By: Praneetha Neelapareddigari, Department of Computer Science & Engineering, Madanapalle Institute of Technology and Science, Angallu (517325), Andhra Pradesh. praneetha867reddy@gmail.com
Abstract
Advanced detection and analysis approaches are required due to the substantial danger posed by the fast growth of malware to cybersecurity. Conventional signature-based techniques frequently fail to stop complex and dynamic malware. This study looks into potential applications of artificial intelligence (AI) to enhance malware detection and analysis. With machine learning and deep learning approaches, artificial intelligence (AI) can identify and classify malware faster and more precisely. The incapacity of conventional techniques to identify novel and polymorphic malware strains is the issue statement that is addressed. According to the report, AI-driven solutions offer a proactive strategy that may improve overall cybersecurity defences and adapt to new threats.
Keywords: Artificial Intelligence, Malware Detection, Cybersecurity, Cyberattacks
Introduction
The possibility of malware distribution is one of the important issues that appeared recently in the field of cybersecurity, based on which the need to develop new methods of protection[1]. Despite protecting against known threats, it seldom provides adequate protection from new strains of malware owing to the model on which the signature database operates. Therefore, the expansions of AI have similarly equally pervaded the identification and recounting of malware. Two general categories of AI Namely machine learning and deep learning strategies have the shelf capability of learning from giga byte data, analysing the trend and even re-orienting on real time threats. AI can also increase the rate of identifying malware and the efficiency of the process while giving further details on the nature of potentially suspicious software with the help of these frameworks. This revolutionary strategy is all aiming to increase the degree of their stability in the cyber defence and solidifying it with regards to the disclosed threats[2].
This is what challenges that modern malware create are and these are what AI and ML are trying to solve. Large data volumes can be analysed, and specific trends can be found, while abnormalities which may signify the existence of viruses can be found with the help of artificial intelligence. AI is more effective in cases of recognizing the existing and random malicious codes opposed to the conventional methods because AI evolves from previous invasions. Deep learning has a notable potential in tasks of features’ extraction from raw data and in increasing the accuracy of the malware detection.
The methodologies under AI can go a long way in making a lot of research on malware time bound and the cyber experts get better help in blocking threats or viruses on Internet. AI in malware analysis and detection is one of the tremendous strides in a bid to stop as well as protect infrastructures from cyber threats due to their dynamism.
1. Introduction of Malware
Malware is derived from the term malicious software and can be described as a program designed with an objective of causing harm to the devices, networks or computer systems intentionally or taking advantage of the present loopholes[3]. Malware’s main goal is to interfere with normal operations, gather and transfer data, or acquire access rights. Besides this, malware can work in the background and carry out actions invisibly; moreover, it can be classified under many different subcategories. It can also use various approach in the achievement of those goals[4].
1.1 Historical Evolution
Malware is constantly changing as did the tendencies and, at the same time, the complexity of the technologies in the recent years. The transmission of malware was relatively quite simple during early stages of computation and sometimes malware was created with support of physical vehicles such as floppy disks. This is because, with the increase in the members of the networks and the use of the internet, there was ‘‘malware’’, which was designed to act as a fraudster to the very systems. Although viruses and worms in general were created in the 1980 the advanced threats like Trojan horses and the worms based on emails appeared in the 1990. Spyware and ransomware increased its visibility on the 2000s due to such reasons as increased usage of the Internet to conduct online banking and the disclosure of their personal information. The latter, similarly, to other recent technologies, has shifted towards cloud configuration, smart devices, mobiles and Internet of Things[5].
1.2 Types of Malware
Viruses, which are also categories of the malicious programs, link themselves with authentic files or programs. When such files are opened, the virus that crops up among other files and beams out to other computers with the purpose of deleting or replacing information is run. Commonly, they are spread through transfer of files or viruses from the web, tainted messages and attached files from the mail services.
These ones are just simple reprehensible programs that take advantage of the known holes in the software or protocol to self-replicate as well as disseminate independently in the network. Sometimes, they influence the capability of the system directly by using up the resources such as the system’s resources or the network resources.
These viruses are named through the ball known as the Trojan horse; they appear as all genuine application that will help an individual download and install in the system. Once triggered, they can execute operations that the user does not need; for example, steal information or allow the attackers to infiltrate.
It encrypts the data that resides in the user’s PC or denies the user the right to access it, then the attacker can ask for a specific amount of money in exchange for the decryption of the data or granting of the right of access. To people and companies, it may seem more favourable than unfavourable for their fiscal picture and organization.
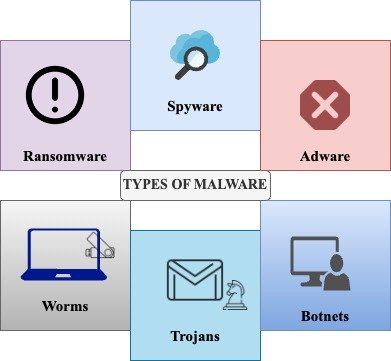
2. AI Techniques in Malware Detection
This method of identification, once the file or the network traffic has been detected, matches it with patterns or signatures. Although this technique is good in helping the antivirus to come across threats which it already has knowledge of, this technique is not useful when it comes to fresh strains of malware or the same strains with which slight alterations are made to such that they are not recognized by the antivirus software[6].
It supervises the implementation of the program to check for any-il legal activities such as access of files that is prohibited or any unusual activities in the network[7]. It also makes it possible to found new risks because that is a cornerstone of this method, which focuses on the subject’s behaviour rather than signals.
Last, anomaly detection with AI is the process of training machine learning models and, in turn, explaining their behaviour to find the pattern in the existing normality. Anomaly-based, which recognizes activities not in line with the patterns of the system’s operation, is effective in identifying new malware[8].
Algorithms that are incorporated in the classification of malware include decision tree, Support Vector Machines and neural network. These are applied to filter characteristics from files and network traffic and the apply them to malware[9]. Neural networks are connected nodes arranged in layers to self-train based on the given patterns and sort all the data and information while decision trees use rules of features to come to a decision and support vectors uses hyperplane to differentiate one class of data from the other. All these methods have their advantages and disadvantages; the usage of type of data may also affect the efficacy of each method or the task it is conducted for.
3. Machine Learning Models for Malware Detection
Classical models of artificial intelligence such as Random Forest and Naive Bayes are quite common in malware detection. While Classifiers like Naive Bayes analyse the probability of difference features to determine the nature of the malware, the latter develops many decision trees and averages the results to come up with a more accurate prognosis. These models commonly work well if the used data are structured and possess clear characteristics.
The sophisticated malware detection features give deep learning models such as recurrent neural networks (RNNs) and convolutional neural networks (CNNs). RNNs are more appropriate to timely analyse such things as system logs or network traffic, while CNNs are appropriate for analysing things that have hierarchical characteristics such as binary files or pictures[10]. These models improve the identification of advanced threats since they analyse complex pattern and interdependencies of the data.

4. Challenges
One of the biggest issues in Malware recognition is the occurrence of false positives and false negatives. Whenever good software is flagged by a security program as a threat, it results in false alarms and unnecessary disturbances[11]. These can be negative, in other words, negative results that occur when malware is genuinely unseen and unknown to the antivirus software. These issues can cause threats to the dependability and effectiveness of the detection systems.
5. Future Trends
This is partly true because of the dynamic development of AI technologies making it difficult to develop set ways of detecting malware. That is why new methods such as Generative Adversarial Networks (GANs) and transformer models, which belong to the deep learning process, are being actively studied. As these models become more precise and faster, to some extent these models could detect even complex behaviour patterns of malwares and signatures, which increases the possibility of detecting threats with previously unidentified or polymorphic entities.
Conclusion
In conclusion, a major development in cybersecurity is the incorporation of AI in malware analysis and detection. AI uses machine learning techniques and pattern recognition to improve the capacity to recognize and respond to hostile attacks more accurately and efficiently. Proactive defensive strategies and real-time threat analysis are made possible by these technologies, and they are essential for battling the constantly changing cyberthreat environment. AI will probably play an ever more important role in malware identification as it develops, providing advanced solutions to protect digital environments.
References
- Ö. A. Aslan and R. Samet, “A Comprehensive Review on Malware Detection Approaches,” IEEE Access, vol. 8, pp. 6249–6271, 2020, doi: 10.1109/ACCESS.2019.2963724.
- P. Pappachan, Sreerakuvandana, and M. Rahaman, “Conceptualising the Role of Intellectual Property and Ethical Behaviour in Artificial Intelligence,” in Handbook of Research on AI and ML for Intelligent Machines and Systems, IGI Global, 2024, pp. 1–26. doi: 10.4018/978-1-6684-9999-3.ch001.
- S. Abijah Roseline and S. Geetha, “A comprehensive survey of tools and techniques mitigating computer and mobile malware attacks,” Comput. Electr. Eng., vol. 92, p. 107143, Jun. 2021, doi: 10.1016/j.compeleceng.2021.107143.
- A.-A. Mustafa Majid, A. J. Alshaibi, E. Kostyuchenko, and A. Shelupanov, “A review of artificial intelligence based malware detection using deep learning,” Mater. Today Proc., vol. 80, pp. 2678–2683, Jan. 2023, doi: 10.1016/j.matpr.2021.07.012.
- M. Alenezi, H. Alabdulrazzaq, A. Alshaher, and M. Alkharang, “Evolution of Malware Threats and Techniques: A Review,” Int. J. Commun. Netw. Inf. Secur., vol. 12, p. 326, Dec. 2020, doi: 10.17762/ijcnis.v12i3.4723.
- M. J. Hossain Faruk et al., “Malware Detection and Prevention using Artificial Intelligence Techniques,” in 2021 IEEE International Conference on Big Data (Big Data), Dec. 2021, pp. 5369–5377. doi: 10.1109/BigData52589.2021.9671434.
- B. R. Maddireddy 1 and B. R. Maddireddy2, “Automating Malware Detection: A Study on the Efficacy of AI-Driven Solutions,” J. Environ. Sci. Technol., vol. 2, no. 2, Art. no. 2, Dec. 2023.
- L. Fritsch, A. Jaber, and A. Yazidi, “An Overview of Artificial Intelligence Used in Malware,” in Nordic Artificial Intelligence Research and Development, E. Zouganeli, A. Yazidi, G. Mello, and P. Lind, Eds., Cham: Springer International Publishing, 2022, pp. 41–51. doi: 10.1007/978-3-031-17030-0_4.
- B. D. Alfia, A. Asroni, S. Riyadi, and M. Rahaman, “Development of Desktop-Based Employee Payroll: A Case Study on PT. Bio Pilar Utama,” Emerg. Inf. Sci. Technol., vol. 4, no. 2, Art. no. 2, Dec. 2023, doi: 10.18196/eist.v4i2.20732.
- N. Pachhala, S. Jothilakshmi, and B. P. Battula, “A Comprehensive Survey on Identification of Malware Types and Malware Classification Using Machine Learning Techniques,” in 2021 2nd International Conference on Smart Electronics and Communication (ICOSEC), Oct. 2021, pp. 1207–1214. doi: 10.1109/ICOSEC51865.2021.9591763.
- F. A. Aboaoja, A. Zainal, F. A. Ghaleb, B. A. S. Al-rimy, T. A. E. Eisa, and A. A. H. Elnour, “Malware Detection Issues, Challenges, and Future Directions: A Survey,” Appl. Sci., vol. 12, no. 17, Art. no. 17, Jan. 2022, doi: 10.3390/app12178482.
- Mishra, P., Jain, T., Aggarwal, P., Paul, G., Gupta, B. B., Attar, R. W., & Gaurav, A. (2024). CloudIntellMal: An advanced cloud based intelligent malware detection framework to analyze android applications. Computers and Electrical Engineering, 119, 109483.
- Chui, K. T. (2023, November). A Lightweight Generative Adversarial Network for Imbalanced Malware Image Classification. In Proceedings of the 5th International Conference on Information Management & Machine Intelligence (pp. 1-4).
Cite As
Neelapareddigari P. (2024) AI in Malware Detection and Analysis, Insights2Tecginfo, pp.1