By: Poojitha Nagishetti, Department of Computer Science and Engineering (Data Science), Student of Computer Science and Engineering (Data Science), Madanapalle Institute of Technology and Science, Angallu,517325, Andhra Pradesh.
Abstract –
Artificial Intelligence (AI) is transforming the healthcare sector, particularly in predicting diseases. By using advanced machine learning and deep learning algorithms, AI can analyze complex medical data to accurately estimate the emergence of various diseases. This article investigates the current applications of AI in disease prediction, obsessing on Alzheimer’s disease, cancer, and cardiovascular diseases. It analyzes the benefits of AI, such as enhanced early detection, Personalized treatment plans, increased efficiency, and cost savings, are discussed. However, significant barrier like data privacy, bias, regulatory compliance, and integration with existing healthcare systems remain challenges to widespread adoption. This study also highlights potential future directions for AI in healthcare, foreground the need for ongoing innovation, interdisciplinary collaboration, and robust regulatory frameworks to fully make use of the advantages of AI-driven disease prediction. By revolutionizing traditional diagnostic practices, AI has the potential to significantly improve patient outcomes and reshape the healthcare landscape.
Keywords – Artificial Intelligence, Disease Prediction, Machine Learning, Healthcare.
Introduction –
The origin of Artificial Intelligence (AI) has led to the remarkable transformations across various sectors, particularly in medical sector[1]. By using the capabilities of machine learning and deep learning AI has introduced the modern techniques and methods for predicting diseases, thereby transforming traditional diagnostic practices[2]. AI can analyze the large volume of complex medical data and detect patterns which are invisible to human clinicians and offers considerable promise for early disease detection and improved patient outcomes.
Particularly, in predicting diseases like Alzheimer’s, cancer, and cardiovascular diseases AI indicated a remarkable potential. These diseases, commonly diagnosed at more advanced stages, can very much benefit from early detection, which allows for timely medication and better management[3]. AI models will utilize data from medical imaging, genetic information, and electronic health records, can provide highly accurate predictions, that enables healthcare professionals to make more illuminated decisions.
However, the overview of AI in healthcare is not without challenges. Issues such as data privacy, bias, regulatory compliance, and the seamless integration with existing healthcare systems need to be addressed to fully realize AI’s potential. Moreover, the successful development and implementation of AI technologies require extensive interdisciplinary collaboration among data scientist, healthcare professionals, and policymakers[4].
This article explores the current use of AI in disease prediction, detailing its main applications, advantages, and the challenges. It also considers prospects, stressing the need for ongoing innovations and effective regulations to optimize AI’s benefits in healthcare and enhance patient care.
Methods –
This study conducted a large literature review to evaluate the impact of AI in disease prediction. It involved in collecting and analyzing research articles, reviews from credible academic journals, conference proceedings, online sources. The focus was on the applications of AI in predicting Alzheimer’s disease, cancer, and cardiovascular diseases. By using the performance metrices like accuracy, precision, recall and F1 score the performance of various AI models, such as convolutional neural networks (CNN), support vector machines (SVM), random forests etc., will be assessed[5]. By observing the Figure 1 it explains about the framework for disease prediction using AI analysis. The system integrates various data sources, including genetic sources and clinical data, into an AI analysis module to predict diseases. Challenges faced in the process and future directions for research and development are also outlined.
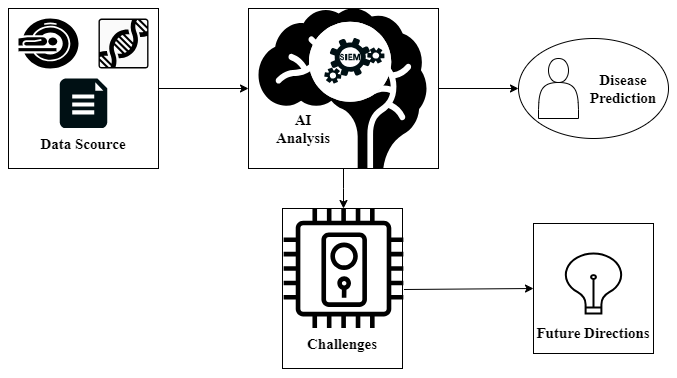
Applications of AI in Disease Prediction –
Artificial Intelligence (AI) has illustrated significant potential in predicting various diseases. As for Alzheimer’s disease, AI models will analyze MRI scans, genetic data, and related to assessments[6][6]. For cancer detection, data such as histopathological images, genetic markers, and patient records are used. Models such as Convolutional Neural Networks (CNN) and Recurrent Neural Networks (RNN) from deep learning are often achieve near-human accuracy in detecting and classifying cancers[7], greatly enhancing diagnostic precision[8]. For cardiovascular conditions, AI analyses electrocardiogram (ECG) signals, medical histories, and lifestyle information. Machine learning models like Random Forest and Gradient Boosting Machines have proven effective in predicting cardiovascular events[9], assisting in preventive measures, and reducing occurrence of heart attacks and strokes. Table 1 explains about the overview of AI applications and their effects on disease prediction.
Table 1: Overview of AI Applications and Their Effects on Disease Prediction
Category | Explanation | Key Features |
Data Inputs | Types of data used for AI analysis. | MRI Scans, DNA Sequences, Electronic Health Records (EHR) |
AI Processing | Methods and algorithms used to analyze the data. | Deep Learning Models, Neural Networks |
Disease Forecasting | Predictions made by AI regarding disease risk. | Alzheimer’s (Brain with Checkmark), Cancer (Awareness Ribbon), Cardiovascular Conditions (Heart) |
Advantages | Benefits derived from AI-based disease prediction. | Early Detection, Customized Treatments, Efficiency Gains, Cost Savings |
Challenges | Issues and obstacles in implementing AI solutions. | Privacy Concerns, Bias, Regulatory Issues, System Integration |
Prospects | Potential developments and areas for future growth. | Technological Innovations, Collaborative Research, Enhanced Regulations |
Benefits of AI in Disease Prediction –
- Early Diagnosis: AI helps in early detection of diseases, that led to better recurrence and more effective treatments.
- Personalized Medicine: By using each patient’s unique data AI algorithms can create individualized treatment planes.
- Efficiency: AI can process large datasets quickly, reducing the time needed for diagnosis and allowing for faster clinical decisions.
- Cost Effectiveness: Early diagnosis and personalized treatments will minimize the extensive treatments and hospital stays which led to significant cost savings.
Challenges and Limitations –
- Data Privacy: Maintaining the privacy and security of patient data is essential.
- Bias and Fairness: AI models need to be trained on diverse datasets to prevent biases that could affect accuracy.
- Regulatory Compliance: Navigating healthcare regulations and obtaining necessary approvals can be complex.
- Integration with Existing Systems: Incorporating AI solutions into existing healthcare infrastructure requires substantial effort and resources.
Future Directions –
The future of AI in disease Prediction looks promising, driven by advancements in technology and increased data availability. Improvements in AI algorithms and the integration of large-scale data will further enhance the accuracy and reliability of disease prediction models[10-13]. Collaboration among data scientists, healthcare professionals, and policymakers is crucial to addressing current challenges and maximizing AI’s potential in healthcare.
Conclusion –
AI has the capability to revolutionize healthcare by enabling accurate and early disease prediction. Despite existing challenges, the benefits of AI – such as early diagnosis, personalized treatment, efficiency, and cost savings – are substantial. Continuous research and collaboration are vital to fully leverage AI’s power in disease prediction, ultimately improving patient outcomes and transforming the healthcare landscape.
References –
- N. Noorbakhsh-Sabet, R. Zand, Y. Zhang, and V. Abedi, “Artificial Intelligence Transforms the Future of Health Care,” Am. J. Med., vol. 132, no. 7, pp. 795–801, Jul. 2019, doi: 10.1016/j.amjmed.2019.01.017.
- R. W. Pettit, R. Fullem, C. Cheng, and C. I. Amos, “Artificial intelligence, machine learning, and deep learning for clinical outcome prediction,” Emerg. Top. Life Sci., vol. 5, no. 6, pp. 729–745, Dec. 2021, doi: 10.1042/ETLS20210246.
- A. M. Widodo et al., “Port-to-Port Expedition Security Monitoring System Based on a Geographic Information System,” Int. J. Digit. Strategy Gov. Bus. Transform. IJDSGBT, vol. 13, no. 1, pp. 1–20, Jan. 2024, doi: 10.4018/IJDSGBT.335897.
- Y. K. Dwivedi et al., “Artificial Intelligence (AI): Multidisciplinary perspectives on emerging challenges, opportunities, and agenda for research, practice and policy,” Int. J. Inf. Manag., vol. 57, p. 101994, Apr. 2021, doi: 10.1016/j.ijinfomgt.2019.08.002.
- C. Sothe et al., “Comparative performance of convolutional neural network, weighted and conventional support vector machine and random forest for classifying tree species using hyperspectral and photogrammetric data,” GIScience Remote Sens., vol. 57, no. 3, pp. 369–394, Apr. 2020, doi: 10.1080/15481603.2020.1712102.
- N. Kang, K. Lee, S. Byun, J.-Y. Lee, D.-C. Choi, and B.-J. Lee, “Novel Artificial Intelligence-Based Technology to Diagnose Asthma Using Methacholine Challenge Tests,” Allergy Asthma Immunol. Res., vol. 16, no. 1, pp. 42–54, Dec. 2023, doi: 10.4168/aair.2024.16.1.42.
- D. Moitra and R. K. Mandal, “Prediction of Non-small Cell Lung Cancer Histology by a Deep Ensemble of Convolutional and Bidirectional Recurrent Neural Network,” J. Digit. Imaging, vol. 33, no. 4, pp. 895–902, Aug. 2020, doi: 10.1007/s10278-020-00337-x.
- M. Rahaman, C.-Y. Lin, P. Pappachan, B. B. Gupta, and C.-H. Hsu, “Privacy-Centric AI and IoT Solutions for Smart Rural Farm Monitoring and Control,” Sensors, vol. 24, no. 13, Art. no. 13, Jan. 2024, doi: 10.3390/s24134157.
- A. Ogunpola, F. Saeed, S. Basurra, A. M. Albarrak, and S. N. Qasem, “Machine Learning-Based Predictive Models for Detection of Cardiovascular Diseases,” Diagnostics, vol. 14, no. 2, Art. no. 2, Jan. 2024, doi: 10.3390/diagnostics14020144.
- M. Moslehpour, A. Firman, J. Sulistiawan, P.-K. Lin, and H. T. T. Nguyen, “Unraveling the Puzzle of Turnover Intention: Exploring the Impact of Home-Work Interface and Working Conditions on Affective Commitment and Job Satisfaction,” Behav. Sci., vol. 13, no. 9, Art. no. 9, Sep. 2023, doi: 10.3390/bs13090699.
- Sarin, S., et al. (2024). SEIR‐driven semantic integration framework: Internet of Things‐enhanced epidemiological surveillance in COVID‐19 outbreaks using recurrent neural networks. IET Cyber‐Physical Systems: Theory & Applications.
- Chhabra, A., et al. (2024). Sustainable and intelligent time-series models for epidemic disease forecasting and analysis. Sustainable Technology and Entrepreneurship, 3(2), 100064.
- Gupta, B. B., et. al.(2024). A Sustainable W-RLG Model for Attack Detection in Healthcare IoT Systems. Sustainability, 16(8), 3103.
Cite As
Nagishetti P. (2024) AI in Disease Prediction: Transforming Healthcare, Insights2Techinfo, pp.1