By: Aiyaan Hasan, International Center for AI and Cyber Security Research and Innovations (CCRI), Asia University, Taiwan, rayhasan114@gmail.com
Abstract:
Cyber threats have evolved at an exponential rate, which is challenging to organizations as they have to be a step ahead of these “malicious actors”. In this article we shall explore the power of Artificial Intelligence (AI) to reshape the realm of cyber safety and security. We delve into how predictive analysis is helping to revolutionize cyber-security. Predictive analysis has gained a lot of attention over the years in the research community and in addition to this is crucial in the current digital landscape. This article particularly provides insights into the promising future of Cyber threat intelligence.
Introduction:
The cybersecurity realm is expanding exponentially, meaning that the cyberthreats have become more complex and sophisticated. With the advancements in AI and its ability to analyze huge datasets, identifying the patterns and making predictions has emerged as a saviour in the word of cybersecurity. AI-driven predictive analysis has become popular and are taking the Center-stage, which allows the organizations to not only detect the threats but also anticipate what harms they can cause.
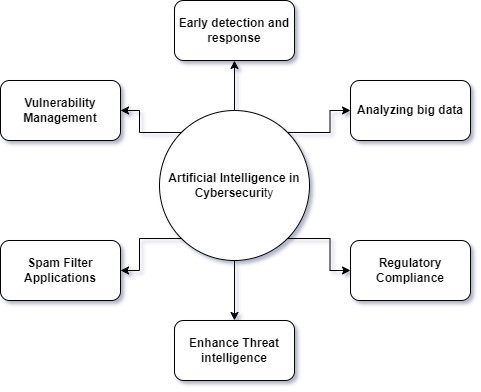
Cyber-threats cannot be eliminated completely and therefore each and every research and development are primarily focused on prevention and lowering the impact of these security related incidents.[1] With respect to the context of cybersecurity, the enormous number of data which can be collected over different time intervals has potential to help and facilitate the understanding the motivation behind these acts with the help of sentiment analysis.[2] Although the advancements in information security has improved ,cyber-attacks are still a critical issue to overcome and tackle.
The role of AI in Cyber Threat Intelligence:
Advancements in AI has played a key role in revolutionizing the way cybersecurity threats are detected, analysed and the way the response needs to be carried out. Ai brings many capabilities into the picture and hence allowing for effective cybersecurity practices:
- Confidentiality: It is a security policy which is often referred to as the protector of information and systems from parties which are unauthorized. The threats of confidentiality mainly target the databases, the serves of applications and the administrators.[3]
- Anomaly Detection: The AI based anomaly detection algorithms are capable of recognizing irregular patterns in the data, for example: user behaviour of unusual network related traffic.
- Threat Prediction: AI is capable of predicating the cybersecurity threats-based data aggregated from ongoing events and historical data this allows them to develop threat prediction algorithms and models which can be used to strengthen the defences.[4]
- Automated threat response: Not only is the model capable of identifying the anomalies and predicting the threats, but also react accordingly for the prevention cyberthreats
- Enhanced Threat Intelligence: AI is capable of handling and analysing vast amounts of data from multiple resources which shall also include identifying potential threats which are specifically targeting the organization.[5]
- Adaptive Defences: AI is capable of adapting to the changing landscapes of threats. As the attackers consistently find out new ways to exploit, AI systems are evolving too and are continuously learning from the new data and are adjusting to new security measure which are much more enhanced.
Advanced Machine learning algorithms:
These are the algorithms which are sophisticated in nature and are used in the field of artificial intelligence (AI) which enables the computers so as to learn from the data and make it convenient for predictions as well as decision making. An overview of some advanced learning algorithms which are commonly used in the field of cybersecurity are as follows:
a) Supervised learning:
- Support vector machines (SVM): SVMs are particularly used for the classification of binaries, such as to differentiate between the legitimate network traffic and the malicious ones. These are highly capable of identifying patterns in data.
- Random Forest: It is basically an ensemble learning method which is used to combine multiple decision trees which leads to the improved classification accuracy. It is primarily used for the detection of any kind of malware and attempts of intrusion.
b) Unsupervised learning:
- K-means Clustering: It is basically used for clustering, basically grouping data points into clusters depending on the level of similarity. With respect to cybersecurity, it is helpful in identifying the “anomalies”.
- Auto-encoders: These are neural networks which are used for feature learning. These are valuable when it comes to recognition of unusual patterns in the network traffic or even in the system behaviour.
c) Deep learning:
- Convolutional Neural network (CNNs): These are commonly used for threat- detection which is image based, like identifying malware in files or the malicious content that might be present in the images.
- Long Short-Term Memory (LSTM) Networks: These are similar to RNN which is designed specifically for sequential data. These are specialized in identifying subtle patterns in time-series data, which makes them a suitable option specifically for the purpose of intrusion detection.
d) Ensemble learning:
- AdaBoost: This is a technique that particularly focuses on the weaknesses of each individual model and is commonly used for the purpose of malware detection.
- Gradient Boosting Machines (GBM): This ensemble technique combines predictions from multiple models. It enhances the accuracy in various tasks in cybersecurity, for example: detecting phishing emails.
e) Semi-Supervised learning:
- Label Propagation: These techniques can be used to propagate label from a small labelled dataset to a larger unlabelled dataset, used in threat detection.
Challenges and ethical considerations:
Performing cybersecurity research requires a reference data, which is based on real systems and users, in order to test the validity as well as its efficacy for the predictions of a particular given theory.[6] Ethical and practical concerns arise when the operational data needs to be shared. Here’s an overview of some key challenges and ethical considerations:
- Regulatory Compliance: Navigating the complex scene of regulations and the standards, such as GDPR, while implementing Artificial intelligence in cybersecurity which can be challenging.
- Resource requirements: Training as well as maintaining the AI models, especially when it comes to deep neural networks can be very expensive computationally and intensive, which can act as a major impediment for new and small businesses and organizations.
- Data quality and quantity: AI algorithms primarily rely on high-quality datasets for the purpose of training. When it comes to cybersecurity, obtaining a clean and labelled data for both the malicious and the normal activities can be a challenging task.
- Transparency and Accountability: The organizations are supposed to remain transparent with respect to the usage of AI in cybersecurity and therefore be accountable for every decision made by the AI systems.
- Privacy: Protection of the privacy of its users be it an individual or an organization is of paramount importance. AI systems should ensure the sensitive data which is being handle by it is for legit security purposes only.
As our world continues getting interconnected, threats to our cybersecurity can come from unlikely and unexpected sources and directions. In recent years, malicious activities have sky-rocketed and are becoming increasingly complex and serious.
Calendar year | Number of reported incidents of malicious cyber activity | Percent increase from previous year |
2000 | 1415 | N/A |
2001 | 3651 | 158.02% |
2002 | 4352 | 19.2% |
2003 | 9919 | 127.92% |
2004 | 16110 | 62.42% |
2005 | 23031 | 42.96% |
2006 | 30215 | 31.19% |
2007 | 43880 | 45.23% |
2008 | 54640 | 24.52% |
2009 | 71661 | 31.15% |
Table 1. Represents the reported incidents of malicious cyber activities targeting the US
Department of Defense’s system from 2000 to 2009. [6]
The Promising Future of Cyber Threat Intelligence:
In recent years many infrastructures and power systems have been subjected to countless sophisticated cyberthreats, which includes targeted attacks. A response to this challenging situation is building enhanced threat intelligence which is interlinking information sharing and situation awareness.[7]
Cyberthreats have become increasingly sophisticated over the years. However, there’s a promising future in the fields of cybersecurity- A future where the advanced technologies like artificial intelligence, machine learning and data analytics can be used to transform the way organizations look and approach the cyber threat intelligence.
Conclusion:
As organizations are embracing predictive analysis, AI-driven capabilities, behavioural analytics and much more, they gain a significant advantage over the battle against the cyber threats. In order to understand the capabilities and its full potential, organizations are supposed to be investing in AI based solutions, developing cybersecurity strategies and prioritizing AI deployment. The future of cyber threat intelligence is bright, which offers enhanced protection in the evolving field of cyber threats.
References
- Husák, M., Bartoš, V., Sokol, P., & Gajdoš, A. (2021, February 1). Predictive methods in cyber defense: Current experience and research challenges. Future Generation Computer Systems; Elsevier BV.
- Hernandez-Suarez, A., Toscano-Medina, K., Martínez-Hernández, V., Olivares-Mercado, J., & Sanchez, V. (2018, April 29). Social Sentiment Sensor in Twitter for Predicting Cyber- Attacks Using ℓ1 Regularization. Sensors; Multidisciplinary Digital Publishing Institute.
- Sarker, I. H., Furhad, H., & Nowrozy, R. (2021, March 26). AI-Driven Cybersecurity: An Overview, Security Intelligence Modeling and Research Directions. SN Computer Science; Springer Science+Business Media.
- Kandias, M., Mylonas, A., Virvilis, N., Theoharidou, M., & Gritzalis, D. (2010, January 1). An Insider Threat Prediction Model. Lecture Notes in Computer Science.
- Leszczyna, R., & Wróbel, M. R. (2019, May 29). Threat intelligence platform for the energy sector. Software: Practice and Experience, 49(8), 1225–1254.
- Announcement (ScienceDirect Article in Press). (2004, January). Assessing Writing, 9(1), III–IV.
- Shou, D. (2012). Ethical Considerations of Sharing Data for Cybersecurity Research. Financial Cryptography and Data Security, 169–177.
Cite As:
Hasan A. (2023) The Future of Cyber Threat Intelligence: AI-Driven Predictive Analysis, Insights2Techinfo, pp.1