By: Arya Brijith, International Center for AI and Cyber Security Research and Innovations (CCRI), Asia University, Taiwan,sia University, Taiwan, arya.brijithk@gmail.com
Introduction
Time-series data analysis and prediction are well-known to be strengths of the Seasonal Autoregressive Integrated Moving Average, or SARIMA, which is a potent forecasting model. It is a shining example of forecasting accuracy in the field of statistical modeling and time-series analysis, especially when it comes to datasets with intricate and seasonal patterns. Let us explore its components and applications.
Components
- Sеasonal (S): This element recognizes the patterns that recur inside the data at fixed intervals.
- Autorеgrеssivе (AR): The relationship between an observation and a specific number of lagging observations is reflected.
- Intеgratеd (I): consists of separating the time series to achieve stationarity and reduce trends or non-stationarity in the data.
- Moving Avеragе (MA): Takes into account the weighted average of error terms when forecasting.
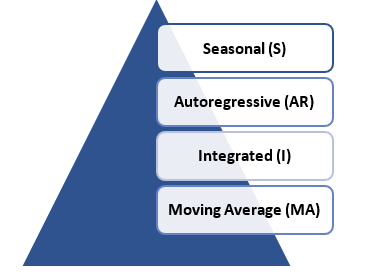
Application
- Economic Forecasting: Seasonal trends and historical patterns have a significant impact on stock prices, GDP, sales, and other economic indicators. SARIMA is particularly useful in this regard.
- Demand Forecasting: SARIMA analyzes past sales data to help the retail and supply chain industries forecast demand, which improves inventory management and supply chain operations.
- Climatе and Mеtеorology: Temperature changes, seasonal trends, and weather patterns are all predicted using SARIMA models, which are used in meteorological and climate studies to help with long-term climate forecasting.
Conclusion
SARIMA is a powerful tool for forecasting. This article sheds light on the potential future paths of many phenomena influenced by time-based patterns and cyclical behavior.
SARIMA’s journey persists as industries shift and datasets get increasingly intricate. It is propelled by ongoing advancements and breakthroughs in predictive analytics, which underscore the crucial role that it plays in decision-making across a range of sectors and promise improved forecasting accuracy and efficiency.
References
- Luo, C. S., Zhou, L. Y., & Wei, Q. F. (2013). Application of SARIMA model in cucumber price forecast. Applied Mechanics and Materials, 373, 1686-1690.
- Otu, O. A., Osuji, G. A., Opara, J., Mbachu, H. I., & Iheagwara, A. I. (2014). Application of Sarima models in modelling and forecasting Nigeria’s inflation rates. American Journal of Applied Mathematics and Statistics, 2(1), 16-28.
- Rajput, R. K. S., Goyal, D., Pant, A., Sharma, G., Arya, V., & Rafsanjani, M. K. (2022). Cloud data centre energy utilization estimation: Simulation and modelling with idr. International Journal of Cloud Applications and Computing (IJCAC), 12(1), 1-16.
- Sharma, A., Singh, S. K., Badwal, E., Kumar, S., Gupta, B. B., Arya, V., … & Santaniello, D. (2023, January). Fuzzy Based Clustering of Consumers’ Big Data in Industrial Applications. In 2023 IEEE International Conference on Consumer Electronics (ICCE) (pp. 01-03). IEEE.
- Chui, K. T., Gupta, B. B., Jhaveri, R. H., Chi, H. R., Arya, V., Almomani, A., & Nauman, A. (2023). Multiround transfer learning and modified generative adversarial network for lung cancer detection. International Journal of Intelligent Systems, 2023, 1-14.
- Chui, K. T., Gupta, B. B., Torres-Ruiz, M., Arya, V., Alhalabi, W., & Zamzami, I. F. (2023). A Convolutional Neural Network-Based Feature Extraction and Weighted Twin Support Vector Machine Algorithm for Context-Aware Human Activity Recognition. Electronics, 12(8), 1915.
Cite As
Brijith A. (2023) SARIMA (Seasonal Autoregressive Integrated Moving Average): Its Components and Applications, Insights2Techinfo, pp.1