with Threat detection through machine learning by utilizing network traffics and users’ performance analytics to unveil bizarre activities of threat outfits. These algorithms possess unique ability to learn from the past events and enhance their performance by considering the new material, which makes them very useful in detecting both, known and novel threats[2]. For instance, AI systems can establish that there are certain changes in the behaviour of a certain network that may indicate that a particular device has been compromised or that there is a slow evolving attack, which may not be obvious to human analysts. Further, the AI-based threat detection can work on automation, synching with the limit of capability significantly higher than human, and take data through a real-time approach while simultaneously giving alerts and measures to consider in terms of threats. This not only augments the general security framework of an organization but also provides the cybersecurity teams the ability to prioritize and do proper analysis of the most significant threats while [3]avoiding the fakeness of the alarms as much as possible so that resources are properly utilized.
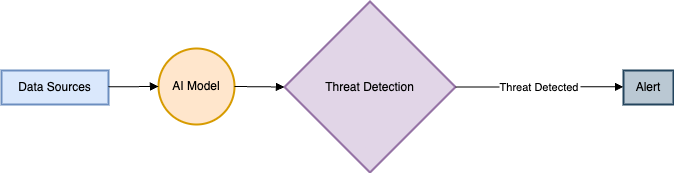
Automated Response to Cyber Threats
Cyber threat mitigation is a key element within present-day protection schemes that rely on artificial intelligence to rapidly perform well-defined responses as soon as there is a possibility of encountering a threat. This capability becomes helpful today’s world where a window of opportunity to the attacker can range from minutes to seconds. AI-based systems can respond to events like malware infection, attempted unauthorized access, or data leakage by sub jecting the diseased systems, blacklisting IPs or applying patches without human inputs. This immediate response not only helps to stop the further expansion of the malicious activity in the network but also contributes to the reduction of the time needed to stop the threats hence sparing the network from further losses. This way, many repetitive and time-demanding activities in the sphere of security can be revealed to be addressed to specific algorithms, freeing the experts for more important and challenging problems. Further, the learning capability of AI makes sure that these automated responses become more accurate and hence help to enhance the security position. Nevertheless, as automation adds to effectiveness it requires precise setting up and supervision to prevent some negative effects like over-blocking or getting false positives that would interfere with other lawful conduct.
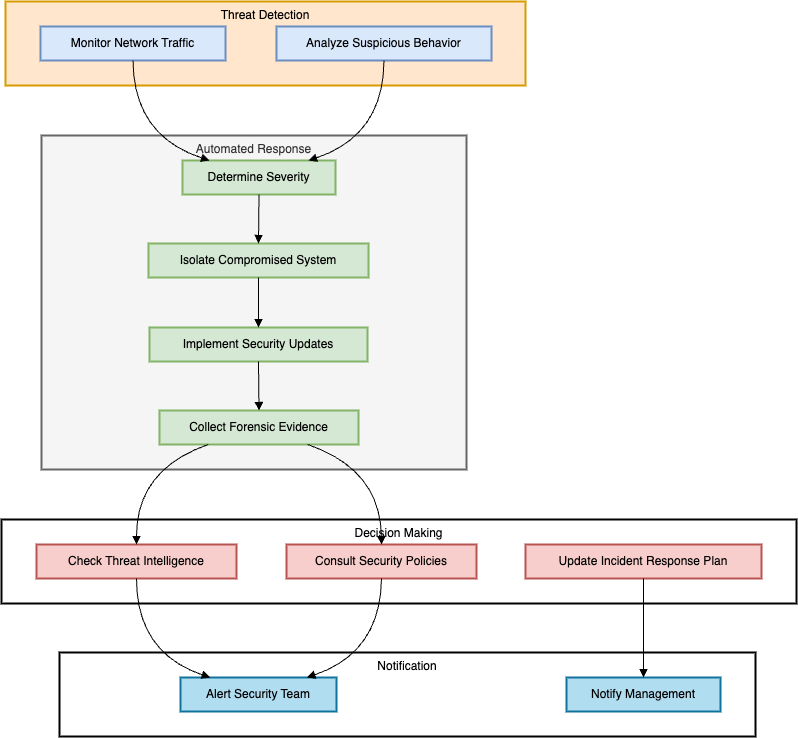
Predictive Analytics for Network Security
One of the ways in which the use of AI in organizations is making a difference in the practice of network security is by making predictive analytics possible. As opposed to other reactive methodologies, predictive concern leverages artificial intelligence to analyse small historical and future attack and forecast information and prepare for them. By analysing the activity flow and user behaviour within the network, the indicators of new attacks can be identified, such as frequent phishing attempts or any specific login pattern and estimate the likelihood of various threats in specific time and space zones. Such visions help strengthen weak links, find ways to optimally distribute and allocate infrastructure and capital, apply fixable hazards to security before they occur, and so on[4]. For instance, predictive analytics is used to ensure that patching of gaps in software is done in an efficient manner by using past attack data to determine those that are more prone to be attacked. It also increases the robustness of the networks while fading the possibility of a successful attack. This is even more powerful in networks since as more data is fed to it and it is trained to incorporate it as knowledge to be used in predicting, it can adapt to the modern world’s ever-changing cyber threats by constantly learning from new data inputs. However, to be realized, predictive analytics rely more on the quality of the data and the AI models, which leads to data quality and model calibration as critical success factors.
Case Studies: AI in Network Security
Over time, incorporating AI in network security has been proven to be quite beneficial across the different sectors, as will be discussed through some of the specific examples. For example, a large banking organisation implemented AI-based threat identification as well as the use of autonomous response measures into its security plan. This immediately reduced response time to potential threats from several hours to mere minutes and helped the organization to avoid data breach incidents that, if exploited, could cost the organisation a great deal of money and its reputation. An example is a global technology company that uses AI based predictive analysis to prevent DDoS or distributed denial of service attacks. Thanks to traffic analysis and detection of the signs of an attack, the AI system was able to call the buffers in before the attack could progress to a dangerous level and millions of users remained unaffected. [5]Also, the MedCords turned to AI as board members put the healthcare provider’s network at risk of ransomware by accessing electronic health record systems. Due to the AI, the provider was able to identify anomalous activities for example unauthorized data access or file encryption and was then able to quarantine those systems that had been compromised thus limiting the risk of massive system disruption.[6] These examples also demonstrate that AI while increasing the security of networks’ performance also has a broad potential that can be implemented depending on the line of business. But they also reveal the problems with the implementation, for instance, the need for quality data, the compatibility of AI with the rest of the system architecture, and the constant need to improve the model to counter new threats.
Conclusion
The paper has reviewed several case studies that illustrate a massive transformation of network security using Artificial Intelligence. In the financial sector, technology companies and healthcare organizations, AI solutions have improved the possibility to identify, counteract, and forecast cyber threats that protect vital digital assets. These real-life cases highlight the real value of incorporating AI in protecting organizations assets, processes and individuals by generating a much faster response time, increased accuracy in threat detection and the ability to prevent attacks before they can occur. However, the integration of AI in network security has its complexity level thus has it forms challenges. Evaluating the quality of data, an ability to integrate the AI-driven security into the existing processes, and improving models based on the changing threats are among the critical aspects of the security strategy. With new and more serious threats being identified daily, the future and continuous use of AI will remain necessary to securing networks and other technology systems. As for the AI’s role in the future of cybersecurity, it means that cybersecurity will rely on AI, but it will remain an auxiliary rather than a primary mean due to the constant evolution of the threats, the necessity for upgrades in technologies, and the necessity for close cooperation between the branches of industries.
References
- D. V. V. Vegesna, “Enhancing Cybersecurity Through AI-Powered Solutions: A Comprehensive Research Analysis,” Int. Meridian J., vol. 5, no. 5, Art. no. 5, Dec. 2023.
- “Proactive Cyber Defense: Utilizing AI for Early Threat Detection and Risk Assessment | International Journal of Advanced Engineering Technologies and Innovations.” Accessed: Aug. 13, 2024. [Online]. Available: https://ijaeti.com/index.php/Journal/article/view/321
- B. R. Maddireddy and B. R. Maddireddy, “Proactive Cyber Defense: Utilizing AI for Early Threat Detection and Risk Assessment,” Int. J. Adv. Eng. Technol. Innov., vol. 1, no. 2, Art. no. 2, Dec. 2020.
- E. Unal, S. Sen-Baidya, and R. Hewett, “Towards Prediction of Security Attacks on Software Defined Networks: A Big Data Analytic Approach,” in 2018 IEEE International Conference on Big Data (Big Data), Dec. 2018, pp. 4582–4588. doi: 10.1109/BigData.2018.8622524.
- M. Rahaman et al., “Utilizing Random Forest Algorithm for Sentiment Prediction Based on Twitter Data,” 2022, pp. 446–456. doi: 10.2991/978-94-6463-084-8_37.
- M. Rahaman, C.-Y. Lin, and M. Moslehpour, “SAPD: Secure Authentication Protocol Development for Smart Healthcare Management Using IoT,” Oct. 2023, pp. 1014–1018. doi: 10.1109/GCCE59613.2023.10315475.
- Mourelle, L. M. (2022). Robotics and AI for Cybersecurity and Critical Infrastructure in Smart Cities. N. Nedjah, A. A. Abd El-Latif, & B. B. Gupta (Eds.). Springer.
- Keesari, T., Goyal, M. K., Gupta, B., Kumar, N., Roy, A., Sinha, U. K., … & Goyal, R. K. (2021). Big data and environmental sustainability based integrated framework for isotope hydrology applications in India. Environmental Technology & Innovation, 24, 101889.
- A. Gaurav, B. B. Gupta, C. -H. Hsu, D. Peraković and F. J. García Peñalvo, “Deep Learning Based Approach for Secure Web of Things (WoT),” 2021 IEEE International Conference on Communications Workshops (ICC Workshops), Montreal, QC, Canada, 2021, pp. 1-6, doi: 10.1109/ICCWorkshops50388.2021.9473677.
Cite As
Reddy D.R.C. (2024) AI in Action: Securing Our Networks, Insights2Techinfo, pp.1