By: Himanshu Tiwari, International Center for AI and Cyber Security Research and Innovations (CCRI), Asia University, Taiwan, nomails1337@gmail.com
In the age of statistics, healthcare has not been left untouched with the aid of the transformative power of advanced generation. Federated getting to know, a singular method to machine studying, has emerged as a promising approach to deal with a number of the most pressing challenges within the healthcare enterprise. This revolutionary technique is about to change the manner healthcare specialists, researchers, and institutions cope with sensitive patient facts, improve diagnosis accuracy, and broaden extra customized treatment plans. In this article, we are able to delve into the world of federated gaining knowledge of in healthcare, exploring its ideas, packages, and the ability it holds for the future[1][2].
Understanding Federated Learning
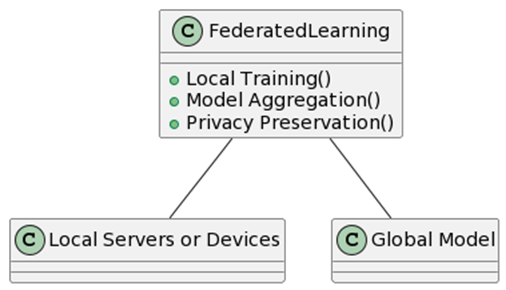
Federated studying is a machine studying technique designed to teach a global model across more than one decentralized records assets whilst keeping the data localized and steady. In the context of healthcare, because of this patient information remains inside the premises of hospitals, clinics, or person gadgets, and the version is educated collaboratively with out ever centralizing sensitive records[1].
The middle principles of federated getting to know include privacy renovation, records safety, and decentralized model education. Here’s how it works:
1. Local Training: Data stays on the local servers or gadgets. Hospitals, clinics, or individuals retain manage over their statistics.
2. Model Aggregation: Instead of centralizing statistics, handiest version updates are shared, permitting the global model to be improved without sharing the statistics.
3. Privacy Preservation: Federated gaining knowledge of employs strategies like encryption and differential privacy to make sure affected person confidentiality.
Applications in Healthcare

Federated studying has the potential to revolutionize various elements of healthcare, together with[2][3]:
1. Diagnosis and Prediction: By education models on various healthcare datasets, federated studying can improve the accuracy of ailment prognosis and affected person outcome prediction. This is particularly beneficial in areas which includes radiology, pathology, and genomics.
2. Drug Discovery: Pharmaceutical businesses can benefit from federated gaining knowledge of by way of collaboratively studying clinical trial statistics with out sharing proprietary statistics. This can boost up drug discovery and improvement.
3. Personalized Medicine: Federated gaining knowledge of enables the advent of pretty personalised treatment plans based totally on a patient’s specific health information, such as genetics, lifestyle, and medical history.
4. Epidemiology and Public Health: Healthcare groups and researchers can use federated gaining knowledge of to music and are expecting sickness outbreaks, for this reason enhancing public fitness responses.
5. Clinical Decision Support: Healthcare companies can combine federated mastering models into their Electronic Health Records (EHR) systems to provide real-time clinical decision guide, making sure that docs have get admission to to the most up to date scientific expertise.
Benefits of Federated Learning in Healthcare
1. Data Privacy: Patient statistics remains secure and private, lowering the danger of information breaches and making sure compliance with statistics protection policies including HIPAA and GDPR.
2. Data Diversity: Federated getting to know encourages collaboration between one of a kind healthcare establishments, ensuing in more numerous datasets, which could result in greater sturdy and accurate fashions.
3. Reduced Data Transfer: Since information stays localized, there is no want to transfer big datasets, lowering bandwidth and infrastructure prices.
4. Efficiency: Federated getting to know can drastically speed up the version schooling system, enabling faster scientific discoveries and treatment development.
5. Improved Healthcare Outcomes: With more correct diagnostics, customized remedies, and real-time medical selection guide, federated learning has the capacity to keep lives and enhance patient results.
Challenges and Considerations
While federated mastering holds terrific promise in healthcare, there are demanding situations to cope with, which includes:
1. Standardization: Developing enterprise-huge standards for federated learning is important to make sure interoperability and consistency.
2. Security: Ensuring the security of the federated learning infrastructure is paramount to save you attacks on the model updates and the statistics.
3. Data Imbalance: Striking a stability between records localization and ensuring various illustration may be difficult, as a few institutions may have more facts than others.
Conclusion
Federated learning in healthcare represents a ground-breaking approach that reconciles the need for privateness and statistics protection with the demand for advanced hospital therapy and studies. As the healthcare industry keeps to conform within the digital age, federated gaining knowledge of guarantees to play a pivotal position in riding improvements in diagnostics, drug discovery, and personalized remedy. Its adoption will depend upon overcoming technical challenges, growing sturdy standards, and fostering collaborative efforts among healthcare institutions, however the capability advantages for patients and the enterprise are undeniably significant.
Reference
- Zhang C, Xie Y, Bai H, Yu B, Li W, Gao Y. A survey on federated learning. Knowledge-Based Systems. 2021 Mar 15;216:106775.
- Mammen PM. Federated learning: Opportunities and challenges. arXiv preprint arXiv:2101.05428. 2021 Jan 14.
- Li T, Sahu AK, Talwalkar A, Smith V. Federated learning: Challenges, methods, and future directions. IEEE signal processing magazine. 2020 May 1;37(3):50-60.
- Cvitić, I., Perakovic, D., Gupta, B. B., & Choo, K. K. R. (2021). Boosting-based DDoS detection in internet of things systems. IEEE Internet of Things Journal, 9(3), 2109-2123. https://ieeexplore.ieee.org/abstract/document/9461235/
- Alieyan, K., Almomani, A., Anbar, M., Alauthman, M., Abdullah, R., & Gupta, B. B. (2021). DNS rule-based schema to botnet detection. Enterprise Information Systems, 15(4), 545-564.
- Deveci, M., Pamucar, D., Gokasar, I., Köppen, M., & Gupta, B. B. (2022). Personal mobility in metaverse with autonomous vehicles using Q-rung orthopair fuzzy sets based OPA-RAFSI model. IEEE Transactions on Intelligent Transportation Systems.
- Chopra, M., Singh, S. K., Gupta, A., Aggarwal, K., Gupta, B. B., & Colace, F. (2022). Analysis & prognosis of sustainable development goals using big data-based approach during COVID-19 pandemic. Sustainable Technology and Entrepreneurship, 1(2), 100012.
Cite As:
Tiwari H. (2023) Revolutionizing Healthcare: Federated Learning, Insights2Techinfo, pp.1