By: Indu Eswar Shivani Nayuni, Department Of Computer Science & Engineering(Data Science), Student Of Computer Science & Engineering(Data Science) ,Madanapalle Institute of Technology and Science, Angallu(517325),Andhra Pradesh . indunayuni1607@gmail.com
Abstract
Generative AI is turning into a revolutionizing force in achieving the maximization of profit by providing new instruments and approaches other than data processing. The following areas are discussed in this article as to how generative AI is applied in increasing the profit through knowing what comes next, in real-time price control, and the resourceful data that is generated by an AI system about the potential of innovative products and services. According to different lines of generative AI, companies benefit from higher quality of customer profiles and higher targeting selection, supply chains and procedural strategies, and new approaches to the constructive creation of finance plans and budgeting.
The article also good uses cases, which show that generative AI solutions are useful to enhance the top line and productivity by a multiplier. However, the use of generative AI also entails risks that are the breach of data privacy, the issue of the AI algorithm’s fairness, and the challenge of integrating AI into organizational structures. Since the article has mentioned that artificial intelligence has its ethics, improper usage would require that certain measures are followed then the article continues to explain the ethics and precautions that must be taken. In the future, generative AI has the potential of further development and invasion of all spheres and business areas as the new paradigm of profit, setting up AI as the base component of digital business success.
Keywords: revolutionizing force, maximization of profit, potential of innovative products, higher quality of customer profiles, higher targeting selection, supply chains, algorithmic fairness, paradigm of profit.
Introduction
In the contemporary world full of constant changes in the business environment and increased competitiveness, the question of the maximization of profit has turned into one of the most crucial. Big organizations are therefore always looking for strategies for getting the most out of their profits and minimizing expenditures or being ahead of rivals in such markets. Many conventional strategies are focused on finding the maximum profit and are based on the analysis of historical data and mathematical models. Albeit useful to certain extent, such approaches may find it difficult to cope with the modern markets and its challenges. [1]
Welcome to the generative AI— a next generation technology that is not only analytical and predictive but also generative in generating new data, new strategies, and new solutions. While generative AI differs from other AI concepts in that it identifies patterns within data, it uses algorithms to create new outputs that can be employed to enhance many areas of a company’s operation, including product offerings, promotions, and supply chain systems.
Applications of generative AI in profit optimization make it possible for business organizations to enhance forward-looking and creative solutions beyond what is reactive. When generative AI comes up with new ideas and proposes solutions, it assists organizations in being one step ahead of the market shift, innovating proficiently, as well as ensuring that their products and services align with the customers’ needs more closely. [2]
In this article the author discusses various possibilities of using generative AI in profit enhancement. We shall discuss how AI can be implemented to improve the dynamism in the pricing systems, product and service differentiation, customer categorization, and efficient supply network. Also, there will be an analysis of case scenarios regarding the application of generative AI and the challenges related to its implementation and the corresponding ethical issues. Last but not least we will discuss the future trends and the idea of how generative AI can further transform the concept of profit optimization to help various businesses prepare for the future in the ever-evolving competitive market influenced by the availability of data.
Functions of Generative AI and its applications in the enhancement of profits
As the offshoot of the harvest from the seed of patent technology which is the generative AI, it has the potential to embody superior usages in the form of new concepts and information out of the existing profit making strategies. [3]It can therefore be used in enhancing several operational parameters of business which leads to increased revenues, reduced costs and an overall enhancement of the competitive position of the business. Below are key applications of generitive AI in profit optimization:The following is a breakdown of generitive AI uses in improving the amount of profits:
The diagrams represents the functions of gen ai and it’s applications in the enhancement of profits
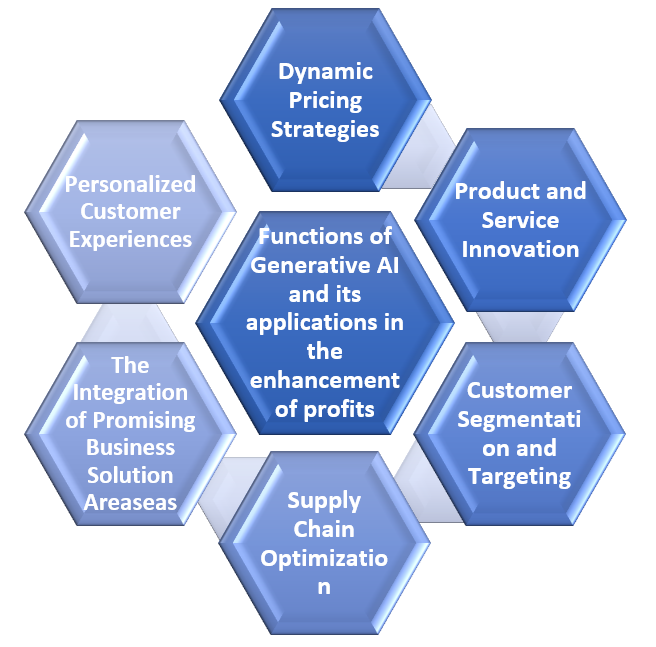
1. Dynamic Pricing Strategies
Description: In contrast to the decision-making AI, generative AI is applied for other market data including competitors’ prices, demand and consumers and sets up the real-time pricing for products.
Benefits:
- Maximized Revenue: In this way, by establishing the prices variable dependant on the current conditions, firms could satisfy more clients in high-demand and bring balance with average, typical sales and the prices in low-demand.
- Competitive Edge: Daily price changes involve changing the prices a little from one day to another and this makes it easier to respond to the actions of the competitor; one can easily time his/her change in prices.
Example: A web-based business utilizes generative AI in setting the price for the nearly fifty thousand items on its site depending on customers’ past browsing history,inventory data, and competitor prices. It assists to increase overall revenue to a steady twenty percent when making changes regarding the approaches to prices.
2. Product and Service Innovation
Description: Knowledge generative AI can assist in design creation and in modifying the current designs in accordance with the market requirements and the consumers and competitors’ analysis.
Benefits:
- Accelerated Innovation: The application of designs and concepts that are derived with the assistance of Artificial intelligence reduces the time one takes in coming up with products as well as get to the market.
- Better Market Fit: When the businesses incorporate the aspect of AI in information, then it would be simple for the aspect of production to deduce what is best suitable for customers.
Example: A case of generative AI at a consumer electronics firm meant that the firm employed an AI system to create new features for its products drawn from technology trends and consumers’ feedback. This leads to a successful launching of the product means a higher market share is achieved.
3. Customer Segmentation and Targeting
Description: The use of generative AI can entail an elite examination of customer information to develop customer architypes and segmentation which in turn facilitates the delivery of better customer targeting within a marketing campaign.
Benefits:
- Improved Marketing Efficiency: Since client segmentation is created through AI, the probabilities for the branded content to be shared are much higher, which means higher ROI.
- Enhanced Personalization: Companies can market better products to customers so as to gain a better shot at the consumer, hence improving the chances of sale.
Example: An example is the use of generative AI where a retail firm uses it to categorise customers into different personas. Thus, targeted marketing campaigns developed in relation to these personas increase conversion coefficients by 30%.
4. Supply Chain Optimization
Description: Because generative AI is stationary, it can mimic various supply chain situations to modify the inventory, predict client demand, and minimize expenses.
Benefits:
- Reduced Inventory Costs: Thus, a better way to predict the demand will help to avoid large stocks of products and, therefore, expensive storage.
- Improved Efficiency: Intelligent technologies assist in the managing of supply chain activities in an effective manner so as to minimize the lead time.
Example: An example of an application of generative AI is in the case of a manufacturing firm where the firm employs it to predict the market and consequently order its stock. The causes therefore mean a cut in inventory cost by fifteen percent and increase in the efficiency of the supply chain by ten percent.
5. The Integration of Promising Business Solution Areas:
FP has to be propelled to make use of predictive analytics.
Description: This is because with generative AI it is possible to come up with multiple management and planning simulations and models in the context of financial.
Benefits:
- Enhanced Financial Forecasting: Correct risk management helps to plan the probable outcomes thus the use of the AI to allow for dynamic output.
- Optimized Resource Allocation: AI boosts efficiency into the manner that the business implements its decision-making process through predictions.
Example: One industry that can benefit from generative AI is the financial services industry: an application case entails the use of generative AI to create various types of investment scenarios. This attribute translates to the right identification of prospects to be invested in thus helping the companies to enhance its investment portfolio and hence increase the companys performance.
6. Personalized Customer Experiences
Description: A primary advantage of generative AI is that it is able to generate specific content and recommends the specific appropriate product/service for every customer based on the input of the particular customer for enhancing the experience of the customer.
Benefits:
- Increased Customer Loyalty: Bespoke communication with the clients enhance customer satisfaction and causality.
- Enhanced Engagement: People do pay more attention to the intended content and respond more to the brand to some extend in case of personalized suggestions.
Example: An online streaming service uses generative AI in suggesting new programmes that the user can watch based on the user’s previous watch history and preferences. This way it gives a priority and attention to the individuals that there is more consumption of the content and high retention rate to the subscriptions again.
7. Units such as Fraud Prevention and Control and Risk Analysis
Description: A use of generative AI that can be of significance is to identify patterns and outliers from transactions data to fight fraud.
Benefits:
- Improved Security: Some of the benefits of using AI technologies includes, greater reduction of deceptive actions, and cost savings as a result of relevant information.
- Enhanced Risk Management: Thus, risks can be managed effectively through getting more data pattern and anomalies from the AI data of companies.
Example: The use of generative AI is illustrated in the banking organization to identify transactions for fraud of any kind that is a going on. Therefore, likely trough patterns in the dataset that might not be discerned with naked vision, financial fraud is massively minimized.
Generative AI and Its Applications in Profit Optimization: Challenges
However, it might be more appropriate to maintain that generative AI can indeed serve to support the process of rebuilding the foundations of profit optimization in practically any organization, which is located in almost all sectors and industries. Nonetheless, appropriate implementation of AI in the management of organizations is succeeded by issues and properties, which have to stimulate to unleash all the functions of application of AI. [4]This section presents main issues that are likely to incur during the process of generating AI for the purpose of enhancing profit.
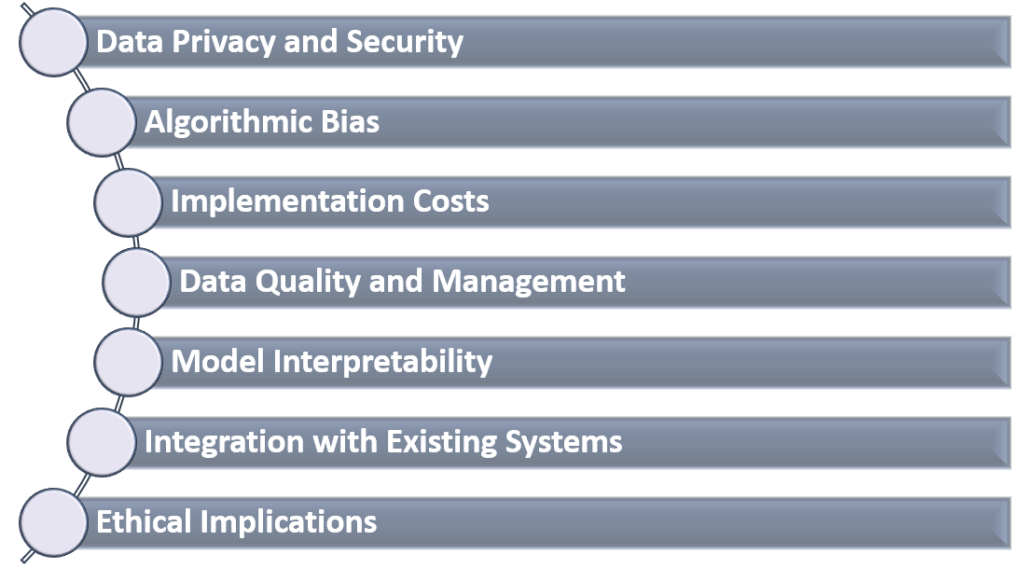
1. Data Privacy and Security
Challenge: Thus, in concordance with the general definition, generative AI systems are profoundly beneficial when worked with colossal datasets. I can confirm that this has resulted to some serious consequences regarding the data confidentiality and security specifically if the data that is being processed consists of sensitive information or details pertaining to the people concerned. Organization’s need to function through compliance regulations such as; GDPR, which is the General Data Protection Regulation and CCPA, which is the California Consumer Privacy Act.
Considerations:
- Data Anonymization: It will also be valuable to know methods that avoid the identification of an individual or methods that substitute the identification which will assist to protect the individual’s privacy.
- Compliance: Ensure that there is compliance with the law as well as ensuring that recommendations on the data protection laws to the particular AI applications are followed.
- Secure Data Handling: In an examination, ensure that the information is safe from any violation and or insecurity threats.
2. Algorithmic Bias
Challenge: Regarding most AI models that were generated, it should be noted that pathologies and prejudice harmonized in the data sets are reinforced and even highlighted. We have prejudices and that is incorrect because prejudices distort environmentally relevant decisions – it can result in unfair treatments of the subgroups of people targeted within the organizations.
Considerations:
- Diverse Data: Integrate the data from the above sources as well as data from different individuals into the training of the AI models in order to reduce on bias data.
- Bias Detection: Organize endeavors that could possibly facilitate the enhancement of a procedure that helps in the identification of bias in AI and the possible expulsion of biases where possible[5].
- Regular Audits: This will also be taken side by side with the check of the fairness of the used AI algorithms and any such check requires a certain action to be taken.
3. Implementation Costs
Challenge: It is also quite costly to include generative AI in normal business as the medium within day to day business operations. Costs are the cost adopted to purchase the technology, cost of new staff, and the cost of training the people[6].
Considerations:
- Cost-Benefit Analysis: When investing, one should perform a balance sheet and decide whether such investment will be worthy of it before investing on generative AI.
- Phased Implementation: Therefore, to reduce the costs during implementation and, in addition, to determine the impact of the implemented system in the organization, one should use the phased/ pilot implementation technique.
- Vendor Selection: Much depends on the better orientation in selection of suppliers rather universal, or partners in technologies, occupying themselves in providing proper references at rather perspective prices[7].
4. Data Quality and Management
Challenge: Most of the generative AI rely on data and so quality as well as management of the data directly determine the efficiency of the model. Absence of proper information or data which one can use to work on leaves a negative impact on creating perfect models; thus, the outcome cannot be perfect.
Considerations:
- Data Governance: Discuss how the information stewards’ framework can be used to ensure data reliability and quality for an organization.
- Regular Updates: Make sure that it is well updated and that the data administered to the models is accurate to assist the AI models.
- Data Cleansing: Such inaccuracies and inconsistencies have to be avoided, that is why it is necessary to transform the data and clean them on a regular basis.
5. Model Interpretability
Challenge: They also have the black box attribute, especially deep learning making it difficult for the stakeholders to understand how the decision making process is done. The effects diminish the level of openness and consequently the amount of the trust and acceptance.
Considerations:
- Explainable AI: Introduction practices and technologies for interpretation of results of AI models.
- Transparency: This is to indicate to an individual how the AI system reaches a certain decision and the factors that it considered to reach the said decision.
- Stakeholder Communication: Sensitize the stakeholders so that they can comprehend on the processes that are required in AI and the innovations to believe in the system.
6. Integration with Existing Systems
Challenge: It takes more factors into consideration when applying generative AI techniques as structures of a business, business processes may require a lot of work in the technical sense than what can be observed initially. There may be issues of cohesion and there can be disturbances in the current process flow.
Considerations:
- Compatibility Assessment: AI proposals should undergo analysis as to their applicability to the organization’s systems before implementation.
- Interoperability: Make they are compatible with the software and the platforms the business uses in their working operations.
- Change Management: Analyse and set the necessary effective changes of change management, which has to be implemented to guarantee the integrated change without creating the issues.
7. Ethical Implications
Challenge: The ethical issues that are not directly linked to generative AI are pertinent to the negative usage of text, video, image, etc. or information originating from this part of the AI system may seriously affect the life of a person. The following are the next ethical issues that assists in ensuring that the artificial intelligence is well implemented Hence we have to put into consideration the following aspects.
Considerations:
- Ethical Guidelines: Sadly, it is about high time that people developed new standards regarding the permitted practice of AI to turn to profit.
- Stakeholder Engagement: It is a process through which the related stakeholders efforts to make decisions in an attempt as they seek to resolve to the ethical ditches related to artificial intelligence[4].
- Governance Framework: To correspond to this call, it is required to lay down the prospect of ethical management in order to manage any concern related to the application of Artificial Intelligence and the deployment of mechanisms[8].
Conclusion
Thus, the integration of the generative AI in the profit improvement initiatives results in the considerable progression of the business entities’ administration and development. Hence, stimulating the abilities of the AI tool it is possible to develop completely new informational patterns that can be used by a firm for implementing variable pricing strategies, creating new good and services, designing the improved chains of supply, and raising the level of customer satisfaction. However some challenges are still found on the road towards the actual generative AI in its ideal form. The issue such as data security, bias in the algorithms, the expense that has to be incurred for implementing AI, ethical questions have to be considered for the proper and constructive use of the artificial intelligence technology. As companies and organisations are experimenting to know what generative AI has to deliver, it becomes quite relevant to response the question how innovation and ethic can go hand in hand which would help AI to be a base in the monetary area in the new world of business. Thus, if one considers the following years, those who will be able to stand or use the threats of artificial intelligence or opportunities in their favor will be those will be in the strongest positions in very competitive markets.
Reference
- L. Busch, “Governance in the age of global markets: challenges, limits, and consequences,” Agric. Hum. Values, vol. 31, no. 3, pp. 513–523, Sep. 2014, doi: 10.1007/s10460-014-9510-x.
- P. Pappachan, Sreerakuvandana, and M. Rahaman, “Conceptualising the Role of Intellectual Property and Ethical Behaviour in Artificial Intelligence,” in Handbook of Research on AI and ML for Intelligent Machines and Systems, IGI Global, 2024, pp. 1–26. doi: 10.4018/978-1-6684-9999-3.ch001.
- R. A. Abumalloh, M. Nilashi, K. B. Ooi, G. W. H. Tan, and H. K. Chan, “Impact of generative artificial intelligence models on the performance of citizen data scientists in retail firms,” Comput. Ind., vol. 161, p. 104128, Oct. 2024, doi: 10.1016/j.compind.2024.104128.
- H. Abdi, “Profit-based unit commitment problem: A review of models, methods, challenges, and future directions,” Renew. Sustain. Energy Rev., vol. 138, p. 110504, Mar. 2021, doi: 10.1016/j.rser.2020.110504.
- M. Rahaman, S. Chattopadhyay, A. Haque, S. N. Mandal, N. Anwar, and N. S. Adi, “Quantum Cryptography Enhances Business Communication Security,” vol. 01, no. 02, 2023.
- L. Cheng and T. Yu, “A new generation of AI: A review and perspective on machine learning technologies applied to smart energy and electric power systems,” Int. J. Energy Res., vol. 43, no. 6, pp. 1928–1973, 2019, doi: 10.1002/er.4333.
- M. Rahaman, F. Tabassum, V. Arya, and R. Bansal, “Secure and sustainable food processing supply chain framework based on Hyperledger Fabric technology,” Cyber Secur. Appl., vol. 2, p. 100045, Jan. 2024, doi: 10.1016/j.csa.2024.100045.
- E. Ferrara, “Fairness and Bias in Artificial Intelligence: A Brief Survey of Sources, Impacts, and Mitigation Strategies,” Sci, vol. 6, no. 1, Art. no. 1, Mar. 2024, doi: 10.3390/sci6010003.
- Gupta, G. P., Tripathi, R., Gupta, B. B., & Chui, K. T. (Eds.). (2023). Big data analytics in fog-enabled IoT networks: Towards a privacy and security perspective. CRC Press.
- Chaudhary, P., Gupta, B. B., & Singh, A. K. (2022). XSS Armor: Constructing XSS defensive framework for preserving big data privacy in internet-of-things (IoT) networks. Journal of Circuits, Systems and Computers, 31(13), 2250222.
- Sedik, A., Maleh, Y., El Banby, G. M., Khalaf, A. A., Abd El-Samie, F. E., Gupta, B. B., … & Abd El-Latif, A. A. (2022). AI-enabled digital forgery analysis and crucial interactions monitoring in smart communities. Technological Forecasting and Social Change, 177, 121555.
Cite As
Nayuni I.E.S. (2024) Generative AI and Its Applications in Profit Optimization, Insights2Techinfo, pp.1