By: Dipesh Singla, Sudhakar Kr
The variety of information is expanding step by step. Breaking down the various information is getting troublesome because the information created is enormous, requiring exceptional techniques to be dissected adequately and proficiently. This expansion in the variety of information and its amount caused fast advancement in the information science industry. Information protection and security are a tremendous concern. The Data Science industry is confronting a colossal danger to its information security and information protection in the entirety of its chains, similar to information assortment, Data Production, information dissecting, and information sharing, and so forth. In this situation, Blockchain comes into a job named uncommonly for its decentralized foundation, security. Blockchain innovation can conquer many impediments to information science. With the help of information science, we can remove important information and product information items from it. However, Privacy, Security, Data Sharing, Uneven Distribution, and Uneven Demand for information are raising a ruckus in the advancement of Data Science [1]. Elements of Blockchain like straightforwardness, security, auditability, protection are correlative to information science.
Challenges in converging Blockchain in Data Science
Blockchain is still at its very initial stage, which has many benefits, as discussed in the next section, but it comes up with many challenges.
- Data Acquisition: – A foremost challenge in converging both is data acquisition. Since if an organization gets some data, it is still not sure that the organization can rely fully on that, and they might not surely extract some fruitful data from it. However, having a tremendous amount of data is not having any threshold, and companies can gain as much data as they can, but getting something out of it matters a lot [3].
- Competition: – These days, various businesses seek to collect as much information and data as possible in order to develop and stay ahead of their competitors in this IT World built on data. The variety and type of data in the Data Industry is also a problem when we wish to extract something from it.
- Scalability: – Data must be examined and processed at fast speeds and on a large scale in data science. However, there is a scalability issue with Blockchain in real-time trading. Because the Blockchain is larger in size, copying data to new nodes on the network takes a long time [28]. And because this problem cannot be handled simply by optimizing algorithms, blockchain providers are turning to solutions such as sharding (which may generate data security issues).
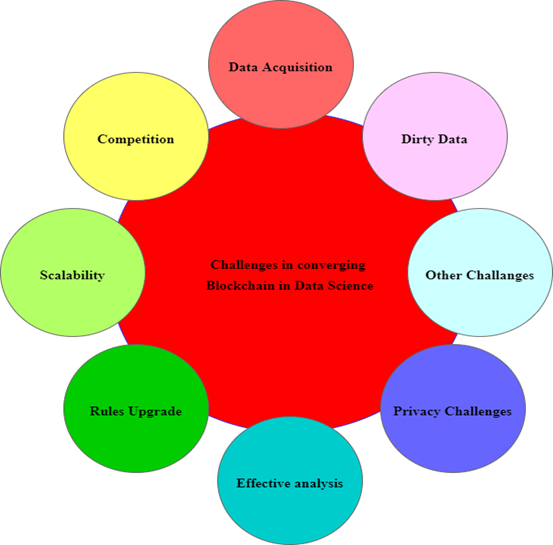
- Rules Upgrade: -Because the public Blockchain does not rely on a single authority, the decentralized nodes must agree on the transaction’s legitimacy. This problem is called a Consensus Upgrade Problem. This Problem came up since we want multiple participants in the network to agree. This is defined in the Consensus Algorithm. This is the role of the consensus algorithm to ensure that all nodes comply with the protocol rules and that all transactions are conducted reliably. Examples of consensus algorithms are POW and POS [4].
- Effective analysis: As the scale of data is getting higher and higher using Blockchain. The data effectiveness and beneficial analysis become important to scale up data and enhance overall use in multiple domains.
- Dirty Data: Controlling dirty data (or incorrect information) is one area where blockchain technology may significantly influence the data science industry. The inclusion of filthy data, such as duplicate or erroneous data, was regarded as the most difficult obstacle to data science in a 2017 poll of 16,000 data professionals.
- Privacy: – Through its decentralized nature, blockchain technology once again ensures data protection and privacy. The vast majority of data is stored on centralized servers, which cyber attackers regularly target; countless examples of hacks and security breaches illustrate the magnitude of the problem. On the other hand, Blockchain gives back control of data to the people who create it, making it impossible for thieves to access and change data on a large scale.
Benefits of using Blockchain for Data science
The main logic when Blockchain was introduced was its openness, transparency and decentralization, and security. By using Blockchain in startups and enterprises, it becomes convenient to trust and rely on Blockchain for security in the Internet World, moving a step further with the Digital Economy.
- Data Security and Data Privacy: Through their decentralized networks, blockchain-based models provide data security and privacy. Because data is housed on centralized servers, there is a risk of data leakage and loss. As a result, cyber attackers frequently target them. Blockchains decentralize data control, making it difficult for thieves to access and modify data on a large scale.
- Transparency: The convergence and interconnectivity of Blockchain and Data Science promote industrial transparency.
- Data Sharing: – To a large extent, Blockchain is resolving the problem of data sharing.
- Credibility: – The credibility of Blockchain is based on its security and avoidance of data theft. Unlike any other technology, it has caused massive businesses to trust it. Blockchain provides data sharing solutions by combining point-to-point transmission, a consensus mechanism, distributed storage, and encryption techniques [5].
References:
- Esposito, C., et al., Blockchain: A panacea for healthcare cloud-based data security and privacy? IEEE Cloud Computing, 2018. 5(1): p. 31-37.
- Mengelkamp, E., et al., A blockchain-based smart grid: towards sustainable local energy markets. Computer science research and Development, 2018. 33(1-2): p. 207-214.
- Lecuyer, M., et al., Enhancing selectivity in big data. IEEE Security & Privacy, 2018. 16(1): p. 34-42.
- Bach, L., B. Mihaljevic, and M. Zagar. Comparative analysis of blockchain consensus algorithms. in 2018 41st International Convention on Information and Communication Technology, Electronics and Microelectronics (MIPRO). 2018. IEEE.
- Wang, X., et al. A Kind of Decision Model Research Based on Big Data and Blockchain in eHealth. in International Conference on Web Information Systems and Applications. 2018. Springer.
Cite the article
Dipesh Signla, Sudhakar Kr (2021) Blockchain for Data Science, Insights2Techinfo, pp. 1
The amazing article is very informative and easy to understand.
Very well explained……
Very Informative and interesting article
Great content ! Easy to understand and informative . Great work !!!
Great content ! Easily understandable Great work !!!
Great work. I like it so much
Great. I like this content and help me a lot
Very good article!
Good research directions presented!👏
Thanks for providing such a great work in a flawless flowed manner.
Thank you for your appreciation
Thanks for such a great article all content is shown flawlessly and in easy to understandable manner
Thank you
https://insights2techinfo.com/blockchain-for-data-science/#comment-423
good Dipesh
amazing article all hardwork speaks itself
Thank you for your appreciation
Great article, very informative!
Thank you
Very knowledgeable
Thank you for your appreciation