By: K. Sai Spoorthi, Department of computer science and engineering, Student of computer science and engineering, Madanapalle Institute of Technology and Science, 517325, Angallu, Andhra Pradesh.
Abstract
One of the most discussed ways of AI application is the use of generative AI, which concerns the improvement of efficiency and creativity of business processes and production in many spheres. However, these benefits contradict at a great deal financially hence calling for several considerations. In this paper, an effort is made to present the economic patterns of apply generative AI solutions, which we specify by evaluating the initial capital outlay to implement this solution, expense required to maintain or to keep it running, and additional costs that are essential for training. Thus, generative AI is characterized by potential increases in efficiency and productiveness, as well as by significant requirements for investments, platforms, and professionals that are problematic for organizations. This is in fact the most critical learning from the study because without the right strategies in place ROI may probably be high, but the costs far outweigh the benefits in the long run resulting in unsustainable operation and business competitiveness.
Key words: Generative AI, implementation costs, Resource allocation, sustainable growth, operational challenges, Economic implications, Maintenance
Introduction
Further with the advancement in various fields a decentralised use of generative artificial intelligence (AI) has been seen as revolutionary in this generation. Now, various companies and different organizations have interest in the chance to utilize generative artificial intelligence systems that naturally enhances the productivity and finds new ideas as well as introduces efficiency.[1] However, the enthusiasm surrounding these technologies often overshadows an essential consideration: some of the economic implications that will accrue to them. But unfortunately the reality of the matter is that generative AI is not cheap; it requires more investments in terms of capital and infrastructure, manpower and time for repeated conducting of various reviews and so on which is likely to pinch even the most heinous financially organizations. The purpose of this essay is to discuss how one should think about generative AI solutions in terms of the first cost that must be estimated, and in terms of all subsequent costs connected with the using of the technologies that have been introduced and how all of these costs can produce significant value. In this way, it tries to provide a detailed view of the economic conditions of generative AI’s usage.
Generative AI and Its Growing Importance
Newer and epoch-making innovations have come into practice in the recent years in the generative AI category which alters the traditionally practiced sectors in a big way. This method in which computer is capable of producing material; text, graphics as well as music has important application ever expanding sectors such as marketing, entertainment as well as education. The fact that generative AI is not only able to augment the creative process with the capacity to optimise efficiency in doing so places it in a position of growing indispensability in a world that can only grow increasingly competitive. In view of the fact that organizations seek to harness these capabilities, there has been growing application of generative AI solutions as indicators of model-based business and transforming consumer engagements. However, its work is not without some problems – the main one being its need for much financial investment, expertise and ethical dilemmas related to its applicability. Therefore, while generative AI is progressively integrating into industries, is now necessary to assess its cost spectrum in order to harness as many possible advantages while decreasing the possible drawbacks.
Financial Implications of Generative AI Implementation
Generative AI technologies belong to this category, and the financial effects of using such technologies require thorough analysis because the cost may sharply differ from industry to industry. Implementation costs in some cases are not only the cost price of technology but other costs like how much technology training costs, how much it will cost to implement the selected technology or how much it will cost to maintain the technological advancement made over the current year. Many costs could incur in powerful equipment, software, and professionals who can solve the issues with generative AI for businesses. Also such operational costs as cost for utilizing a cloud service, energy consumption and frequently recurring cost for managing data and compliance must be taken into account. Still, these expenditures may be made up by an increase in productivity and, most notably, by the revenues generative AI can generate. And when such solutions get used in companies, they are guaranteed to stand to gain from the benefits that come with it including the cutting of costs which include the automation of repetitive tasks and improvements in the systems used in making decisions that are used in the production of a firmer and stronger bottom line. That is why the choice of the works and materials to order as well as the question of how they are going to be paid are vital for successful integration between the two firms: Given this, as in the fig.1, it is always a matter of how much cash the firm can commit initially to reap a reasonably high return, or high profit in the future.
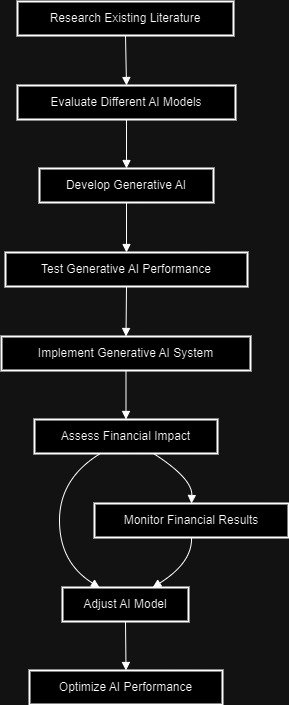
Operational Challenges and Ongoing Cost
Applying generative AI solutions in engineering and design bring a number of operational measures that affect the continuing costs greatly. The first and perhaps the most apparent is the fact that the use of generative algorithms and tools requires a lot of expertise and knowledge to set up, which may be a costly affair since it may require training of the current employees. This is especially the case in the area of application of built environment especially generative design (GD) where a symbiotic relationship has to be struck between programming and architecture. . In addition, the current and future improvement of material properties as well as the overall optimisation of production parameters with the help of generative AI increases the operational workload as it has to be constantly adapted and adjusted. When organizations implement these technologies the experience challenges with regard to system integration, data handling, and support. Consequently, the concepts of increased innovation and efficient processes can be very appealing; however, to ensure generative AI solutions will continue to deliver improvements, one must come to terms with costs: the initial and recurring ones required to run them.
Maintenance, Training, and Resource Allocation
It is mandatory and significant to maintain the generative AI systems for their continual functionality and to meet the organizational dynamic requirements. Usually such systems are updated often and checked for their performance in order to prevent futures that may be costly to the business performance.[2] Also, specific training for staff is necessary, not for the improvement of users’ competence only but for creating an innovation climate within which workers are challenged to apply AI solutions. Application-based sessions are much more effective in terms of user engagement that can provide a vast improvement in the results. In addition, there is a necessity to prioritize the distribution of the financial and human capital on such initiatives. This also involves setting of funds for future training and development plans as well as setting of maintenance factors that may involve scaling and addition as the technology becomes more stable.[3] Lacking such well-coordinated investments, organisations could lose an opportunity to unleash the full potential of their generative AI solutions
Costs and Future Considerations for Generative AI Solutions
The execution of the generative AI solutions is a process that involves multiple forms of costs: direct and indirect, and organizations need to choose wisely. Preliminary costs usually include the costs of licenses, and construction or purchase of the data structure which might be expensively burdensome for an organization. In addition, fixed costs like maintenance and hardware costs, cost of storage of data, energy costs, etc have to be incorporated into revenue and expenditure considerations in the long run. However, such investments up front are frequently warranted in advance by the opportunities for significant gains in productivity and the release of innovative potential. With respect to the future environment, one should carefully monitor which technological solutions in the field of generative AI, as well as shifts in the market and organizational environment, may affect the cost of implementing AI solutions. [4]Besides, legal requirements and professionalism mandates may require more investment to avoid abuse of the technology, and enhance the general public’s confidence in the AI technologies. [5]Therefore a comprehensive and a long-term orientated budgeting strategy will be crucial for the future development and market positioning in this sphere.
Conclusion:
To sum up, it can be concluded that in order to apply the generative AI solutions successfully, the expand and the contracts are to achieve the understanding of all the pros and cons, as well as all the costs implied. Similarly, from discussions and research conducted in previous and current analyses, the possible dangers that include ethical issues, privacy and technology constraints are some of the barriers that organizations must contend for these tools to be fully exploited. Not only do the advancing capabilities of generative AI extend the prospect of improving the design and manufacture of products, As shown above in the case of materials science and engineering, but they also require heavy capital investments and recurrent recurring costs for training and other supporting structures. Finally, the prospective organizations, planning to integrate this concept of generative AI to their processes, must weigh these staggering monetary concerns with the opportunities generated by the existence of such innovations and adjust their key decisions corresponding to the identified problematics of deployment of generative AI. All of this will be of vital importance for establishing more sustainable growth in the conditions of the growing competitive pressure.
References
- N. Rane, “Role of ChatGPT and Similar Generative Artificial Intelligence (AI) in Construction Industry,” Oct. 10, 2023, Rochester, NY: 4598258. doi: 10.2139/ssrn.4598258.
- C.-Y. Lin, M. Rahaman, M. Moslehpour, S. Chattopadhyay, and V. Arya, “Web Semantic-Based MOOP Algorithm for Facilitating Allocation Problems in the Supply Chain Domain,” Int J Semant Web Inf Syst, vol. 19, no. 1, pp. 1–23, Sep. 2023, doi: 10.4018/IJSWIS.330250.
- J. A. Jindal, M. P. Lungren, and N. H. Shah, “Ensuring useful adoption of generative artificial intelligence in healthcare,” J. Am. Med. Inform. Assoc., vol. 31, no. 6, pp. 1441–1444, Jun. 2024, doi: 10.1093/jamia/ocae043.
- B. D. Alfia, A. Asroni, S. Riyadi, and M. Rahaman, “Development of Desktop-Based Employee Payroll: A Case Study on PT. Bio Pilar Utama,” Emerg. Inf. Sci. Technol., vol. 4, no. 2, Art. no. 2, Dec. 2023, doi: 10.18196/eist.v4i2.20732.
- N. Rane, “Role of ChatGPT and Similar Generative Artificial Intelligence (AI) in Construction Industry,” Oct. 10, 2023, Rochester, NY: 4598258. doi: 10.2139/ssrn.4598258.
- Sedik, A., Maleh, Y., El Banby, G. M., Khalaf, A. A., Abd El-Samie, F. E., Gupta, B. B., … & Abd El-Latif, A. A. (2022). AI-enabled digital forgery analysis and crucial interactions monitoring in smart communities. Technological Forecasting and Social Change, 177, 121555.
- Mourelle, L. M. (2022). Robotics and AI for Cybersecurity and Critical Infrastructure in Smart Cities. N. Nedjah, A. A. Abd El-Latif, & B. B. Gupta (Eds.). Springer.
- Keesari, T., Goyal, M. K., Gupta, B., Kumar, N., Roy, A., Sinha, U. K., … & Goyal, R. K. (2021). Big data and environmental sustainability based integrated framework for isotope hydrology applications in India. Environmental Technology & Innovation, 24, 101889.
Cite As
Spoorthi K.S. (2024) The Cost of Implementing Generative AI Solutions, Insights2Techinfo, pp.1