By: Abhinav Singh1, Sunil Singh1 , Sherlyana2, Ahmad Nur Badri2
1 CSE Department, Chandigarh College of Engineering & Technology, Chandigarh, India.
2Department of computer science, Esa Unggul University, Indonesia
Abstract
This paper discusses data-based, Artificial Intelligence to address the current problem of congestion in urban areas. Tools such as real time monitoring, predictive analytics and dynamic signal control pertain to the discussed aspects of topics including real-time analysis, predictive modeling and intelligent traffic management. Different types of technologies are addressed, such as intelligent infrastructure and connected and autonomous vehicles (CAVs). Intelligent Traffic Systems, toll lanes and predictive policing are examples of their uses through successful implementation in countries like Singapore Los Angeles and London. Data-driven solutions for efficient and sustainable urban transportation have been pointed out as sources of the revolutionary potential in conclusion.
Keywords: – Intelligent Traffic Systems, AI-Powered Solutions, Intelligent Traffic Systems, Real-Time Analysis, Predictive Modeling, Dynamic Signal Control
1. Introduction: –
The agony has become a curse in the modern city; frustration, wasted time and value can no longer be ignored. With the increasing population in urban areas and vehicle ownership, conventional infrastructure-based solutions are deemed less adequate. But the advent of AI [1] driven approaches based on data has brought some hope to fight this perennial challenge.
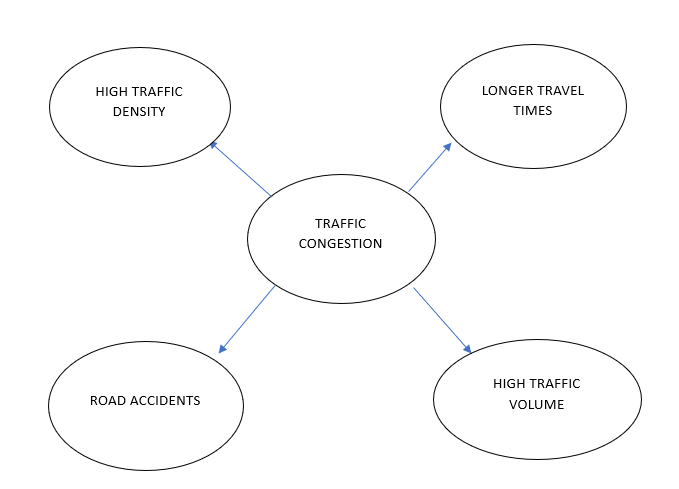
It explains that too many cars on the road means everyone moves slower. Like squeezing water through a tiny pipe, it takes longer to get anywhere. This slows everyone down, and wastes precious time, which also cause accidents. The solutions that have been created to fight against traffic congestion is discussed in this article. We discuss how the use of real-time data analysis, predictive modeling and intelligent traffic management systems are contributing to a seamless flow for cars.
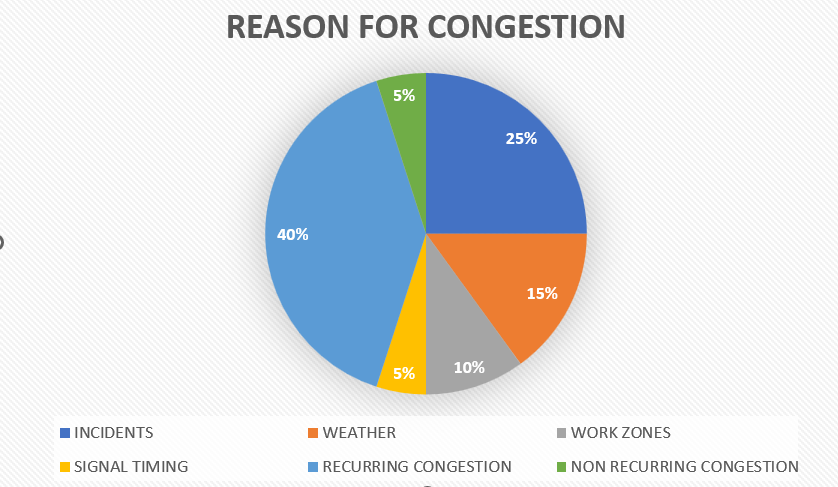
This figure explains that rush hour & busy spots contribute most in the charts 40%. Unforeseen events like accidents & surprises cause 25% of jams. Road work adds in 15%. Weather adds 10%, while poorly timed lights & incidents each contribute 5% and non recurring also contribute 5% congestion on roads
2. The Power of Data: –
The way to achieve data-driven solutions is through the control of all big amounts from vehicles, sensors and transportation networks. This entails GPS data, CCT cameras footage, mobile app utilization as well as weather information. Through real-time analysis of this data, we are able to obtain important information about traffic dynamics [2] such as the identification of bottlenecks and forecasting congestion hotspots.
Table 1: Potential Solutions of Traffic Congestion
Data Source | Insights Gained | Potential Solutions |
GPS Data: Location, speed, acceleration, route choice | Understand travel patterns, identify popular routes, detect real-time congestion | Dynamic traffic signal control, congestion pricing, personalized navigation |
Traffic Camera Footage: Traffic flow, lane utilization, incidents | Monitor conditions, detect accidents and lane blockages, analyze volume variations | Incident response, dynamic lane management, adaptive speed limits |
Mobile App Usage: User reports, route information, travel times | Analyze travel behavior, predict demand for specific routes, gauge traveler satisfaction | Public transportation optimization, ridesharing incentives, demand-responsive transit |
Weather Information: Rainfall, snowfall, visibility | Predict weather-related congestion, adjust traffic flow based on conditions | Early warning systems, proactive detours, weather-adaptive signal timing |
This table shows the potential of using various data sources to gain valuable insights into traffic patterns and develop effective solutions to improve traffic flow. By getting data from GPS, traffic cameras, mobile apps, and weather information, cities and transportation authorities can implement strategies like dynamic traffic signal control, congestion pricing, and improved public transportation options, ultimately leading to a smoother and more efficient travel experience for everyone.
3. Toolbox for Smarter Traffic Management: –
The data-driven toolbox for tackling traffic congestion in smart cities[3-4] includes a variety of powerful tools:
3.1 Real-time Traffic Monitoring [5]:- Sensor networks and intelligent vehicles enable a real-time view of traffic flow such that authorities can adaptively control the signal signals in synchronization with vehicle routing for driving diversion around congested sections.
3.2 Predictive Analytics: – The Machine Learning [6-8] algorithms can use historical information and current conditions to forecast congestion patterns, providing pre-emptive countermeasures. Think about pre-routing school buses or delivery trucks before they interfere with traffic leading up to rush hours.
3.3 Dynamic Traffic Signal Control: – In place of traditional fixed-timing traffic signal[9] , intelligent systems[10] that accommodate real current conditions are being substituted. This would go a long way to reduce the waiting time and improve on traffic management across junction points.
3.4 Multimodal Integration: – Data-driven solutions are not confined to cars. Public transportation systems can use real-time data to perfect their schedules, create more synergies between distinct travel means, and motivate travelers towards a greener option.
4. Beyond the Horizon: –
Data-driven solutions are just in the process of evolving. The future holds even more exciting possibilities, with technologies like:
4.1 Connected and Autonomous Vehicles (CAVs) [11]:- CAVs can connect with other vehicles and infrastructure, allowing platooning (vehicles driving in close order) to occur as well as synchronized maneuvers which ensure smoother traffic flow and the potential for increased road capacity.
4.2 Intelligent Infrastructure: – Roads that utilize sensors and smart meters have the ability to adapt speed limits, lane markers as well as charging stations driven by live traffic data in real-time.
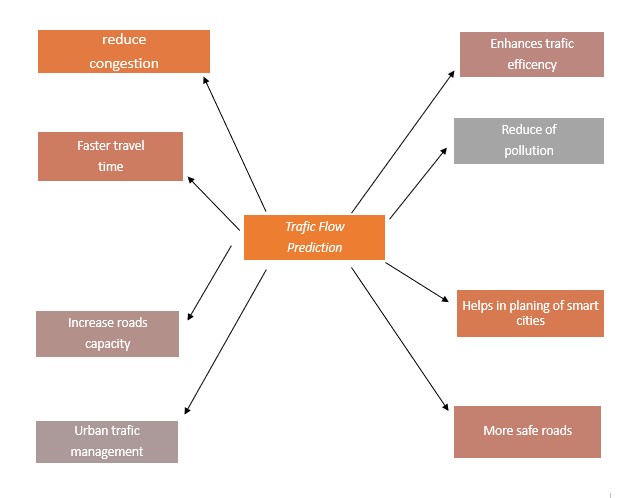
Fig 3: Traffic Flow Prediction
This figure shows the benefits of traffic flow prediction, it helps in reduce congestion, faster travel time , less chances of accident , also helps in reducing pollution , traffic management is improved and helps in planning of smart cities .
5. Real world examples of cities successfully implementing data driven solution: –
5.1 Singapore: –
- Intelligent Traffic System (ITS):-Hence, ITS in Singapore encompasses integration of data from sensors cameras and GPS sources used for traffic monitoring to vary signals dynamically along with provision of real time information to drivers.
- Green Link Determining (GLIDE) System[12]:– This system optimizes signal timings along major corridors to the effect of reducing travel times by as much as 20%.
- Electronic Road Pricing (ERP) :- A peak-hour road pricing system to cut traffic by making drivers use public means of transport, or travel at off peak time.
5.2 Los Angeles: –
- Automated Traffic Surveillance and Control (ATSAC)[13]:- There is a network of more than 4,500 sensors and cameras which provide real-time information on traffic situations facilitating fast response to incidents as well as proactive management.
- Express Lanes:- Toll lanes on freeways that vary their price depending of traffic flows, promoting carpooling and minimizing the congestion in common carrying paths.
- Predictive Policing :- AI can predict crime hotspots that will increase traffic congestion due to accidents[14] and incidents, allowing LAPD officers to be proactively deployed.
5.3 London: –
- SCOOT (Split Cycle Offset Optimization Technique)[15]:- A traffic management system that works dynamically and adjusts the signal timings according to real-time data, improving delays by 12%.
- iBus[16]:- A real-time bus tracking system that predicts arrival times accurately to the commuters and persuades them using public transportations instead of using vehicles.
- Congestion Charge Zone [17] :- A daily charge on drivers entering the central London peak hours, cutting down its congestion while improving air quality there.
6. Conclusion: –
Data-driven technologies are changing traffic congestion management and creating a more intelligent and effective method of urban transportation. The integration of real-time data, prediction algorithm [18-19] and AI powered systems in cities are already showing significant improvements on matters like traffic flow, has shorter commute time as well improved the livability life. Such digital data are changing the future of transportation including predictive traffic congestions, dynamic management signals and automotive linked vehicles with intelligent infrastructure. With these innovations, we can move towards cities that are not only less stressful and people-oriented, but also environmentally sustainable [20] and built for the future.
References: –
- Mandal, V., Mussah, A. R., Jin, P., & Adu-Gyamfi, Y. (2020). Artificial intelligence-enabled traffic monitoring system. Sustainability, 12(21), 9177.
- [2] Avila, A. M., & Mezić, I. (2020). Data-driven analysis and forecasting of highway traffic dynamics. Nature communications, 11(1), 2090.
- [3] Singh, R., Singh, S. K., Kumar, S., & Gill, S. S. (2022). SDN-Aided Edge Computing-Enabled AI for IoT and Smart Cities. SDN-Supported Edge-Cloud Interplay for Next Generation Internet of Things, 41-70.
- [4] Chopra, M., Kumar, S., Madan, U., & Sharma, S. (2021, December). Influence and establishment of smart transport in smart cities. In International Conference on Smart Systems and Advanced Computing (Syscom-2021).
- [5] Ferrier, N. J., Rowe, S., & Blake, A. (1994, December). Real-time traffic monitoring. In WACV (pp. 81-88).
- [6] Chopra, M., Singh, S. K., Aggarwal, K., & Gupta, A. (2022). Predicting catastrophic events using machine learning models for natural language processing. In Data mining approaches for big data and sentiment analysis in social media (pp. 223-243). IGI Global.
- [7] Mengi, G., Singh, S. K., Kumar, S., Mahto, D., & Sharma, A. (2021, September). Automated Machine Learning (AutoML): The Future of Computational Intelligence. In International Conference on Cyber Security, Privacy and Networking (pp. 309-317). Cham: Springer International Publishing.
- [8] Setia, H., Chhabra, A., Singh, S. K., Kumar, S., Sharma, S., Arya, V., … & Wu, J. (2024). Securing the Road Ahead: Machine Learning-Driven DDoS Attack Detection in VANET Cloud Environments. Cyber Security and Applications, 100037.
- [9] Singh, I., Singh, S. K., Kumar, S., & Aggarwal, K. (2022, July). Dropout-VGG based convolutional neural network for traffic sign categorization. In Congress on Intelligent Systems: Proceedings of CIS 2021, Volume 1 (pp. 247-261). Singapore: Springer Nature Singapore.
- [10] Kumar, S., Singh, S. K., Aggarwal, N., Gupta, B. B., Alhalabi, W., & Band, S. S. (2022). An efficient hardware supported and parallelization architecture for intelligent systems to overcome speculative overheads. International Journal of Intelligent Systems, 37(12), 11764-11790.
- [11] Ye, L., & Yamamoto, T. (2018). Modeling connected and autonomous vehicles in heterogeneous traffic flow. Physica A: Statistical Mechanics and its Applications, 490, 269-277.
- [12] Gokulan, B. P., & Srinivasan, D. (2010). Distributed geometric fuzzy multiagent urban traffic signal control. IEEE Transactions on Intelligent Transportation Systems, 11(3), 714-727.
- [13] Rowe, S. E. (1992). Automated Traffic Surveillance and Control (ATSAC) and Smart Corridor Projects in Los Angeles. In International Congress on Transportation Electronics (1992: Detroit Mich.). Vehicle electronics meeting society’s needs.
- [14] Chui, K. T., Kochhar, T. S., Chhabra, A., Singh, S. K., Singh, D., Peraković, D., … & Arya, V. (2022). Traffic accident prevention in low visibility conditions using vanets cloud environment. International Journal of Cloud Applications and Computing (IJCAC), 12(1), 1-21
- [15] Day, I., Ag, S., & Whitelock, R. (1998, July). SCOOT-split, cycle & offset optimization technique. In TRB Mid-Year Meeting and Adaptive Traffic Signal Control Workshop (Vol. 7).
- [16] Wong, A., & Hounsell, N. (2010). Using the iBus system to provide improved public transport information and applications for London.
- [17] Leape, J. (2006). The London congestion charge. Journal of economic perspectives, 20(4), 157-176.
- [18] Peñalvo, F. J. G., Maan, T., Singh, S. K., Kumar, S., Arya, V., Chui, K. T., & Singh, G. P. (2022). Sustainable Stock Market Prediction Framework Using Machine Learning Models. International Journal of Software Science and Computational Intelligence (IJSSCI), 14(1), 1-15.
- [19] Singh, I., Singh, S. K., Singh, R., & Kumar, S. (2022, May). Efficient loop unrolling factor prediction algorithm using machine learning models. In 2022 3rd International Conference for Emerging Technology (INCET) (pp. 1-8). IEEE.
- [20] SINGH, S., KARTIK, J. A. S. G., & KUMAR, S. The Role of Site Reliability Engineering in Sustainable Development. space, 2(10),
Cite As:
Singh A., Singh S. , Sherlyana , Badri A. N. (2024) Data Driven Solutions for Traffic Congestion, Insights2Techinfo, pp.1